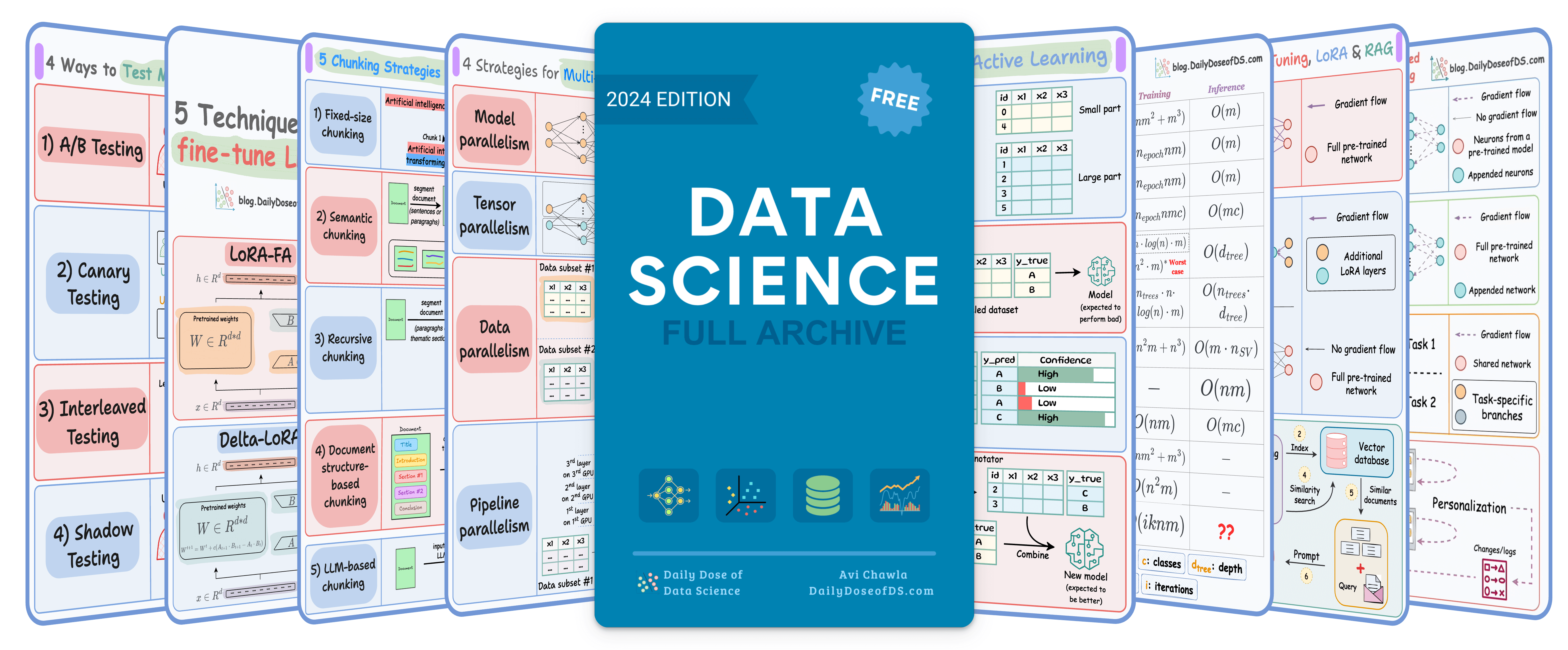
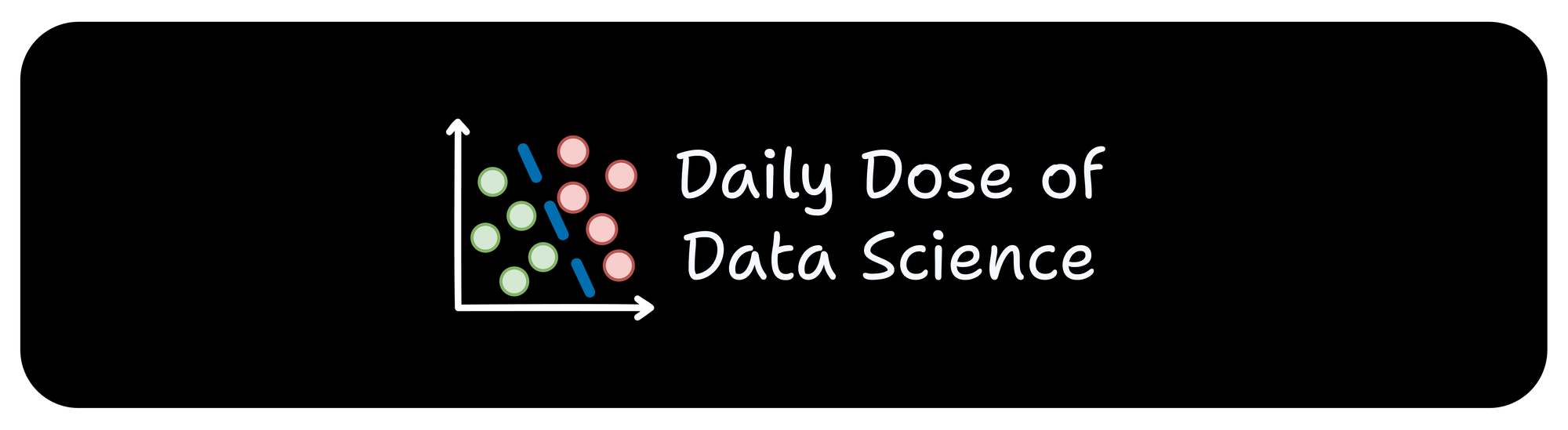
TODAY'S ISSUE
TODAY’S DAILY DOSE OF DATA SCIENCE
[Hands-on] Corrective RAG Agentic Workflow
Corrective RAG is a common technique to improve RAG systems. It introduces a self-assessment step of the retrieved documents, which helps in retaining the relevance of generated responses.
Here’s an overview of how it works:
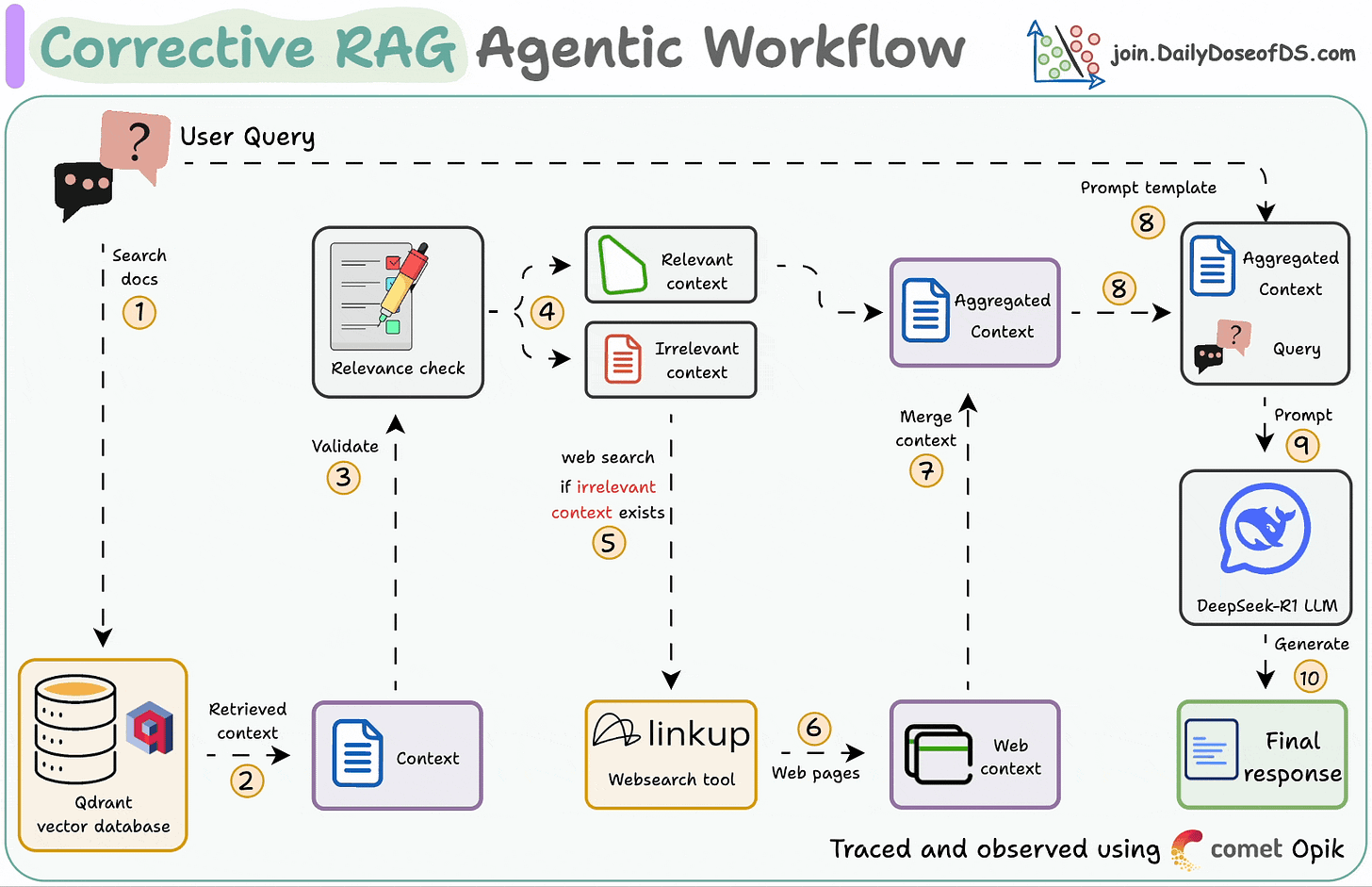
- First search the docs with user query.
- Evaluate if the retrieved context is relevant using LLM.
- Only keep the relevant context.
- Do web search if needed.
- Aggregate the context & generate response.
The video at the top shows how it works!
Let’s implement this today!
Here’s our tech stack for this demo:
- LlamaIndex workflows for orchestration
- Linkup for deep web search
- Cometml’s Opik to trace and monitor
- Qdrant to self-host vectorDB.
The code is available in this Studio: Corrective RAG with DeepSeek-R1. You can run it without any installations by reproducing our environment below:
Implementation
Corrective RAG
Let’s implement Corrective RAG now!
Setup LLM
We will use DeepSeek-R1 as LLM, locally served using Ollama.
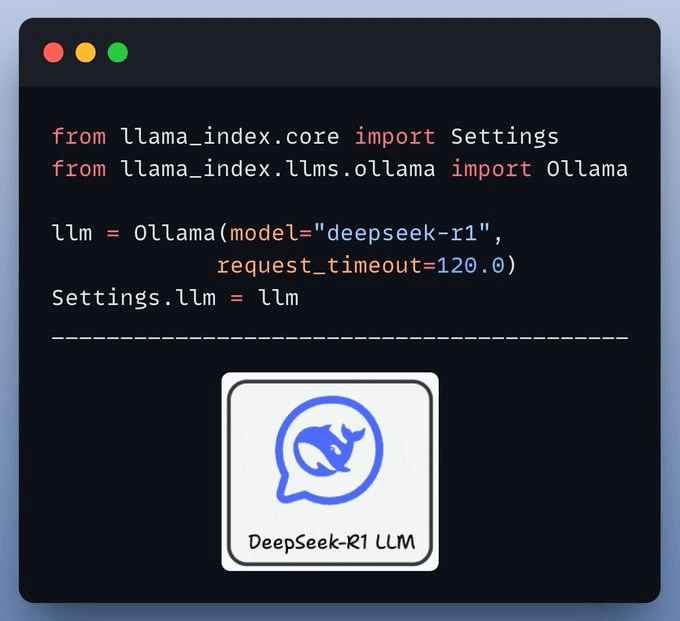
Setup vectorDB
Our primary source of knowledge is the user documents that we index and store in a Qdrant vectorDB collection.
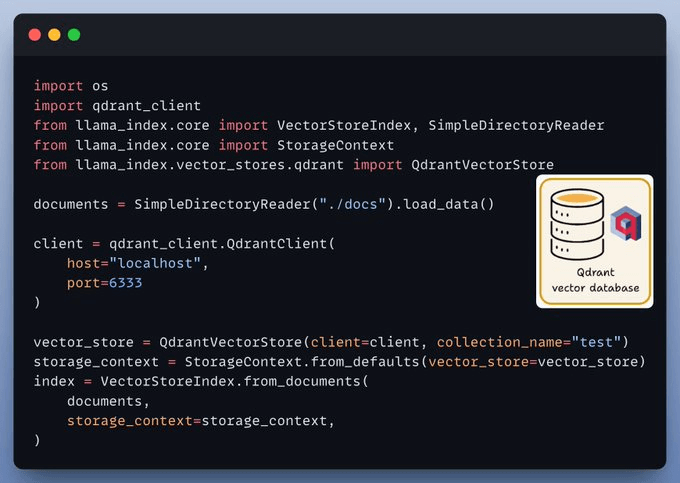
Set up web search tool
To equip our system with web search capabilities, we will use Linkup's state-of-the-art deep web search features. It also offers seamless integration with LlamaIndex.
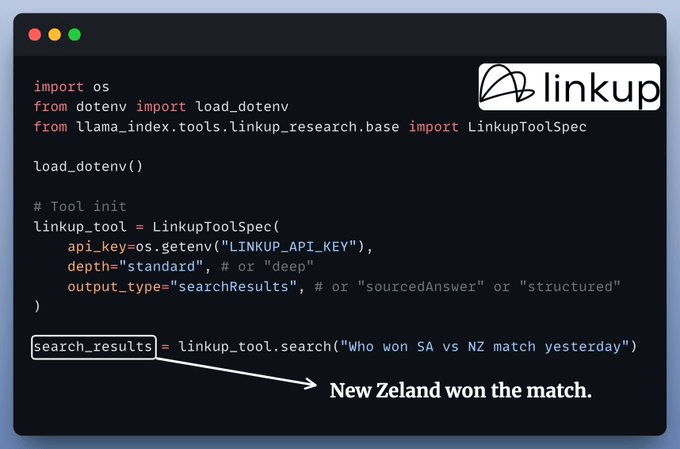
Tracing and Observability
LlamaIndex also offers a seamless integration with CometML’s Opik. You can use this to trace every LLM call, monitor, and evaluate your LLM application.
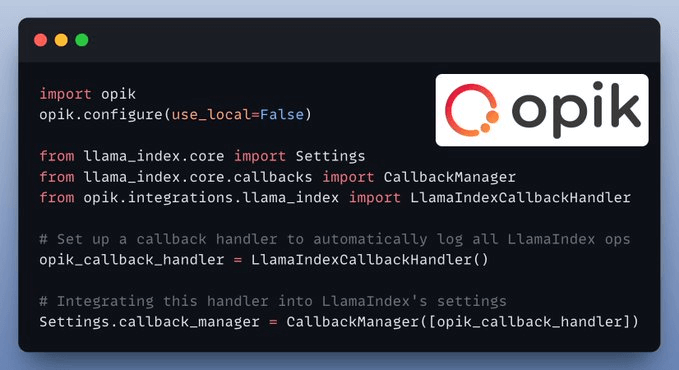
Create the workflow
Now that we have everything set up, it's time to create the event-driven agentic workflow that orchestrates our application.
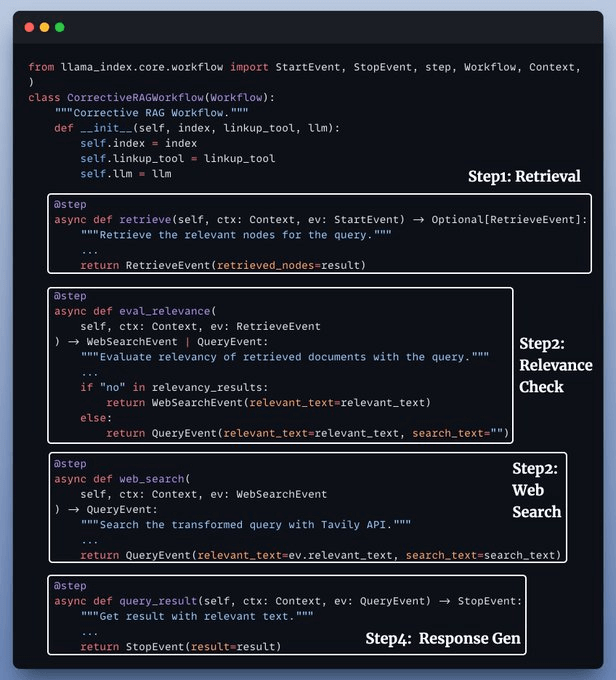
We pass in the LLM, vector index, and web search tool to initialise the workflow.
Visualize the workflow
You can also plot and visualize the workflow, which is helpful for documentation and understanding how the app works.
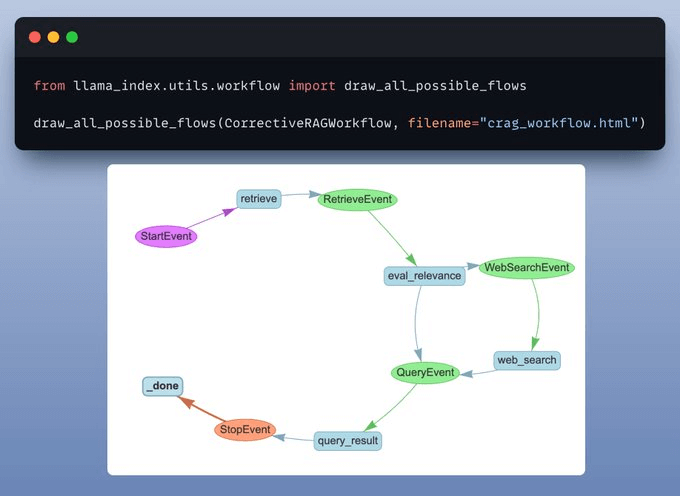
Kickoff the workflow
Finally, when we have everything ready, we kick off our workflow.
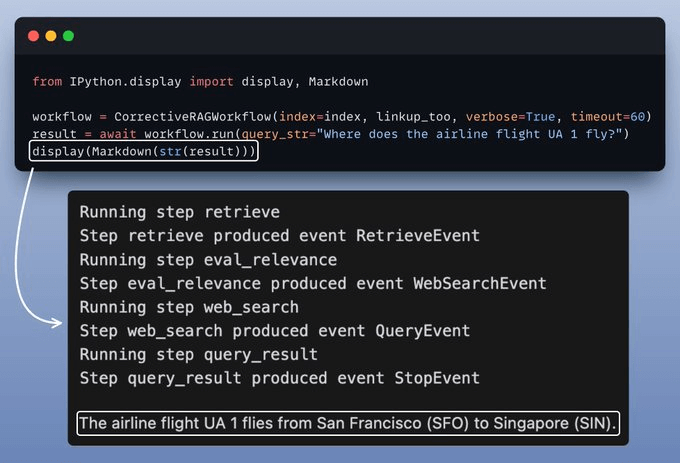
Here’s something interesting. While the vector database holds information about some research papers, it is still able to answer completely unrelated questions—thanks to the web search (which provides additional context when needed):
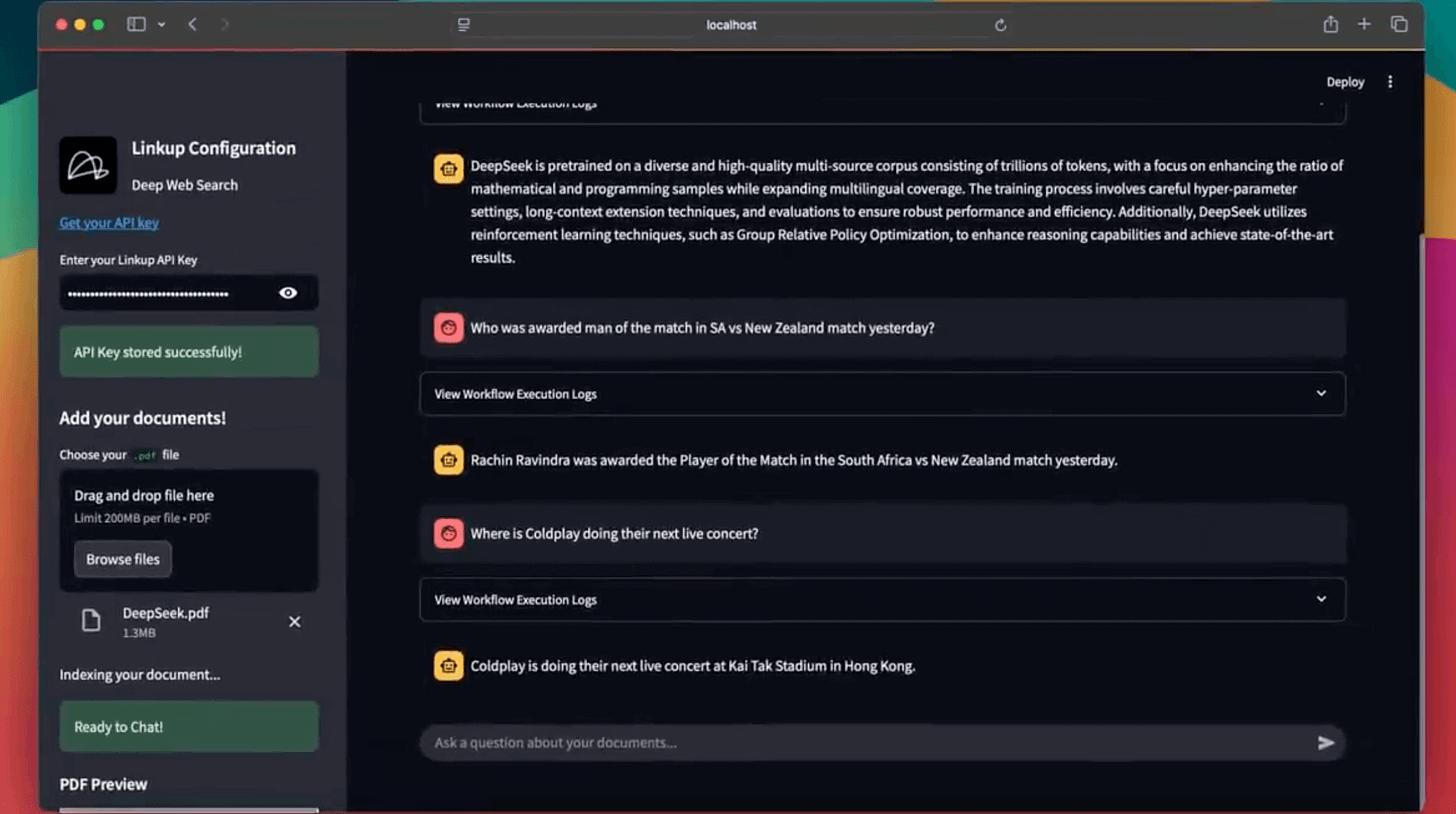
The code is available in this Studio: Corrective RAG with DeepSeek-R1. You can run it without any installations by reproducing our environment below:
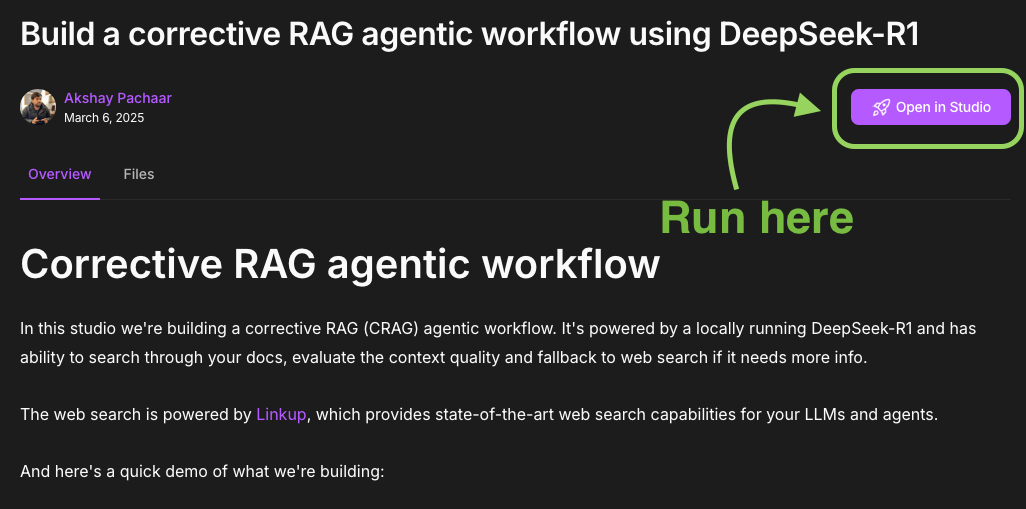
If you want to dive into building LLM apps, our full RAG crash course discusses RAG from basics to beyond:
- RAG fundamentals
- RAG evaluation
- RAG optimization
- Multimodal RAG
- Graph RAG
- Multivector retrieval using ColBERT
- RAG over complex real world docs ft. ColPali
👉 Over to you: What other RAG demos would you like to see?
Thanks for reading!
THAT'S A WRAP
No-Fluff Industry ML resources to
Succeed in DS/ML roles
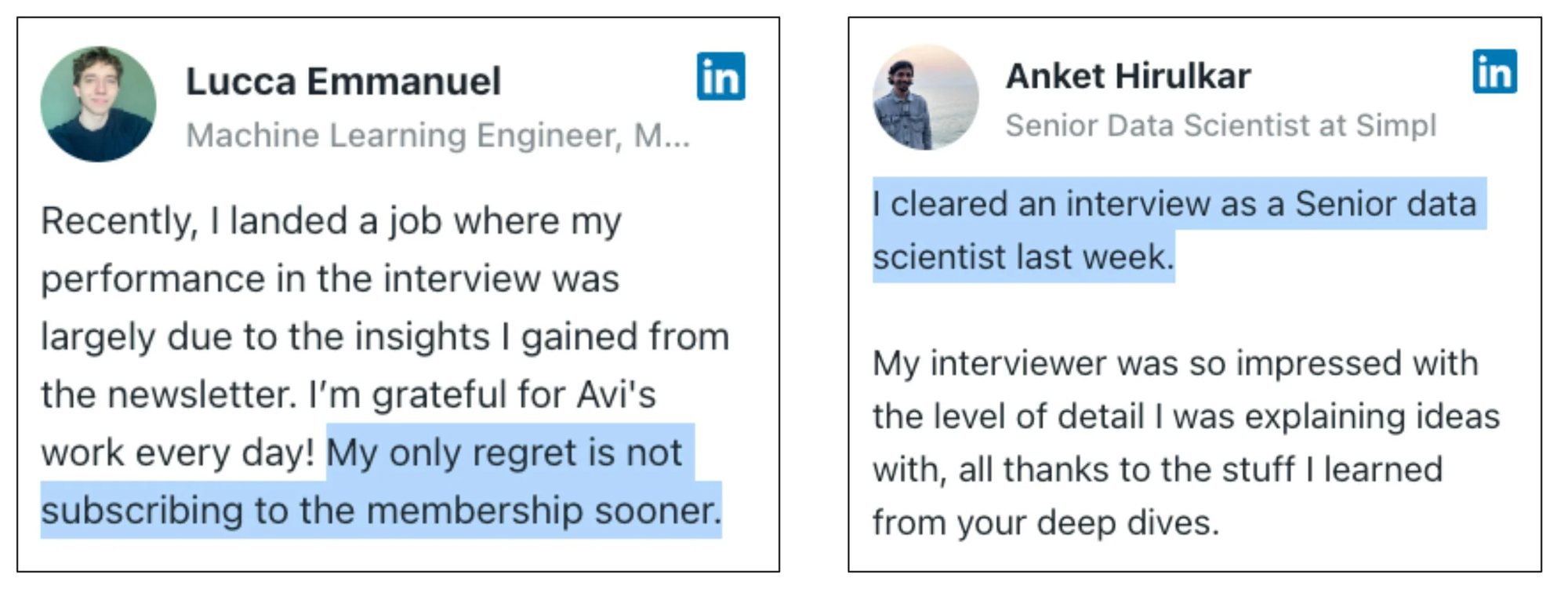
At the end of the day, all businesses care about impact. That’s it!
- Can you reduce costs?
- Drive revenue?
- Can you scale ML models?
- Predict trends before they happen?
We have discussed several other topics (with implementations) in the past that align with such topics.
Here are some of them:
- Learn sophisticated graph architectures and how to train them on graph data in this crash course.
- So many real-world NLP systems rely on pairwise context scoring. Learn scalable approaches here.
- Run large models on small devices using Quantization techniques.
- Learn how to generate prediction intervals or sets with strong statistical guarantees for increasing trust using Conformal Predictions.
- Learn how to identify causal relationships and answer business questions using causal inference in this crash course.
- Learn how to scale and implement ML model training in this practical guide.
- Learn 5 techniques with implementation to reliably test ML models in production.
- Learn how to build and implement privacy-first ML systems using Federated Learning.
- Learn 6 techniques with implementation to compress ML models.
All these resources will help you cultivate key skills that businesses and companies care about the most.
SPONSOR US
Advertise to 600k+ data professionals
Our newsletter puts your products and services directly in front of an audience that matters — thousands of leaders, senior data scientists, machine learning engineers, data analysts, etc., around the world.