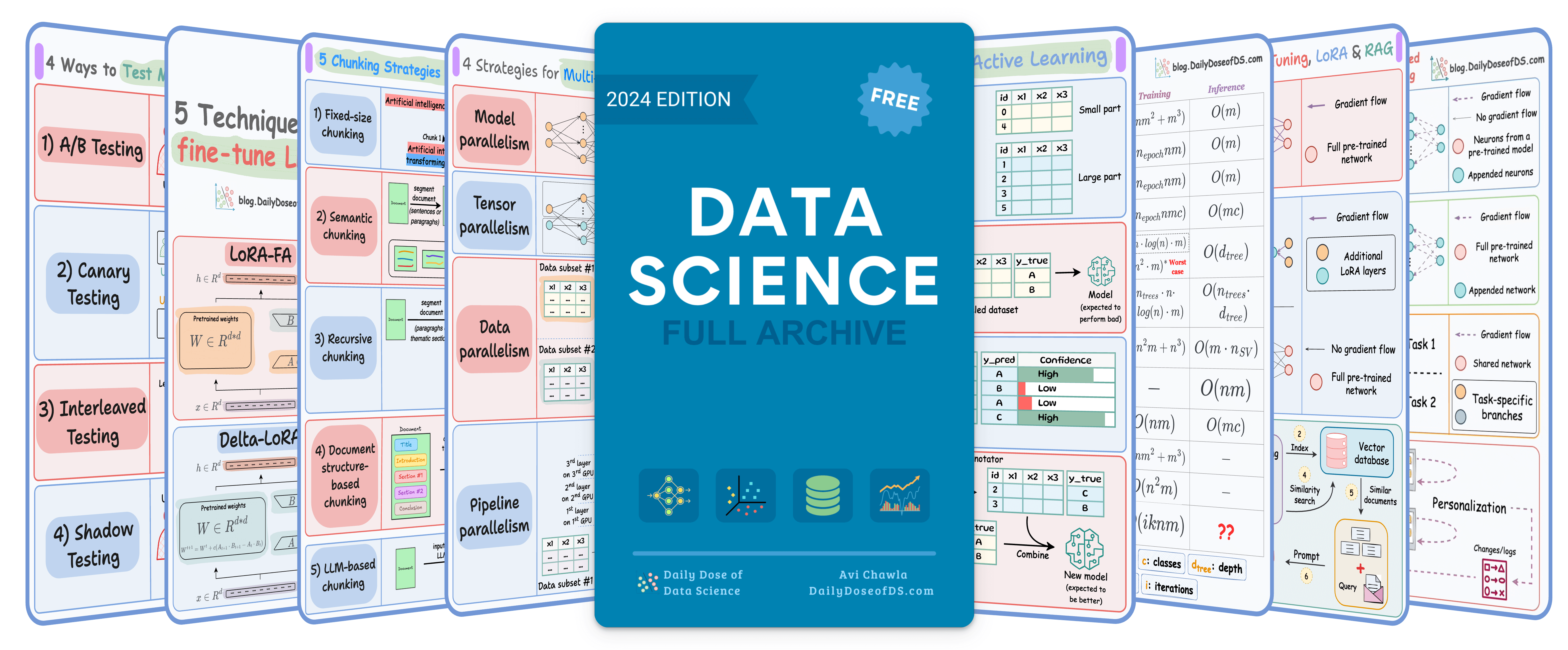
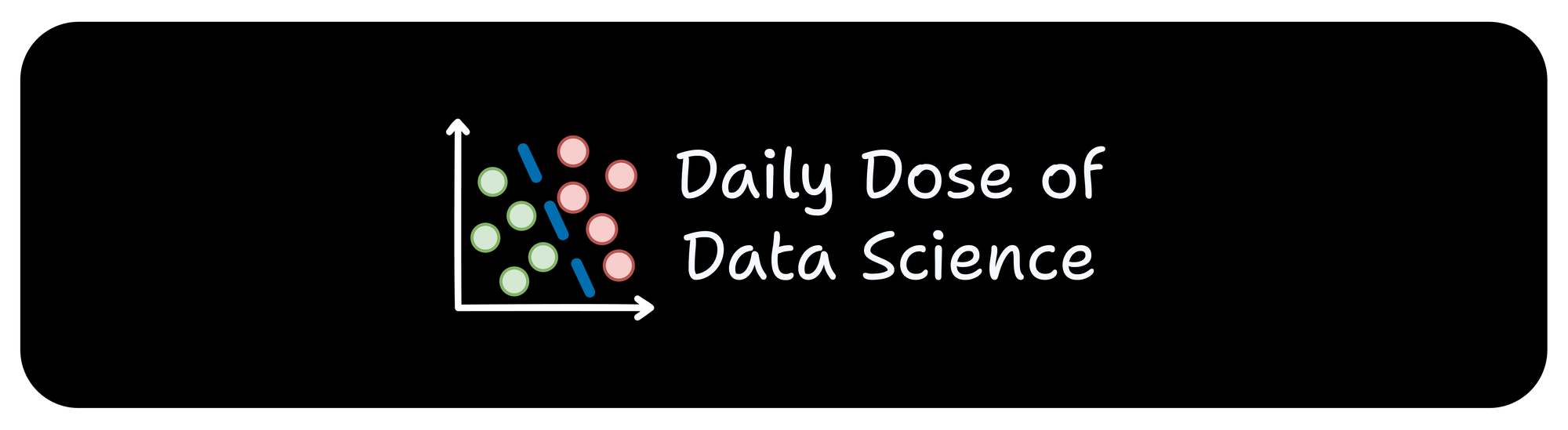
TODAY'S ISSUE
TODAY’S DAILY DOSE OF DATA SCIENCE
MCP-powered Agentic RAG
Today, we are showcasing another demo with MCP—an Agentic RAG.
In the video below, we have an MCP-driven Agentic RAG that searches a vector database and falls back to web search if needed.
To build this, we'll use:
- Bright Data to scrape web at scale.
- Qdrant as the vector DB.
- Cursor as the MCP client.
Here's the workflow:
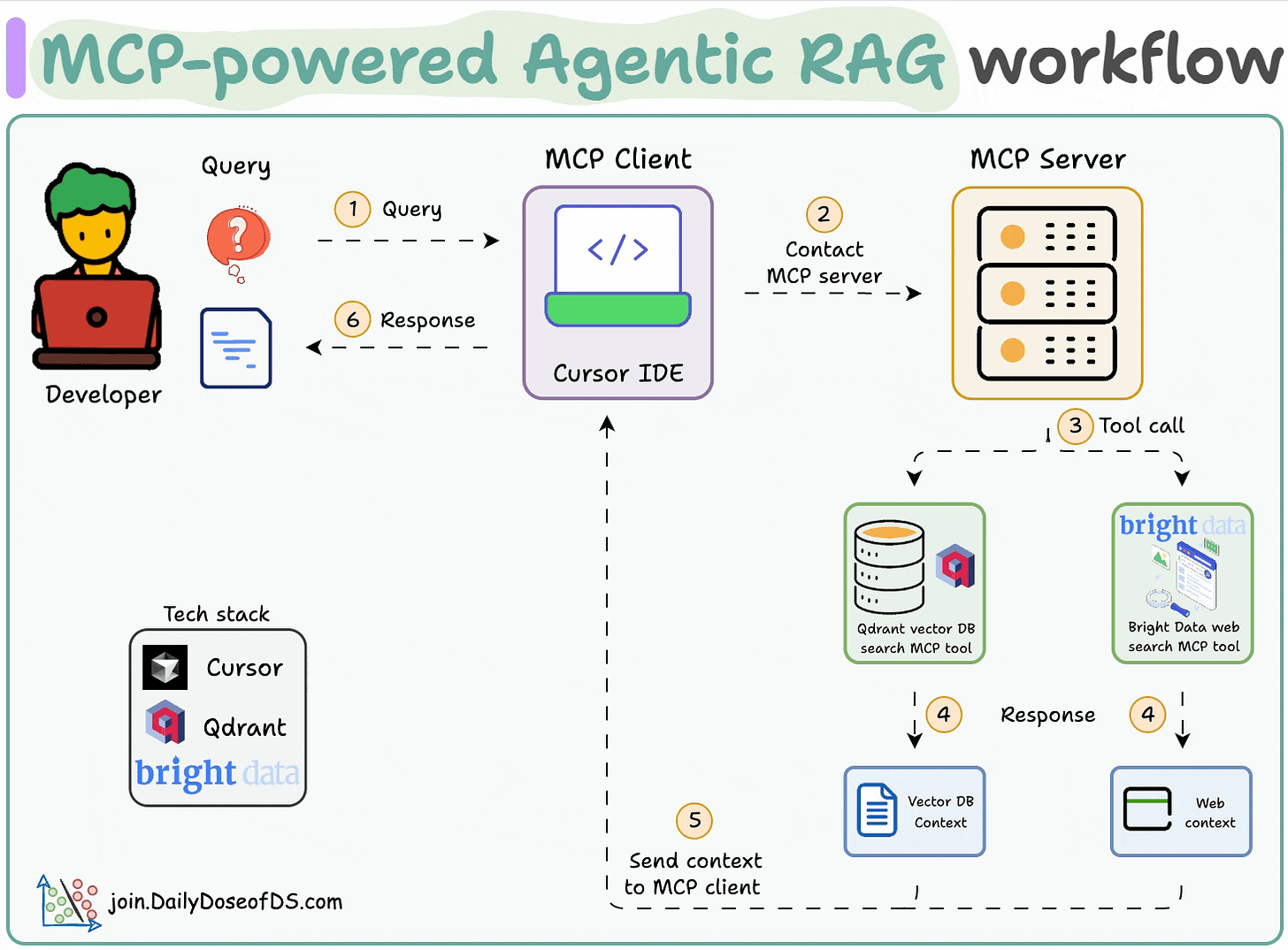
- 1) The user inputs a query through the MCP client (Cursor).
- 2-3) The client contacts the MCP server to select a relevant tool.
- 4-6) The tool output is returned to the client to generate a response.
The code is linked later in the issue.
Let's implement this!
#1) Launch an MCP server
First, we define an MCP server with the host URL and port.
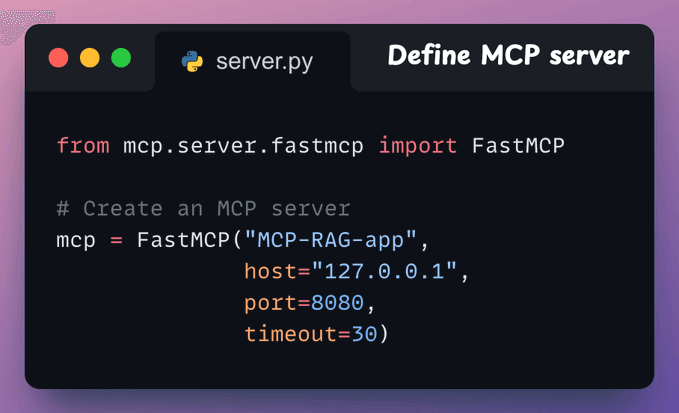
#2) Vector DB MCP tool
A tool exposed through an MCP server has two requirements:
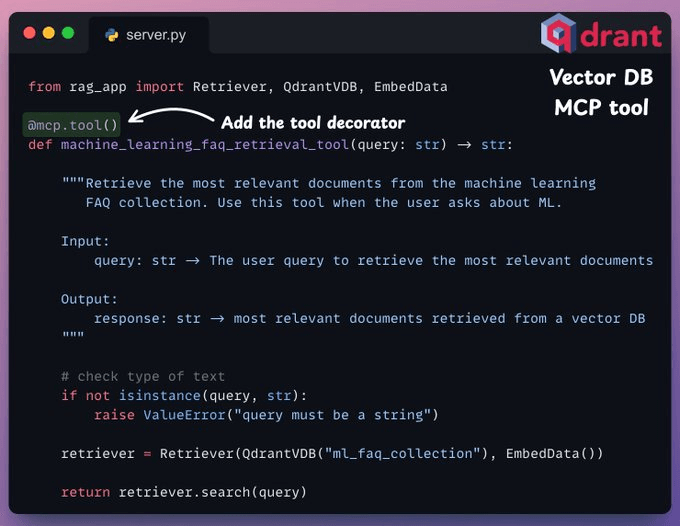
- It must be decorated with the "tool" decorator.
- It must have a clear docstring.
Below, we have an MCP tool to query a vector DB. It stores ML-related FAQs.
#3) Web search MCP tool
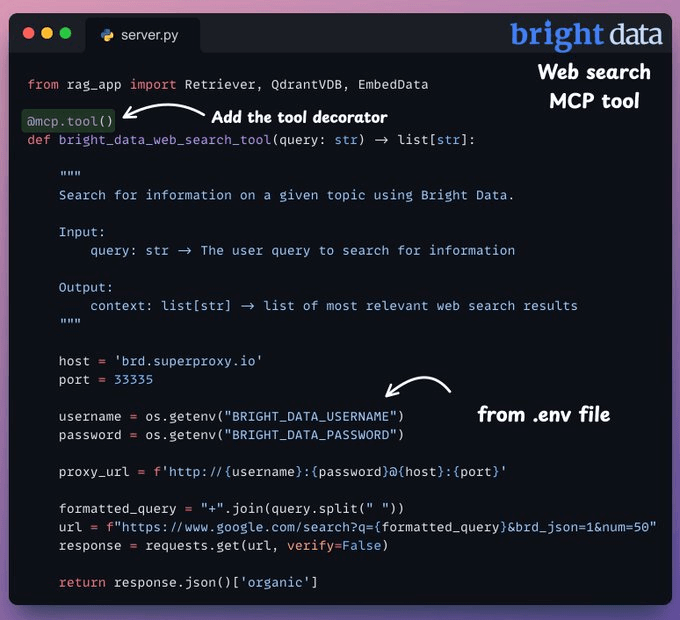
If the query is unrelated to ML, we need a fallback mechanism.
Thus, we resort to web search using Bright Data's SERP API to scrape data at scale across several sources to get relevant context.
#4) Integrate MCP server with Cursor
In our setup, Cursor is an MCP host/client that uses the tools exposed by the MCP server.
To integrate the MCP server, go to Settings → MCP → Add new global MCP server.
In the JSON file, add what's shown below👇
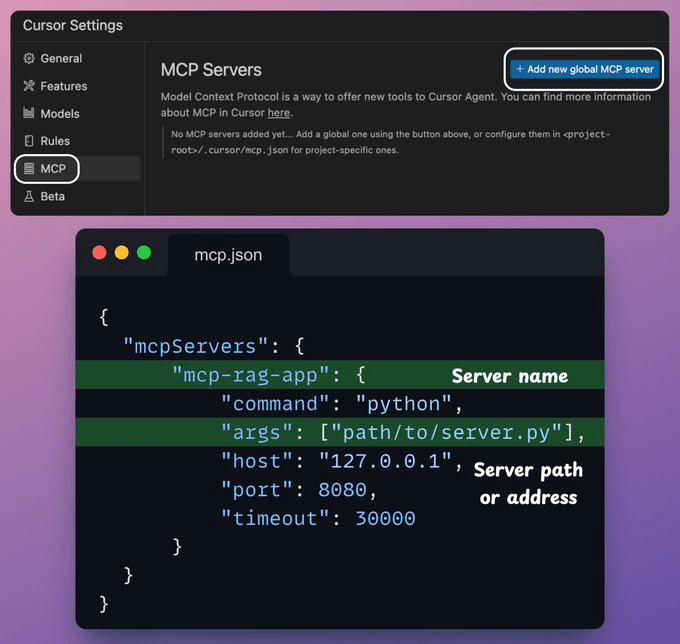
Done! Your local MCP server is live and connected to Cursor 🚀!
It has two MCP tools:
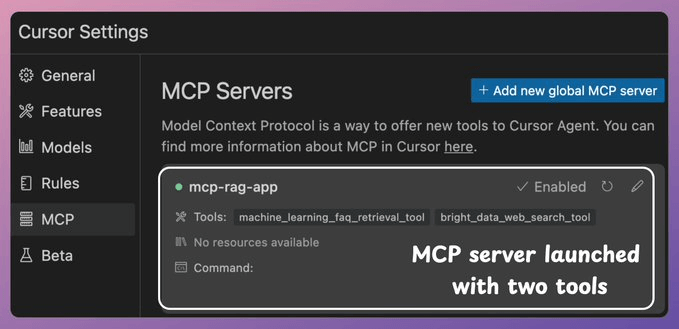
- Bright Data web search tool to scrape data at scale.
- Vector DB search tool to query the relevant documents.
Next, we interact with the MCP server.
- When we ask an ML-related query, it invokes the vector DB tool.
- But when we ask a general query, it invokes the Bright Data web search tool to gather web data at scale from various sources.
When Agents use tools, they run into issues like IP blocks, bot traffic, captcha solvers, etc. This hinders the Agent's execution.
To solve this, we used Bright Data in this demo.
It lets you:
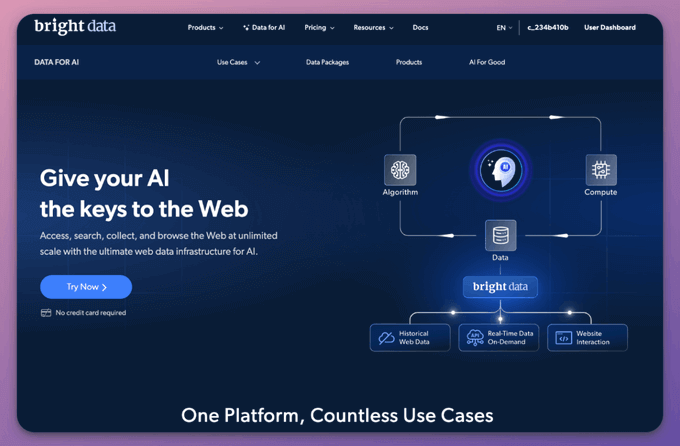
- Scrape data for Agents at scale without getting blocked.
- Simulate user behavior using advanced browser tools.
- Build Agentic apps with real-time and historical web data.
Find the code in this GitHub repo: MCP implementation repo.
Thanks for reading!
IN CASE YOU MISSED IT
​16 techniques to build real-world RAG systems​
On paper, implementing a RAG system seems simple—connect a vector database, process documents, embed the data, embed the query, query the vector database, and prompt the LLM.
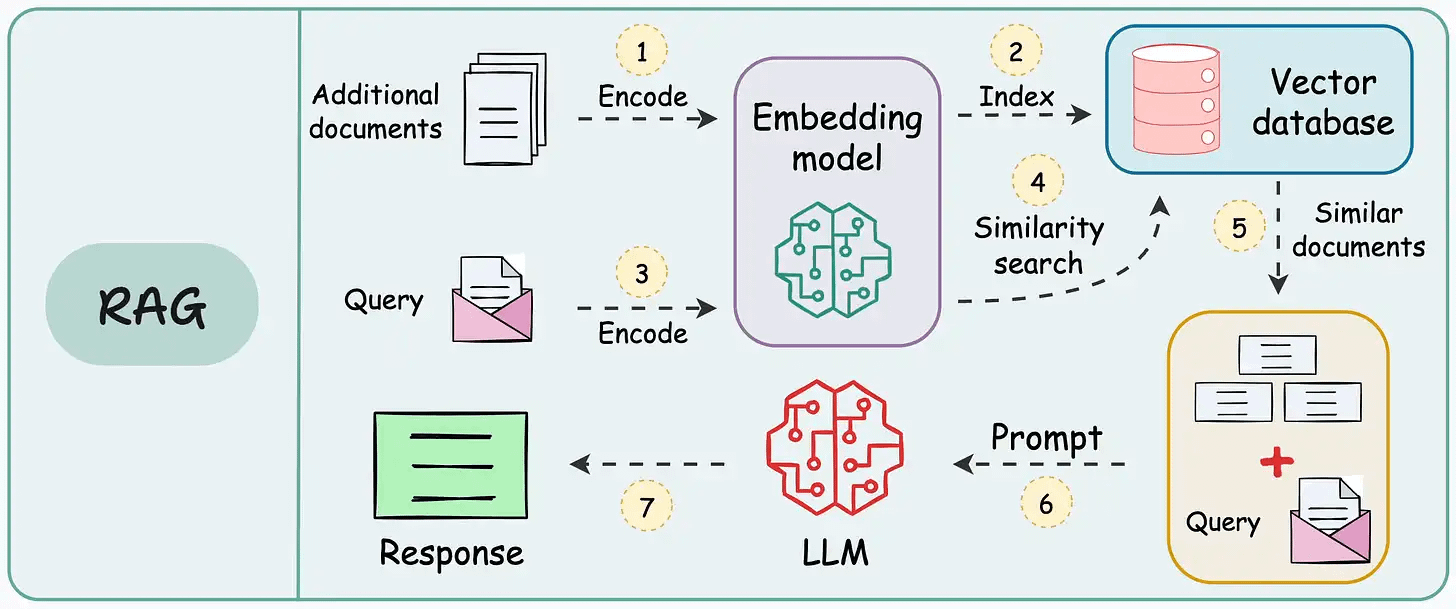
But in practice, turning a prototype into a high-performance application is an entirely different challenge.
We published a two-part guide that covers 16 practical techniques to build real-world RAG systems:
ROADMAP
From local ML to production ML
Once a model has been trained, we move to productionizing and deploying it.
If ideas related to production and deployment intimidate you, here’s a quick roadmap for you to upskill (assuming you know how to train a model):
- First, you would have to compress the model and productionize it. Read these guides:
- Reduce their size with ​Model Compression techniques​.
- ​Supercharge ​​PyTorch Models​​ With TorchScript.​
- If you use sklearn, learn how to ​optimize them with tensor operations​
- Next, you move to deployment. ​Here’s a beginner-friendly hands-on guide​ that teaches you how to deploy a model, manage dependencies, set up model registry, etc.
- Although you would have tested the model locally, it is still wise to test it in production. There are risk-free (or low-risk) methods to do that. ​Learn what they are and how to implement them here​.
This roadmap should set you up pretty well, even if you have NEVER deployed a single model before since everything is practical and implementation-driven.
THAT'S A WRAP
No-Fluff Industry ML resources to
Succeed in DS/ML roles
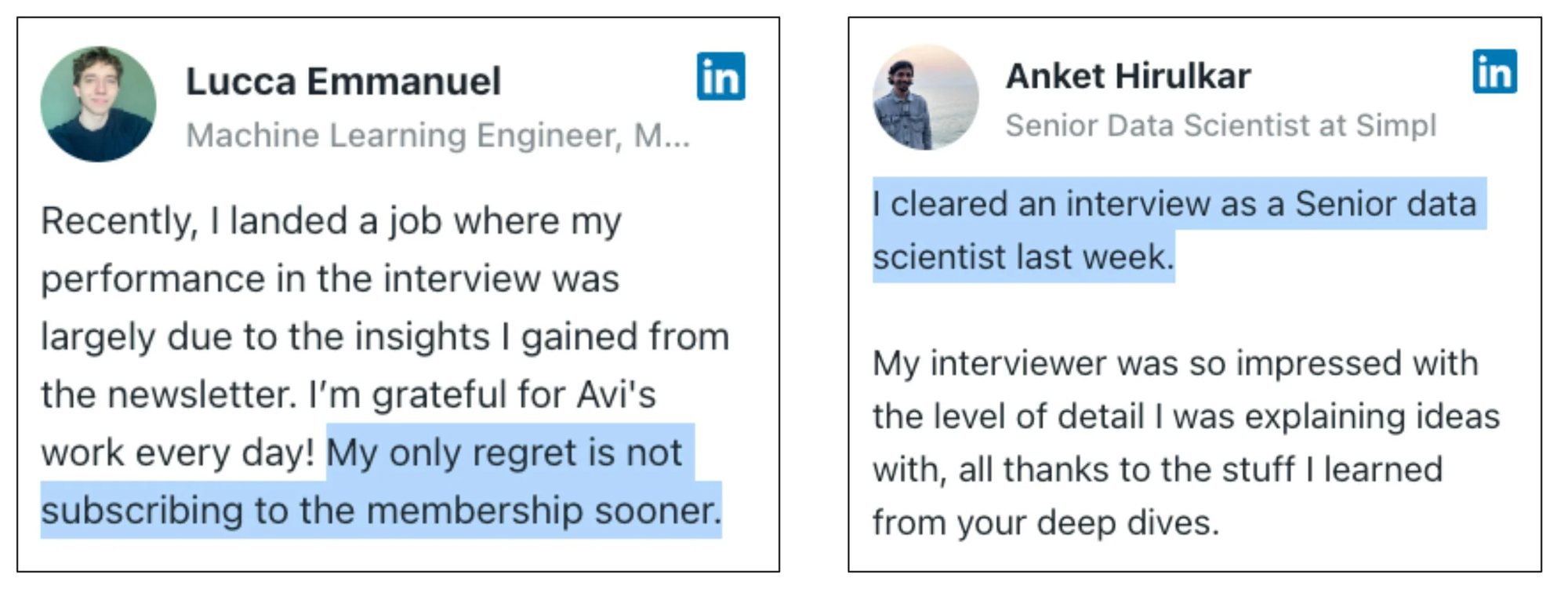
At the end of the day, all businesses care about impact. That’s it!
- Can you reduce costs?
- Drive revenue?
- Can you scale ML models?
- Predict trends before they happen?
We have discussed several other topics (with implementations) in the past that align with such topics.
Here are some of them:
- Learn sophisticated graph architectures and how to train them on graph data in this crash course.
- So many real-world NLP systems rely on pairwise context scoring. Learn scalable approaches here.
- Run large models on small devices using Quantization techniques.
- Learn how to generate prediction intervals or sets with strong statistical guarantees for increasing trust using Conformal Predictions.
- Learn how to identify causal relationships and answer business questions using causal inference in this crash course.
- Learn how to scale and implement ML model training in this practical guide.
- Learn 5 techniques with implementation to reliably test ML models in production.
- Learn how to build and implement privacy-first ML systems using Federated Learning.
- Learn 6 techniques with implementation to compress ML models.
All these resources will help you cultivate key skills that businesses and companies care about the most.
SPONSOR US
Advertise to 600k+ data professionals
Our newsletter puts your products and services directly in front of an audience that matters — thousands of leaders, senior data scientists, machine learning engineers, data analysts, etc., around the world.