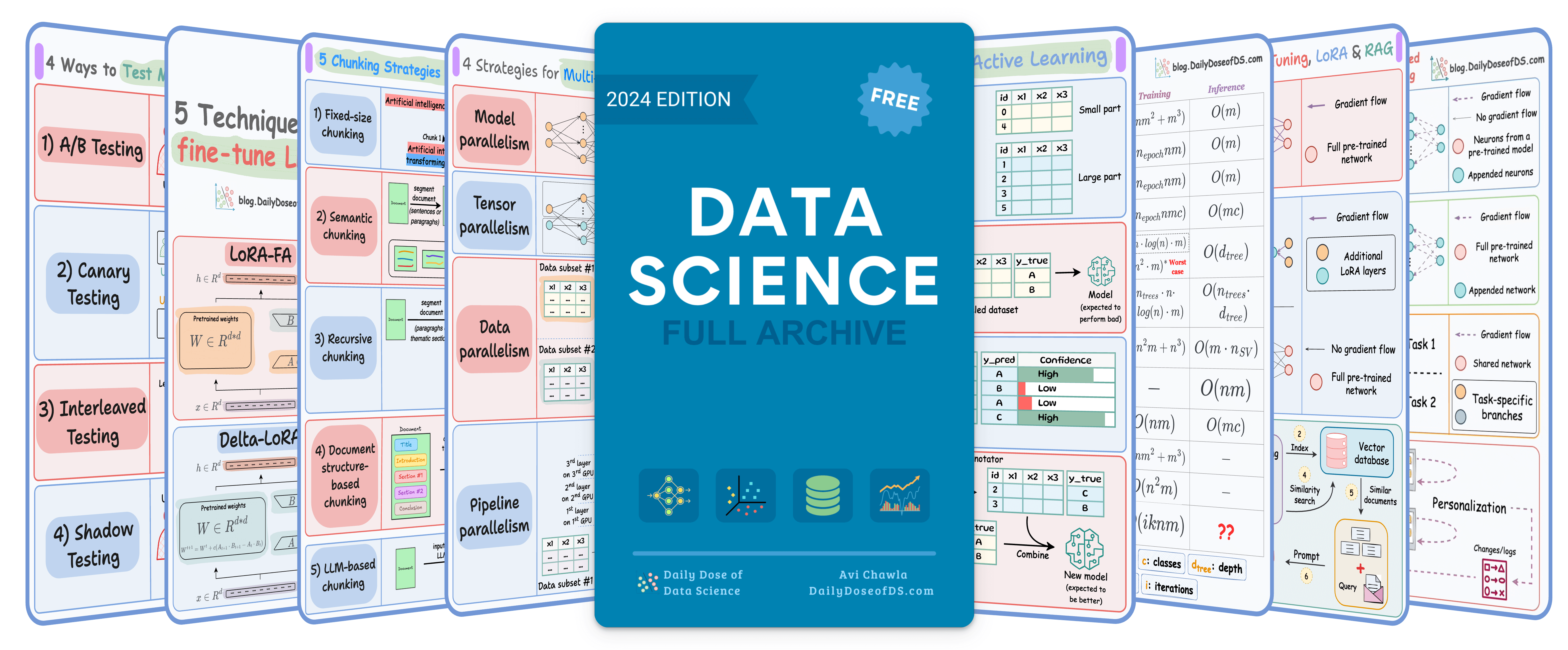
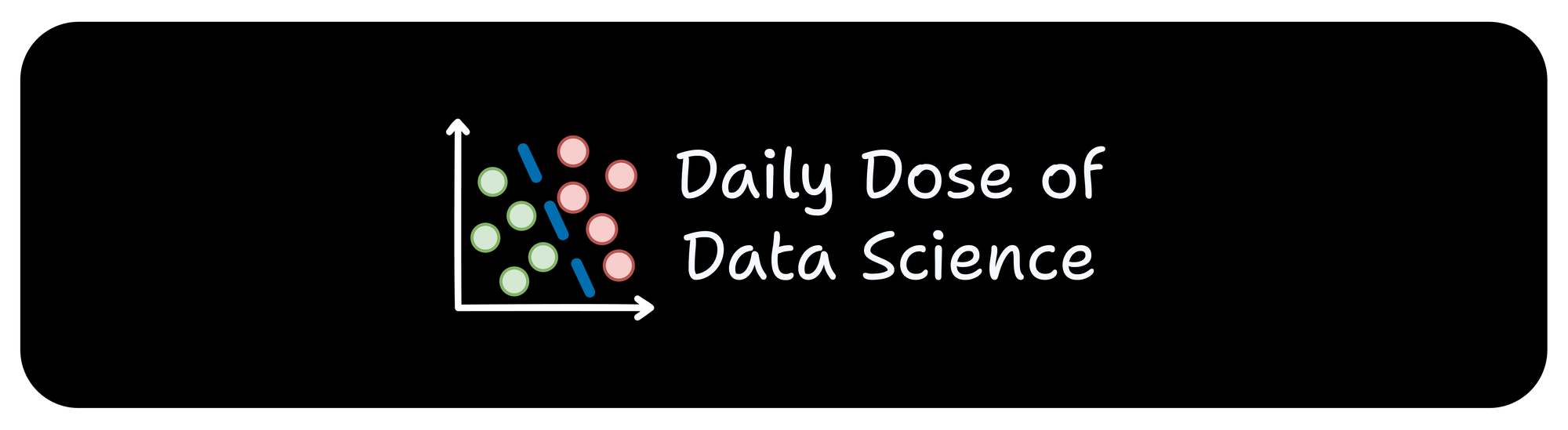
TODAY'S ISSUE
TOGETHER WITH EYELEVEL
Build incredibly robust RAG systems with EyeLevel​
Vanilla RAGs work as long as your external docs. look like the image on the left, but real-world documents are like the image on the right:
They have images, text, tables, flowcharts, and whatnot!
No vanilla RAG system can handle this complexity.
​EyeLevel's GroundX​ is solving this.
They are developing systems that can intuitively chunk relevant content and understand what’s inside each chunk, whether it's text, images, or diagrams as shown below:
As depicted above, the system takes an unstructured (text, tables, images, flow charts) input and parses it into a JSON format that LLMs can easily process to build RAGs over.
Try ​EyeLevel's GroundX​ to build real-world robust RAG systems:
Thanks to ​EyeLevel​ for sponsoring today's issue.
TODAY’S DAILY DOSE OF DATA SCIENCE
Prompting vs. RAG vs. Finetuning?
Continuing the discussion on RAGs from EyeLevel...
If you are building real-world LLM-based apps, it is unlikely you can start using the model right away without adjustments. To maintain high utility, you either need:
- Prompt engineering
- Fine-tuning
- RAG
- Or a hybrid approach (RAG + fine-tuning)
The following visual will help you decide which one is best for you:
Two important parameters guide this decision:
- The amount of external knowledge required for your task.
- The amount of adaptation you need. Adaptation, in this case, means changing the behavior of the model, its vocabulary, writing style, etc.
For instance, an LLM might find it challenging to summarize the transcripts of company meetings because speakers might be using some internal vocabulary in their discussions.
So here's the simple takeaway:
- Use RAGs to generate outputs based on a custom knowledge base if the vocabulary & writing style of the LLM remains the same.
- Use fine-tuning to change the structure (behaviour) of the model than knowledge.
- Prompt engineering is sufficient if you don't have a custom knowledge base and don't want to change the behavior.
- And finally, if your application demands a custom knowledge base and a change in the model's behavior, use a hybrid (RAG + Fine-tuning) approach.
That's it!
If RAG is your solution, check out ​​EyeLevel's GroundX​ for building robust RAG systems on complex real-world documents.
👉 Over to you: How do you decide between prompting, RAG, and fine-tuning?
Thanks for reading!
Training OPTIMIZATION
Multi-GPU TRAINING (A Practical Guide)
If you look at job descriptions for Applied ML or ML engineer roles on LinkedIn, most of them demand skills like the ability to train models on large datasets:
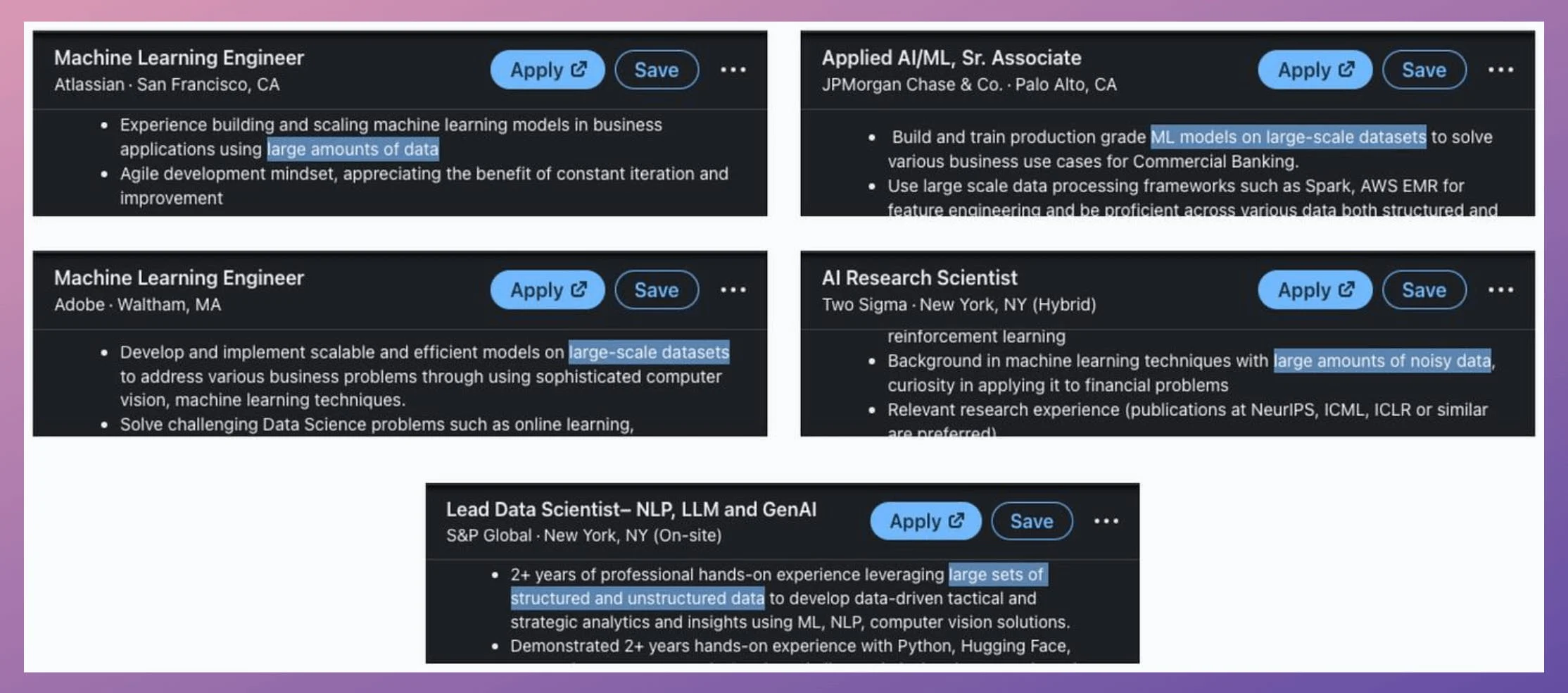
Of course, this is not something new or emerging.
But the reason they explicitly mention “large datasets” is quite simple to understand.
Businesses have more data than ever before.
Traditional single-node model training just doesn’t work because one cannot wait months to train a model.
​Distributed (or multi-GPU) training is one of the most essential ways to address this.
​Here, we covered the core technicalities behind multi-GPU training, how it works under the hood, and implementation details.
We also look at the key considerations for multi-GPU (or distributed) training, which, if not addressed appropriately, may lead to suboptimal performance or slow training.
CRASH COURSE (56 MINS)
Graph Neural Networks
- Google Maps uses graph ML for ETA prediction.
- Pinterest uses graph ML (PingSage) for recommendations.
- Netflix uses graph ML (SemanticGNN) for recommendations.
- Spotify uses graph ML (HGNNs) for audiobook recommendations.
- Uber Eats uses graph ML (a GraphSAGE variant) to suggest dishes, restaurants, etc.
The list could go on since almost every major tech company I know employs graph ML in some capacity.
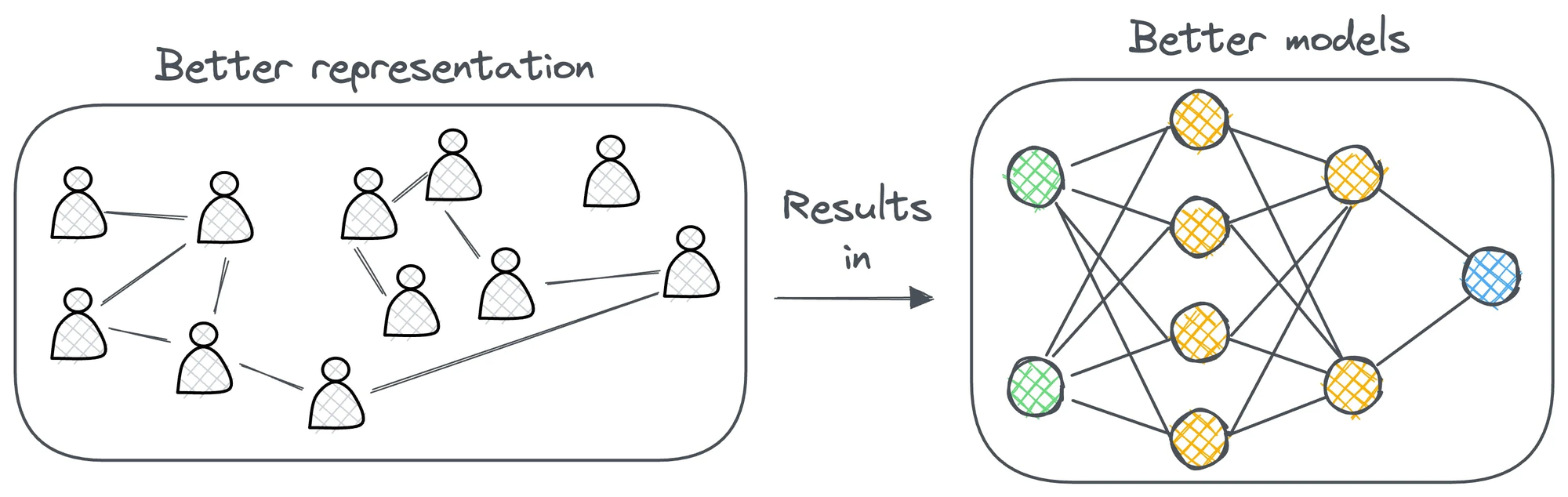
Becoming proficient in ​graph ML​ now seems to be far more critical than traditional deep learning to differentiate your profile and aim for these positions.
A significant proportion of our real-world data often exists (or can be represented) as graphs:
- Entities (nodes) are connected by relationships (edges).
- Connections carry significant meaning, which, if we knew how to model, can lead to much more robust models.
The field of ​graph neural networks (GNNs)​ intends to fill this gap by extending deep learning techniques to graph data.
Learn sophisticated graph architectures and how to train them on graph data in ​this crash course​​ →
THAT'S A WRAP
No-Fluff Industry ML resources to
Succeed in DS/ML roles
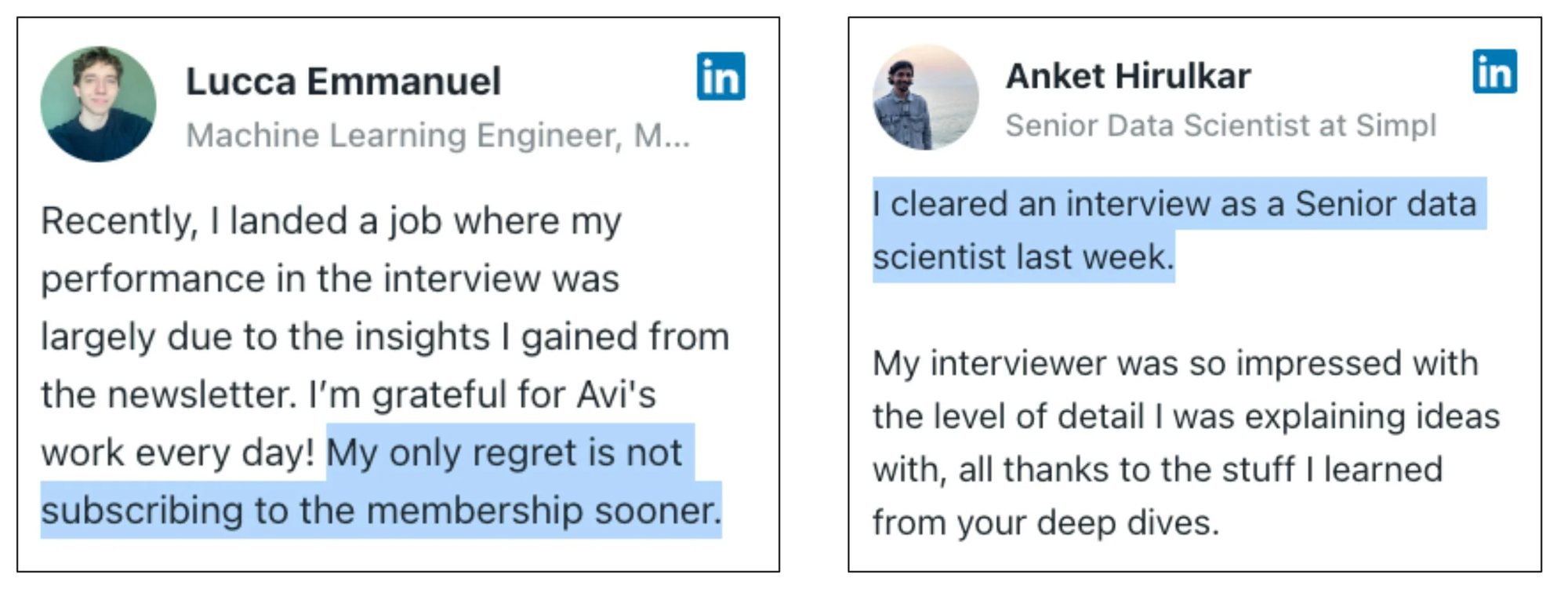
At the end of the day, all businesses care about impact. That’s it!
- Can you reduce costs?
- Drive revenue?
- Can you scale ML models?
- Predict trends before they happen?
We have discussed several other topics (with implementations) in the past that align with such topics.
Here are some of them:
- Learn sophisticated graph architectures and how to train them on graph data in this crash course.
- So many real-world NLP systems rely on pairwise context scoring. Learn scalable approaches here.
- Run large models on small devices using Quantization techniques.
- Learn how to generate prediction intervals or sets with strong statistical guarantees for increasing trust using Conformal Predictions.
- Learn how to identify causal relationships and answer business questions using causal inference in this crash course.
- Learn how to scale and implement ML model training in this practical guide.
- Learn 5 techniques with implementation to reliably test ML models in production.
- Learn how to build and implement privacy-first ML systems using Federated Learning.
- Learn 6 techniques with implementation to compress ML models.
All these resources will help you cultivate key skills that businesses and companies care about the most.
SPONSOR US
Advertise to 600k+ data professionals
Our newsletter puts your products and services directly in front of an audience that matters — thousands of leaders, senior data scientists, machine learning engineers, data analysts, etc., around the world.