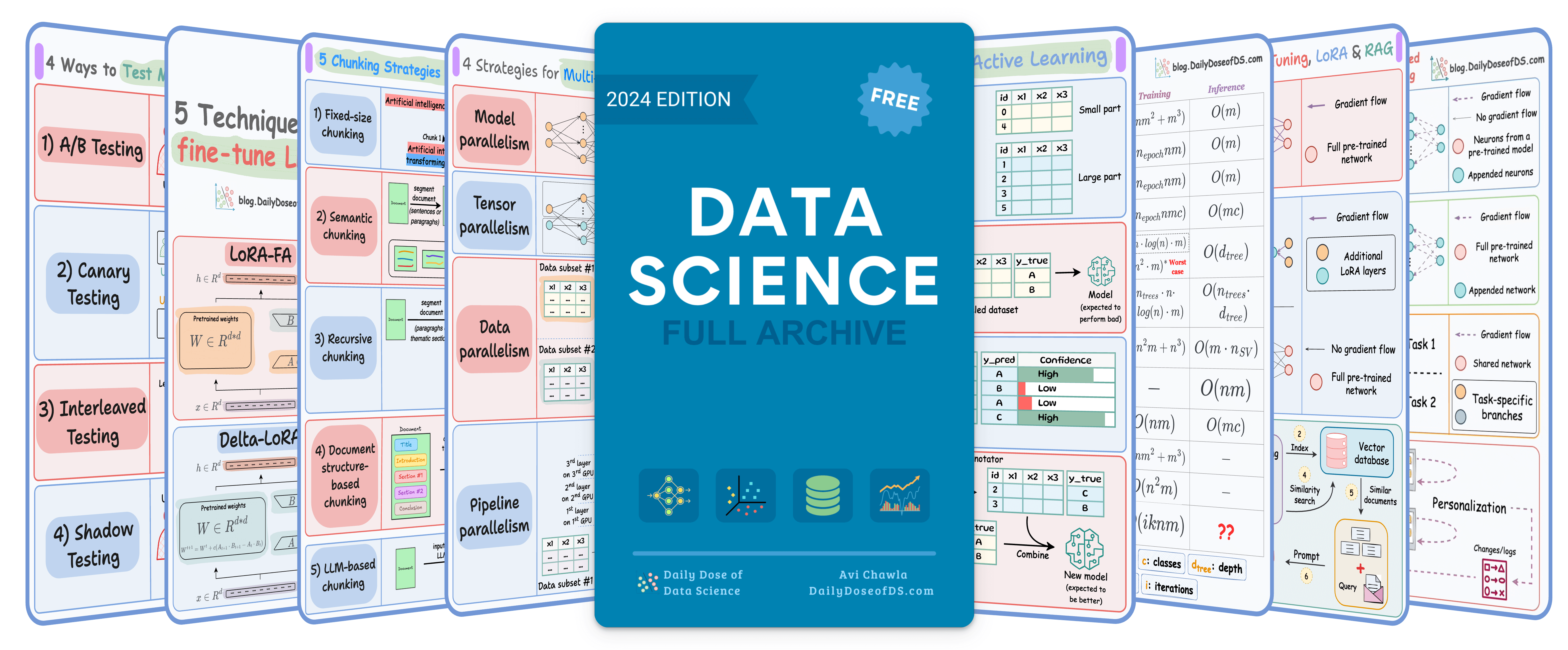
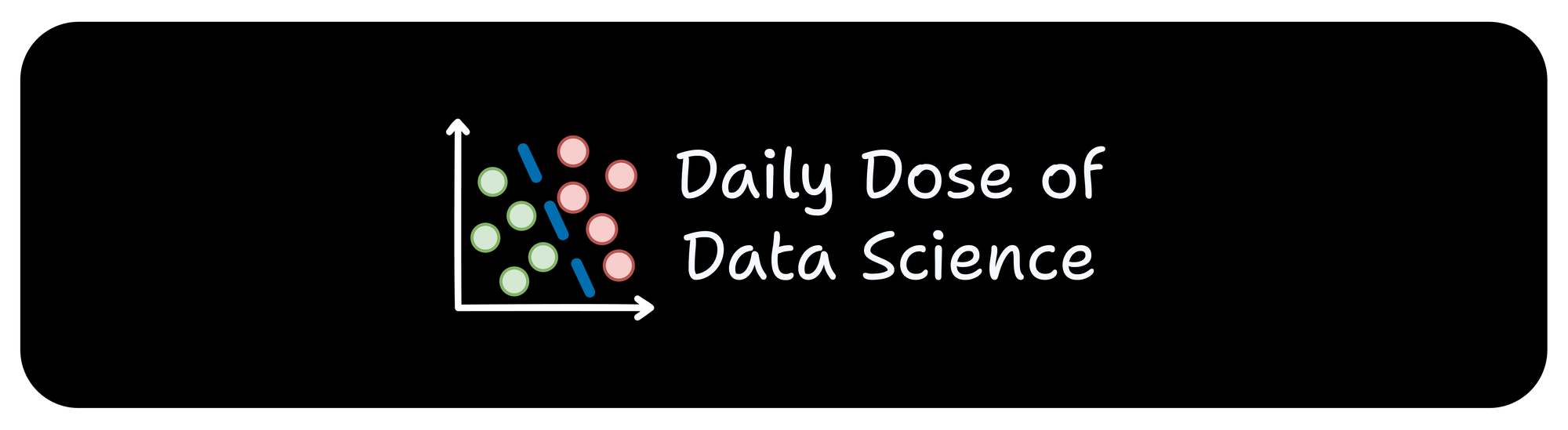
TODAY'S ISSUE
TODAYβS DAILY DOSE OF DATA SCIENCE
5 Levels of Agentic AI Systems
Agentic AI systems don't just generate text; they can make decisions, call functions, and even run autonomous workflows.
The visual explains 5 levels of AI agencyβfrom simple responders to fully autonomous agents.
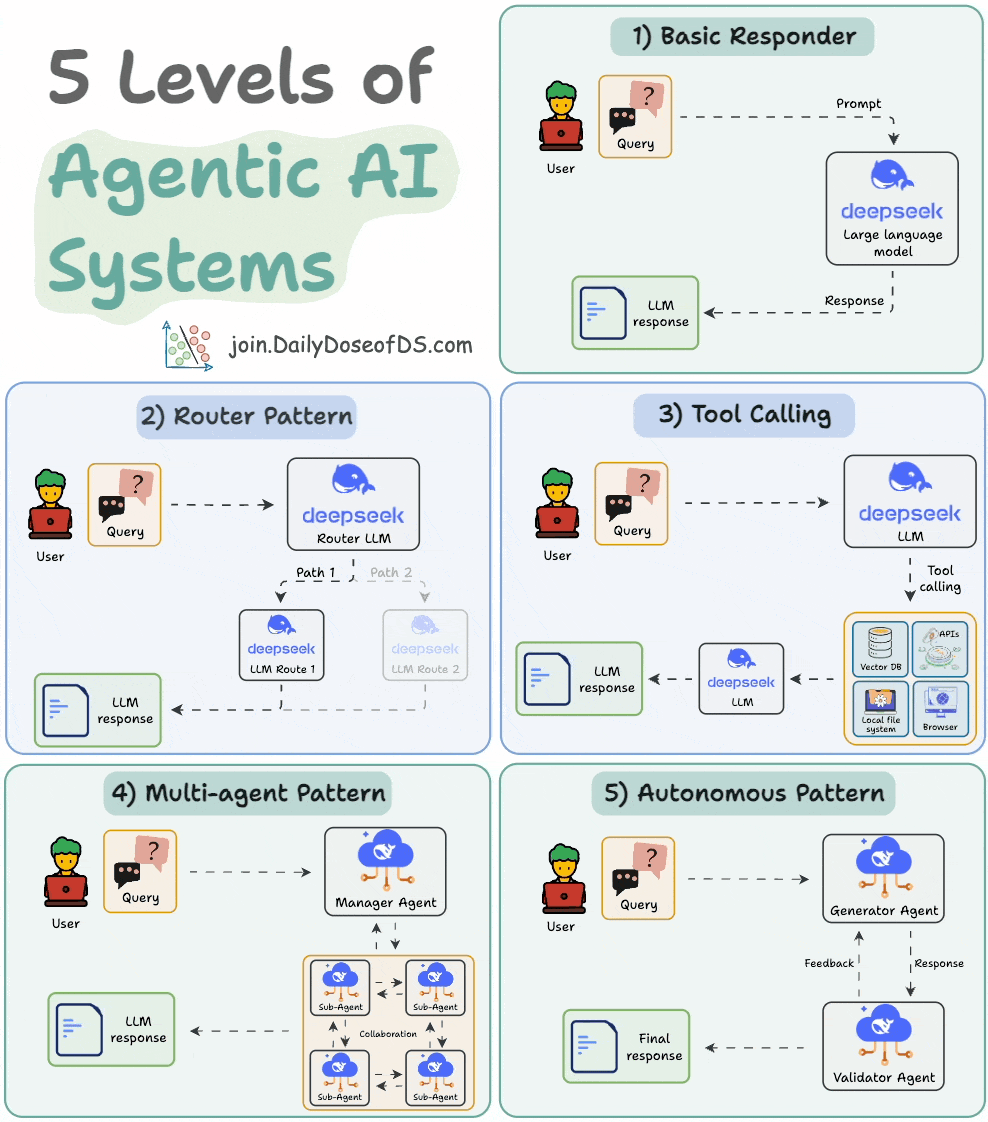
Note: If you want to learn how to build Agentic systems, we have published 6 parts so far in our Agents crash course (with implementation):
- βPart 1 β Why Agents? Creating Agents, Tasks, Tools, etc.β
- βPart 2 β Modular agents, custom tools, structured outputs, etc.β
- βPart 3 β Agentic Flows.β
- βPart 4 β Hands-on deep dive on building 2 agentic Flows systems.β
- Part 5β and βPart 6β β Advanced techniques to build robust Agents.
Letβs dive in to learn more about the 5 levels of Agentic AI systems.
1) Basic responder
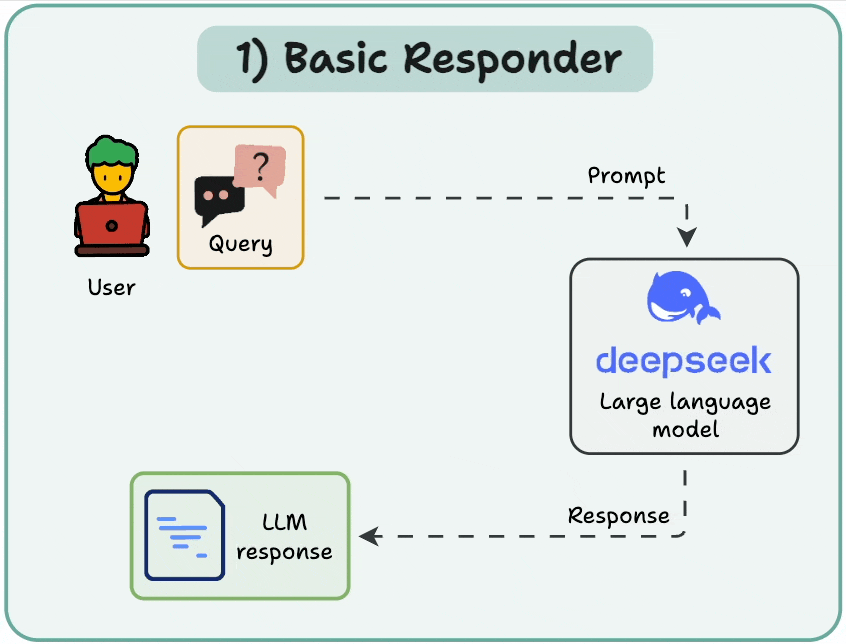
- A human guides the entire flow.
- The LLM is just a generic responder that receives an input and produces an output. It has little control over the program flow.
2) Router pattern
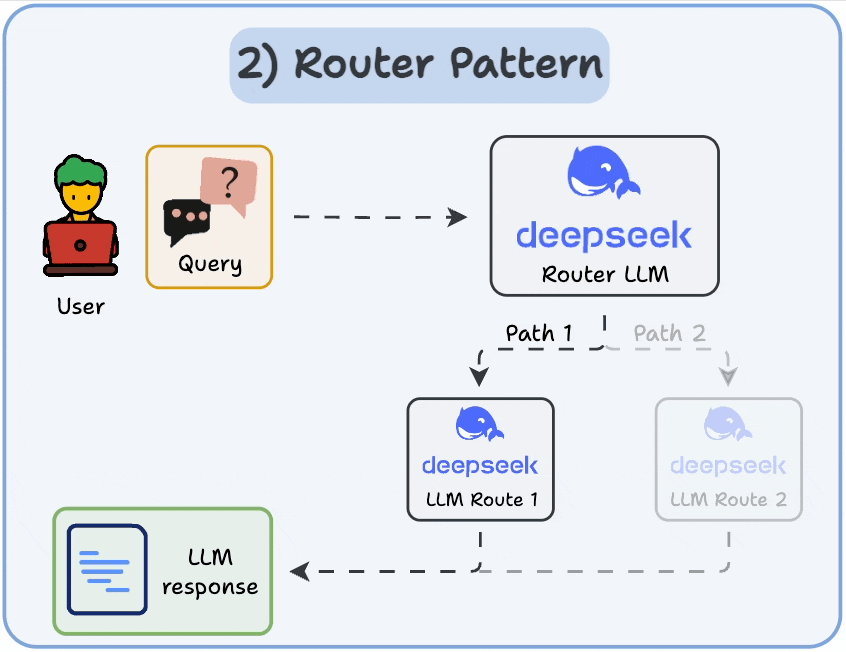
- A human defines the paths/functions that exist in the flow.
- The LLM makes basic decisions on which function or path it can take.
3) Tool calling
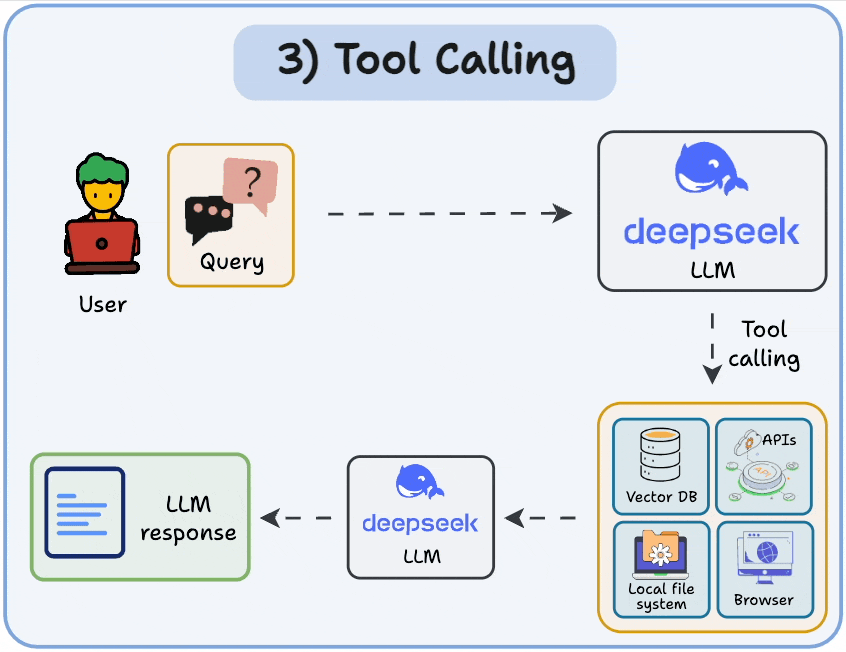
- A human defines a set of tools the LLM can access to complete a task.
- LLM decides when to use them and also the arguments for execution.
4) Multi-agent pattern
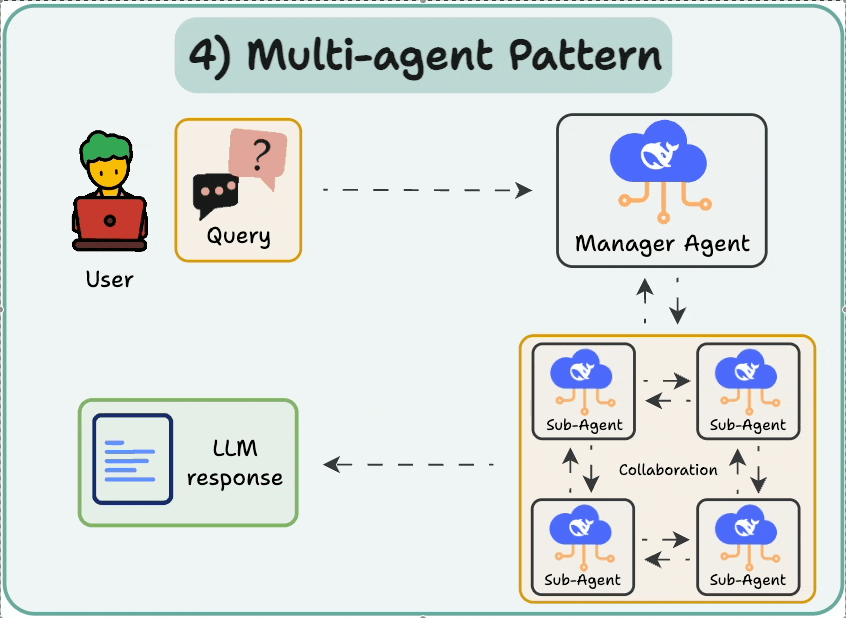
A manager agent coordinates multiple sub-agents and decides the next steps iteratively.
- A human lays out the hierarchy between agents, their roles, tools, etc.
- The LLM controls execution flow, deciding what to do next.
5) Autonomous pattern
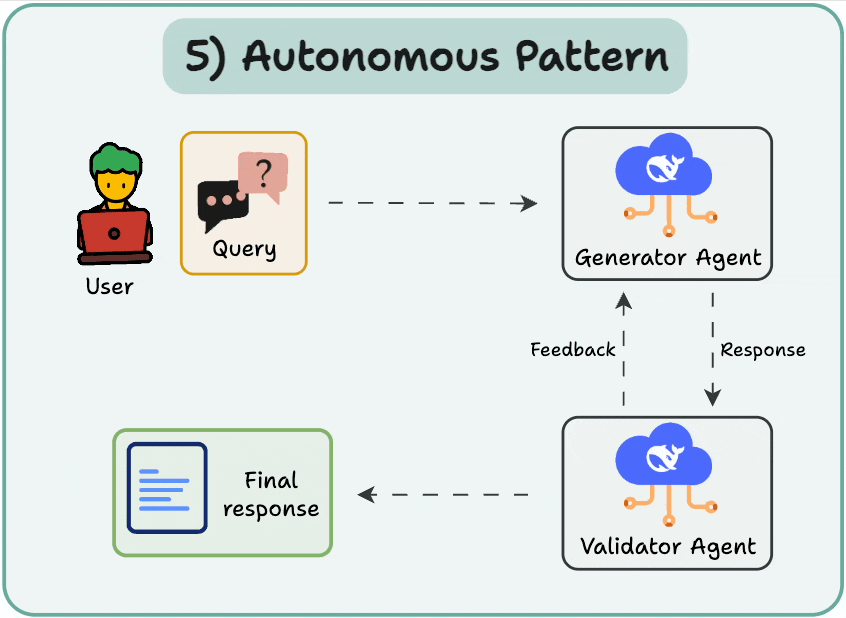
The most advanced pattern, wherein, the LLM generates and executes new code independently, effectively acting as an independent AI developer.
To recall:
- Basic responder only generates text.
- Router pattern decides when to take a path.
- Tool calling picks & runs tools.
- Multi-Agent pattern manages several agents.
- Autonomous pattern works fully independently.
π Over to you: Which one do you use the most?
Note: If you want to learn how to build Agentic systems, we have published 6 parts so far in our Agents crash course (with implementation):
- βPart 1 β Why Agents? Creating Agents, Tasks, Tools, etc.β
- βPart 2 β Modular agents, custom tools, structured outputs, etc.β
- βPart 3 β Agentic Flows.β
- βPart 4 β Hands-on deep dive on building 2 agentic Flows systems.β
- Part 5β and βPart 6β β Advanced techniques to build robust Agents.
IN CASE YOU MISSED IT
βBuild a Reasoning Model Like DeepSeek-R1β
If you have used DeepSeek-R1 (or any other reasoning model), you must have seen that they autonomously allocate thinking time before producing a response.
Last week, we shared how to embed reasoning capabilities into any LLM.
We trained our own reasoning model like DeepSeek-R1 (with code).
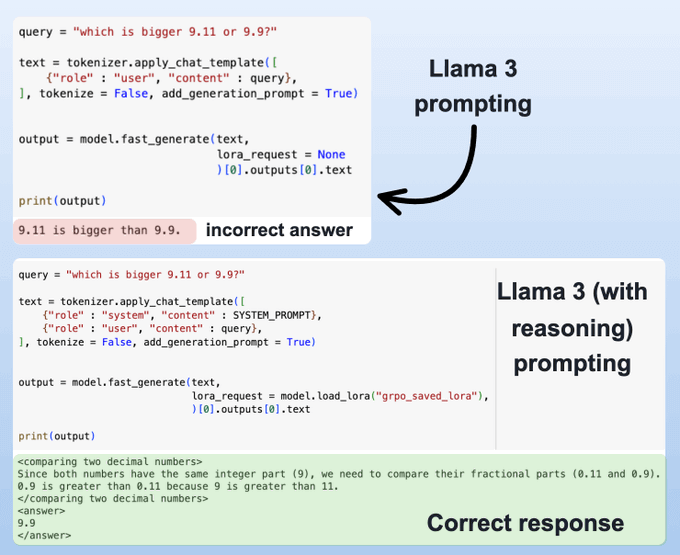
To do this, we used:
- UnslothAI for efficient fine-tuning.
- Llama 3.1-8B as the LLM to add reasoning capabilities to.
βFind the implementation and detailed walkthrough newsletter here ββ
THAT'S A WRAP
No-Fluff Industry ML resources to
Succeed in DS/ML roles
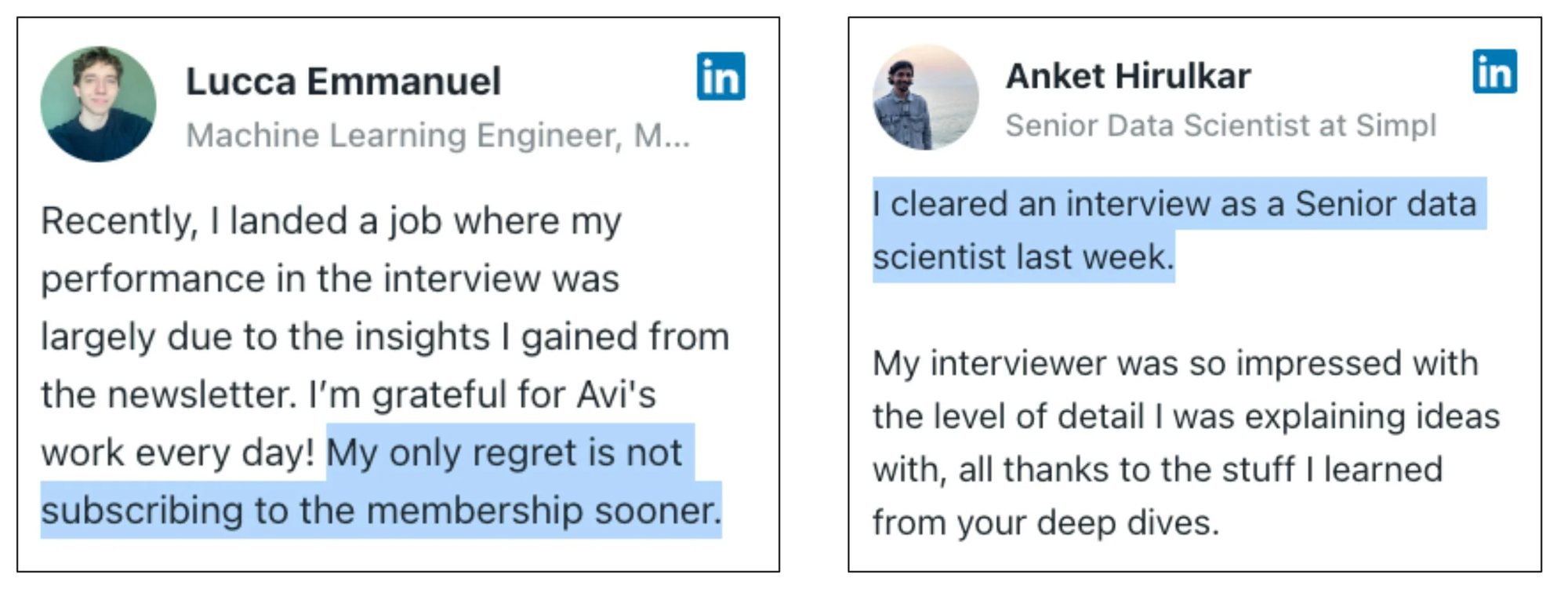
At the end of the day, all businesses care about impact. Thatβs it!
- Can you reduce costs?
- Drive revenue?
- Can you scale ML models?
- Predict trends before they happen?
We have discussed several other topics (with implementations) in the past that align with such topics.
Here are some of them:
- Learn sophisticated graph architectures and how to train them on graph data in this crash course.
- So many real-world NLP systems rely on pairwise context scoring. Learn scalable approaches here.
- Run large models on small devices using Quantization techniques.
- Learn how to generate prediction intervals or sets with strong statistical guarantees for increasing trust using Conformal Predictions.
- Learn how to identify causal relationships and answer business questions using causal inference in this crash course.
- Learn how to scale and implement ML model training in this practical guide.
- Learn 5 techniques with implementation to reliably test ML models in production.
- Learn how to build and implement privacy-first ML systems using Federated Learning.
- Learn 6 techniques with implementation to compress ML models.
All these resources will help you cultivate key skills that businesses and companies care about the most.
SPONSOR US
Advertise to 600k+ data professionals
Our newsletter puts your products and services directly in front of an audience that matters β thousands of leaders, senior data scientists, machine learning engineers, data analysts, etc., around the world.