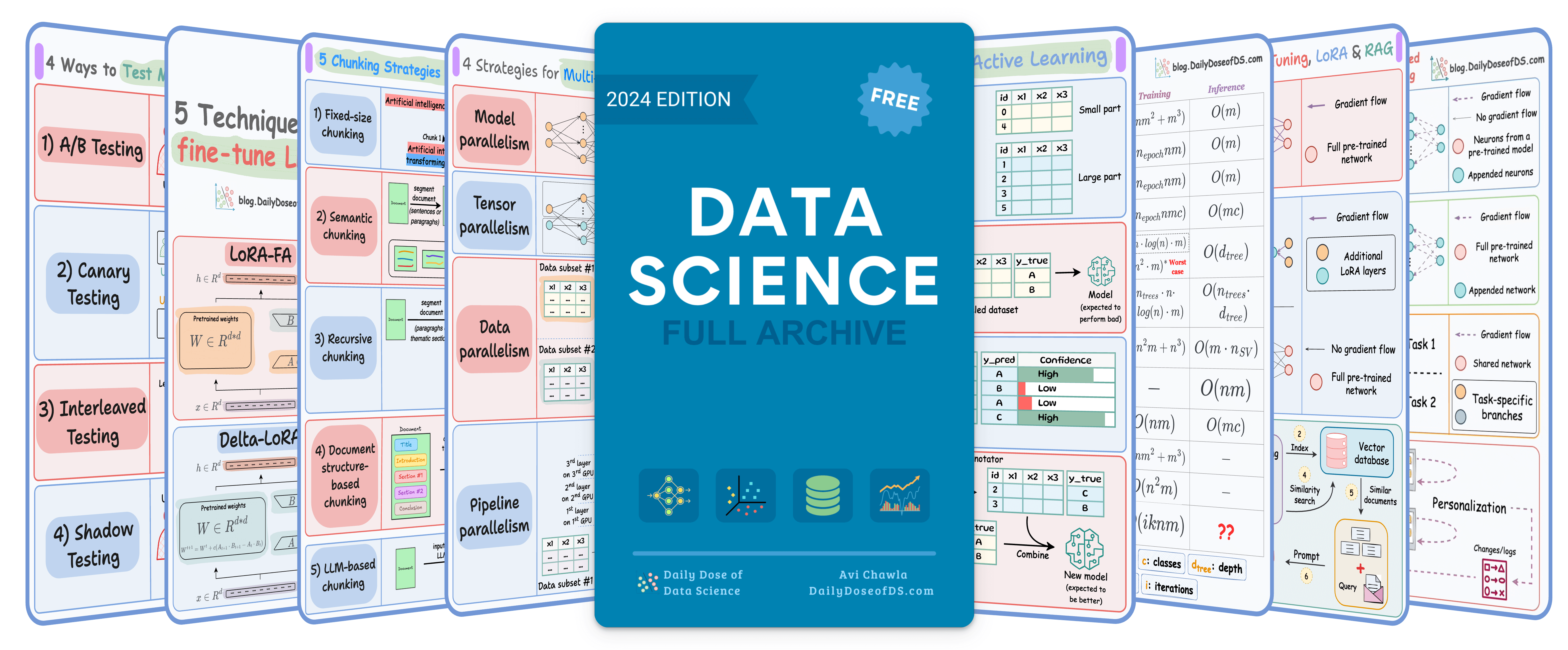
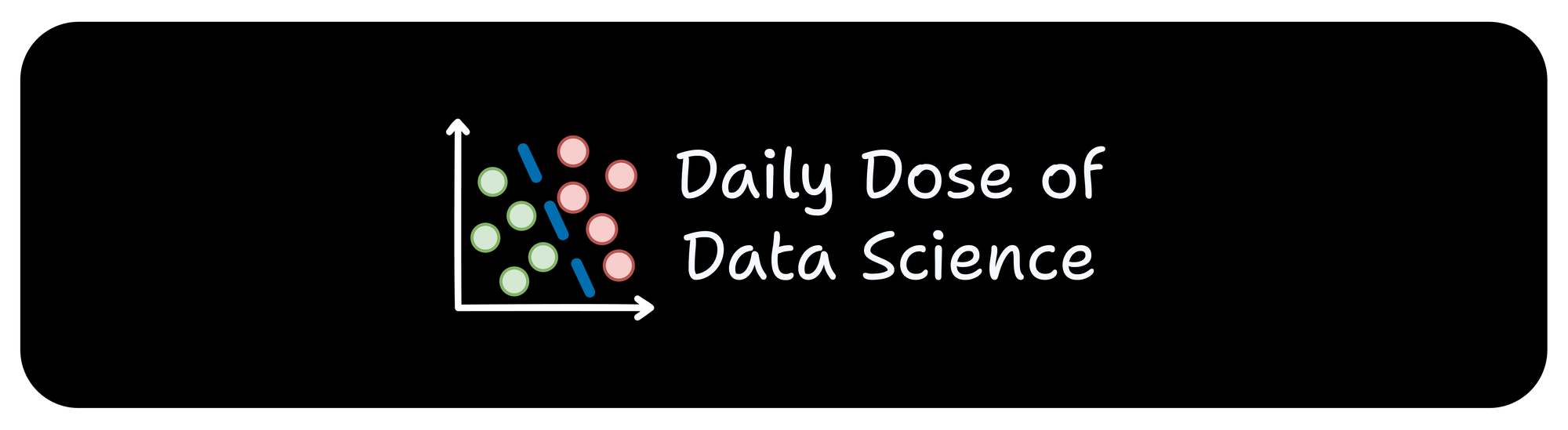
TODAY'S ISSUE
TODAY’S DAILY DOSE OF DATA SCIENCE
Implementing a Siamese Network
In yesterday’s issue, we learned how a Siamese network trained using contrastive loss can help us build a face unlock system.
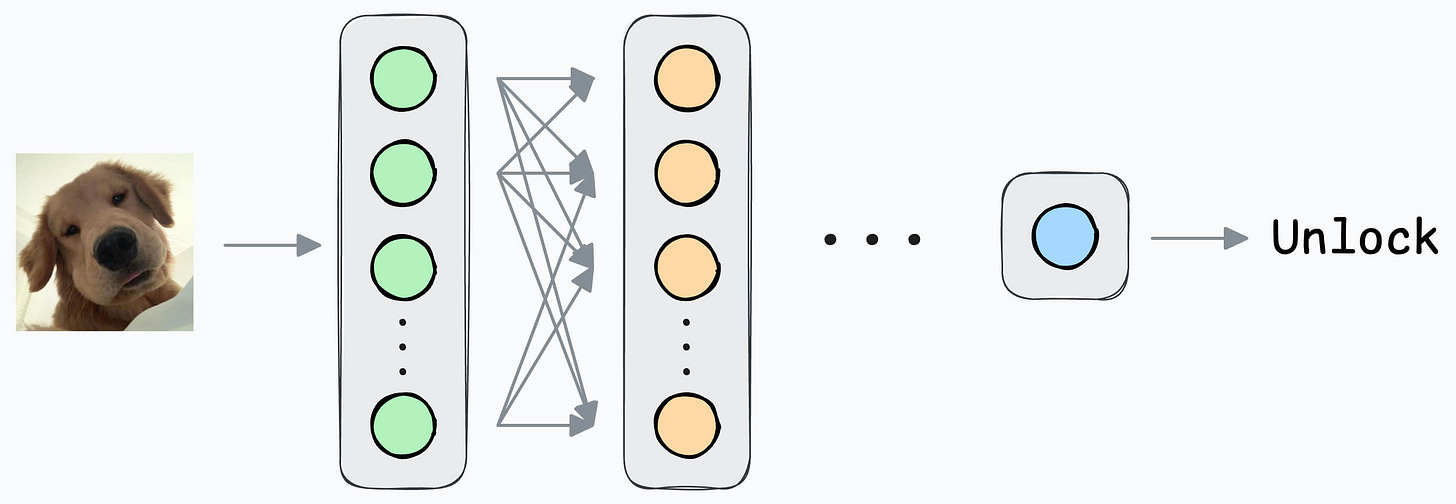
Today, let’s understand the implementation.
Quick recap
If you haven’t read yesterday’s issue, I highly recommend it before reading ahead. It will help you understand the true motivation behind WHY we are using it:
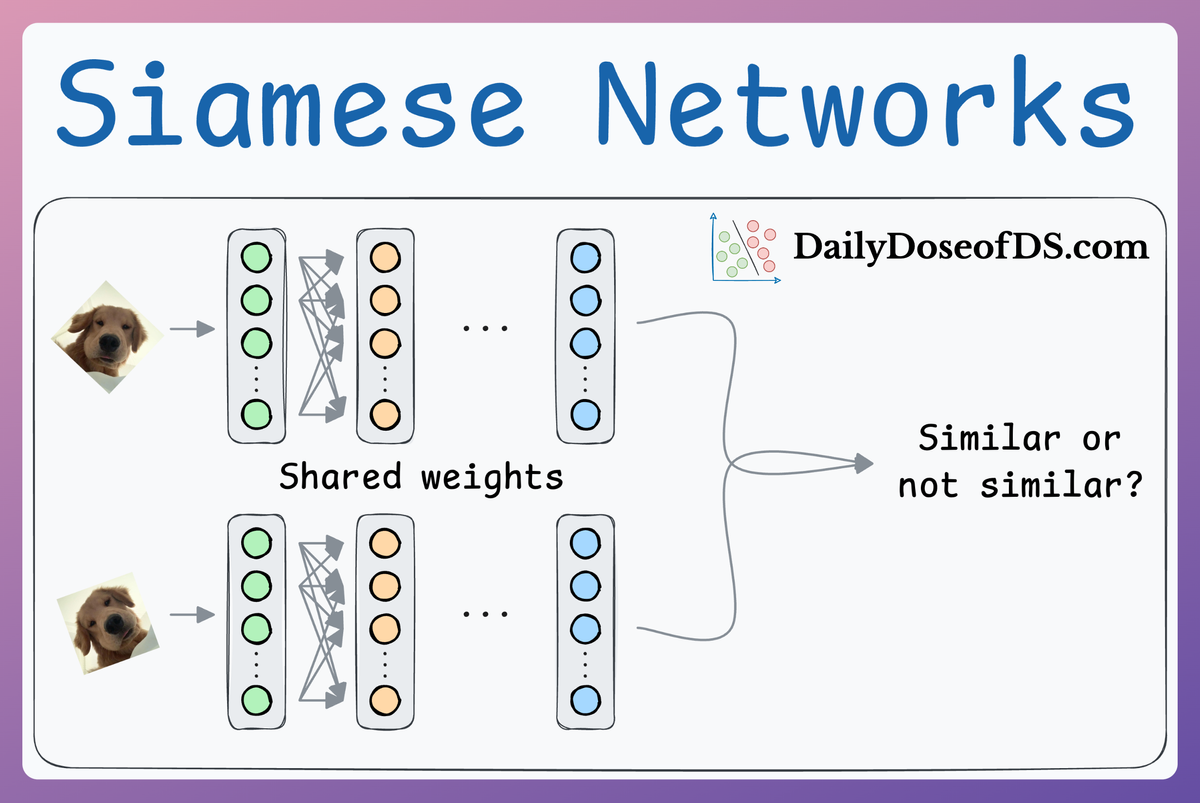
Here’s a quick recap of the overall idea:
- If a pair belongs to different entities, the true label will be 1.
- If a pair belongs to the same entity, the true label will be 0.
- Pass both inputs through the same network to generate two embeddings.
- If the true label is 0 (same entity) → minimize the distance between the two embeddings.
- If the true label is 1 (different entities) → maximize the distance between the two embeddings.
Next, define a network like this:
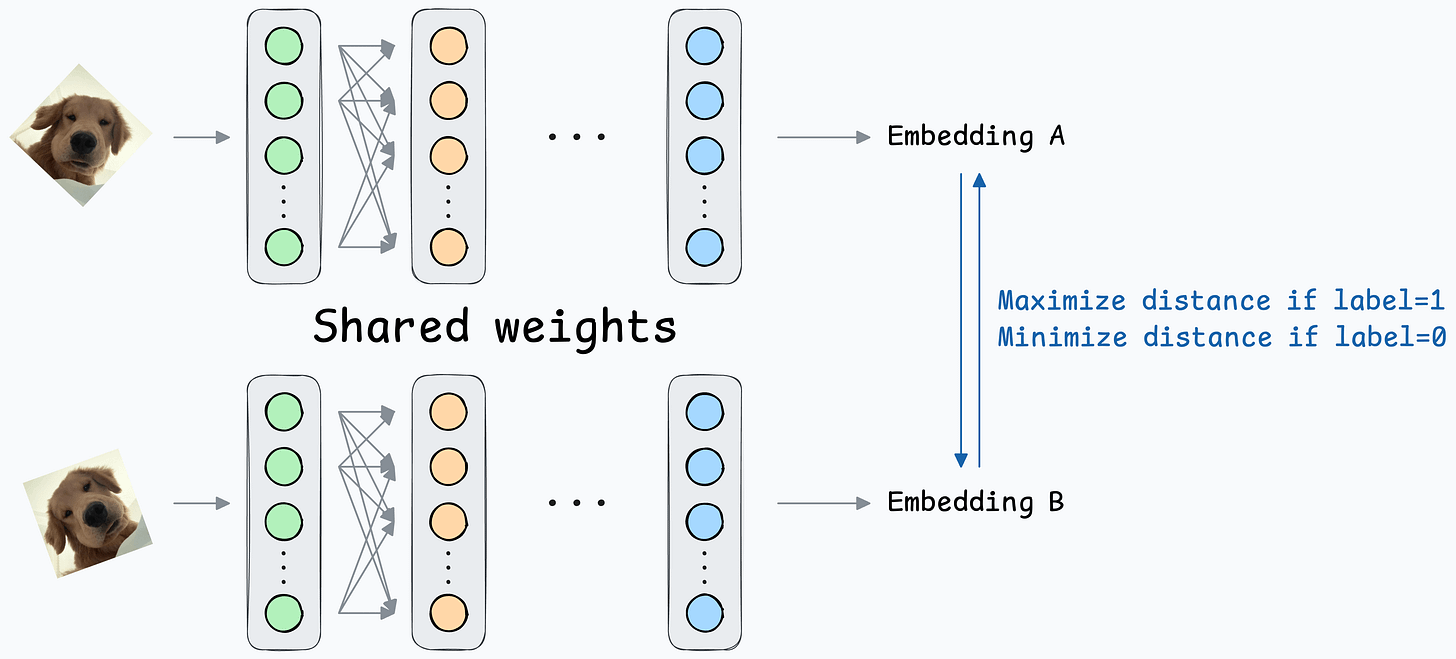
Create a dataset of face pairs:
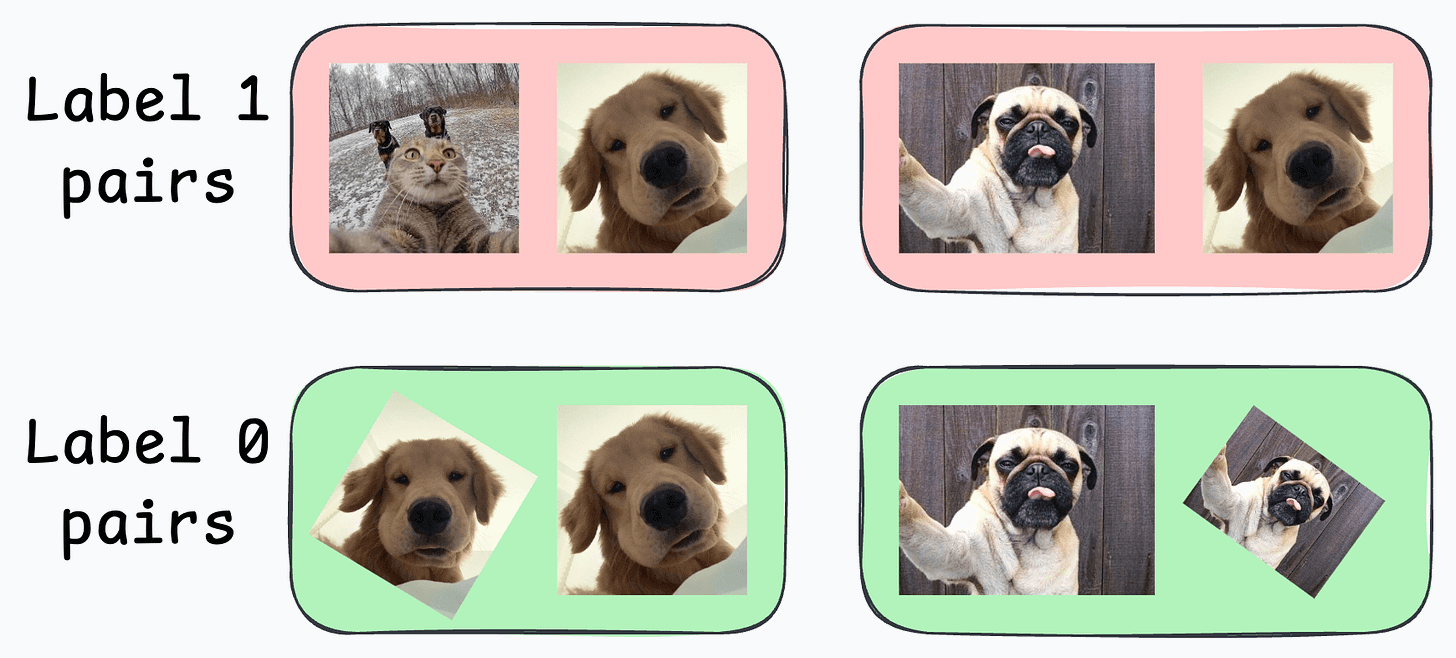
Contrastive loss (defined below) helps us train such a model:

where:
y
is the true label.D
is the distance between two embeddings.margin
is a hyperparameter, typically greater than 1.
Implementation
Next, let’s look at the implementation of this model.
For simplicity, we shall begin with a simple implementation utilizing the MNIST dataset. In a future issue, we shall explore the face unlock model.
Let’s implement it.
As always, we start with some standard imports:
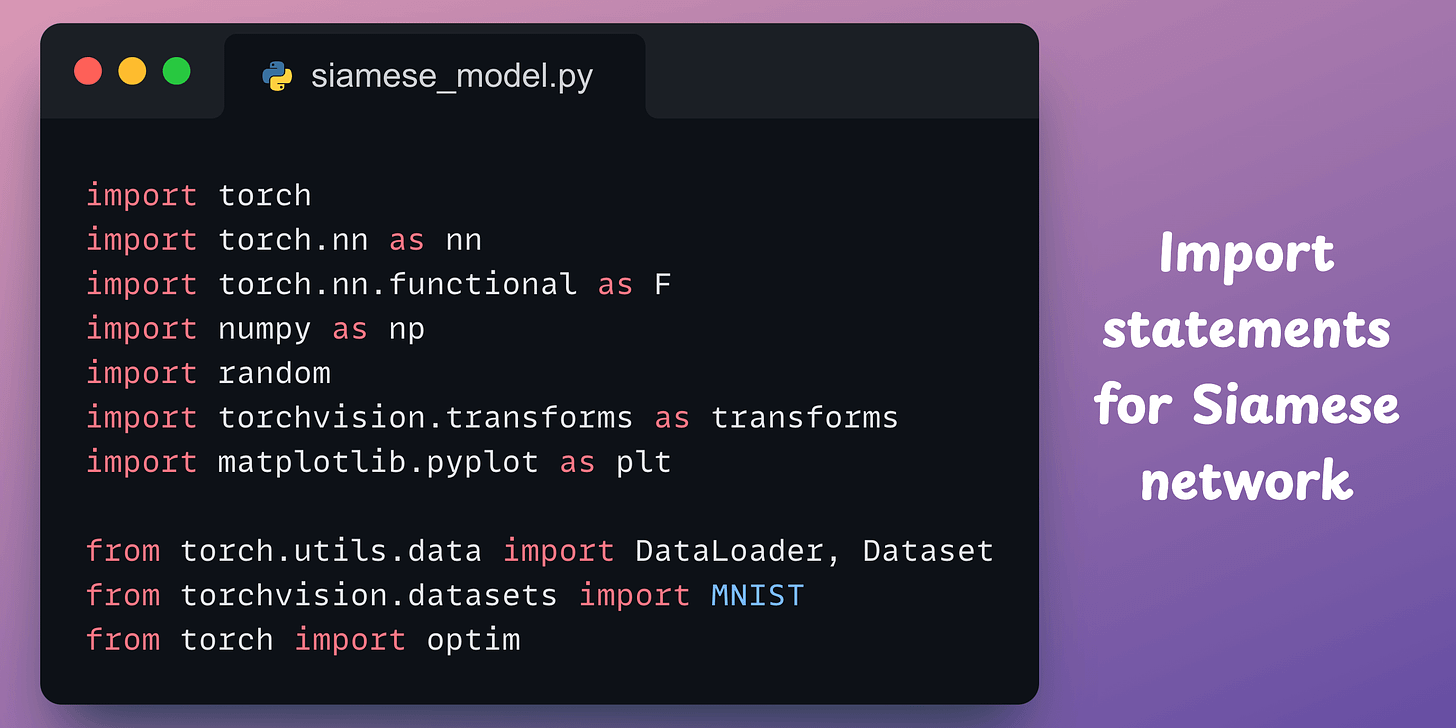
Next, we download/load the MNIST dataset:
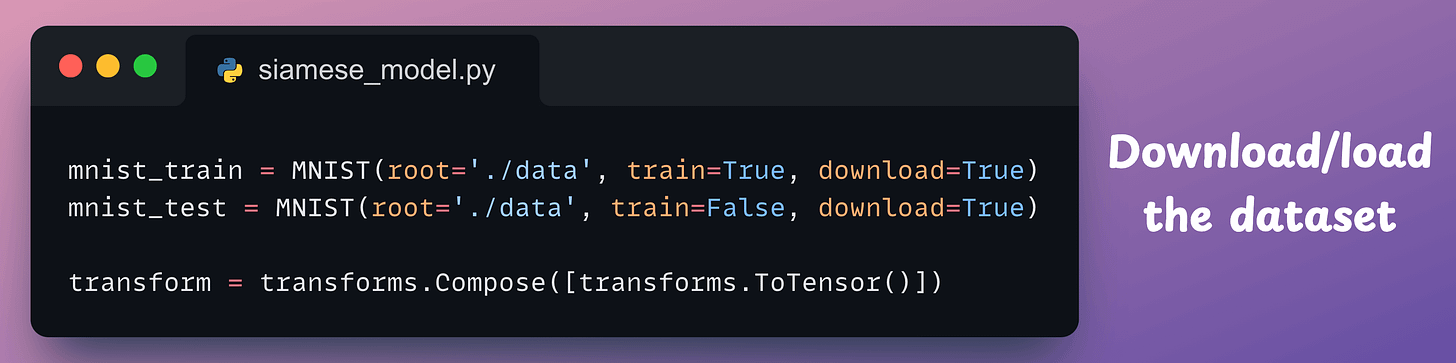
Now, recall to build a Siamese network, we have to create image pairs:
- In some pairs, the two images will have the same true label.
- In other pairs, the two images will have a different true label.
To do this, we define a SiameseDataset
class that inherits from the Dataset class of PyTorch:
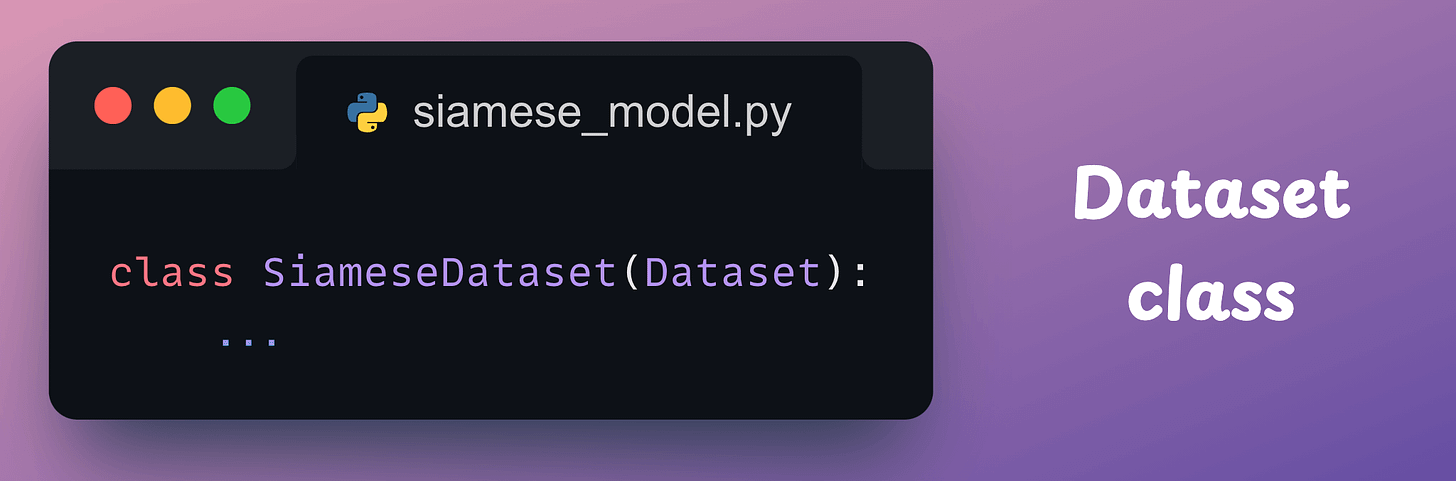
This class will have three methods:
- The
__init__
method:
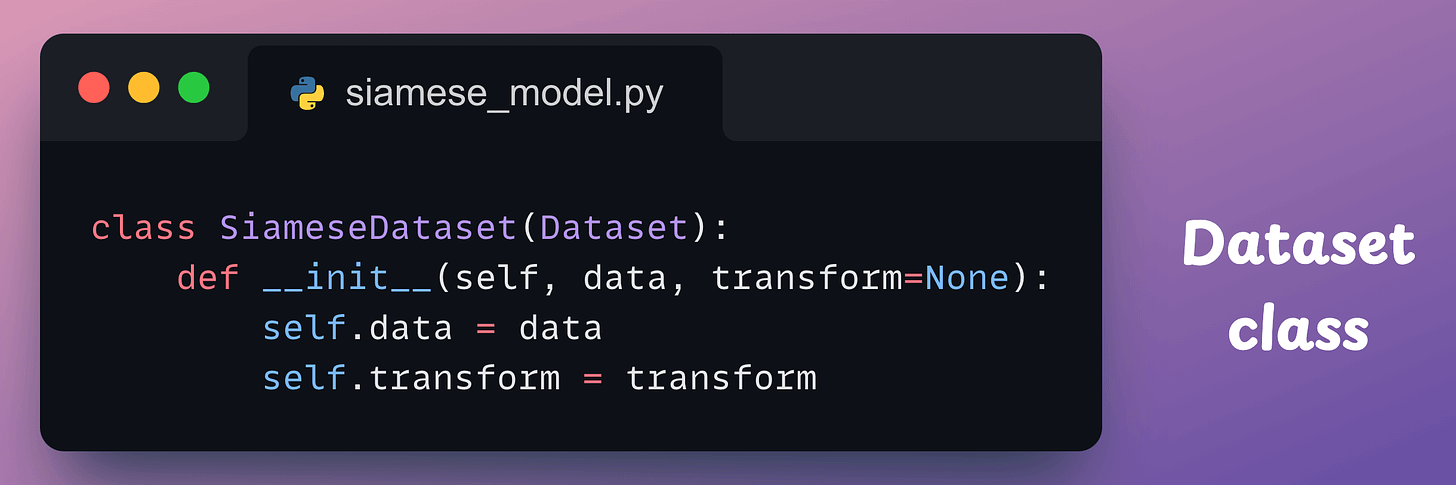
data
parameter will be mnist_train
and mnist_test
defined earlier.- The
__len__
method:
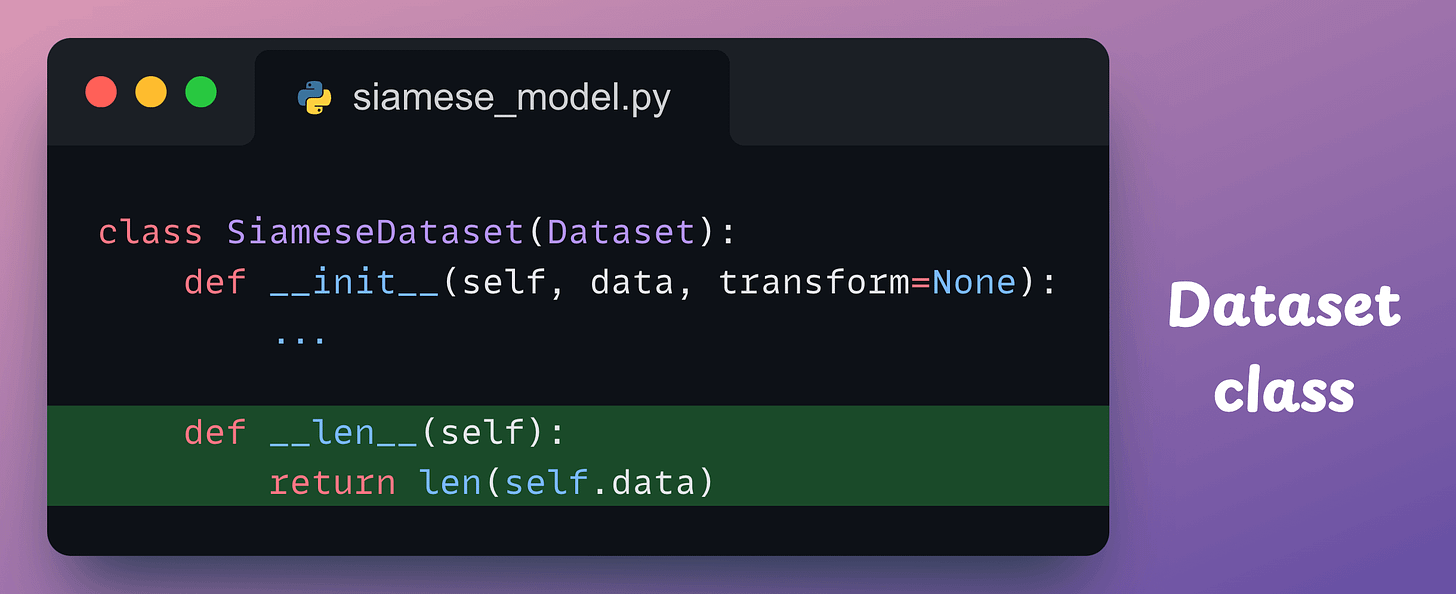
- The
__getitem__
method, which is used to return an instance of the train data. In our case, we shall pair the current instance from the training dataset with:- Either another image from the same class as the current instance.
- Or another image from a different class.
- Which class to pair with will be decided randomly.
The __getitem__
method is implemented below:
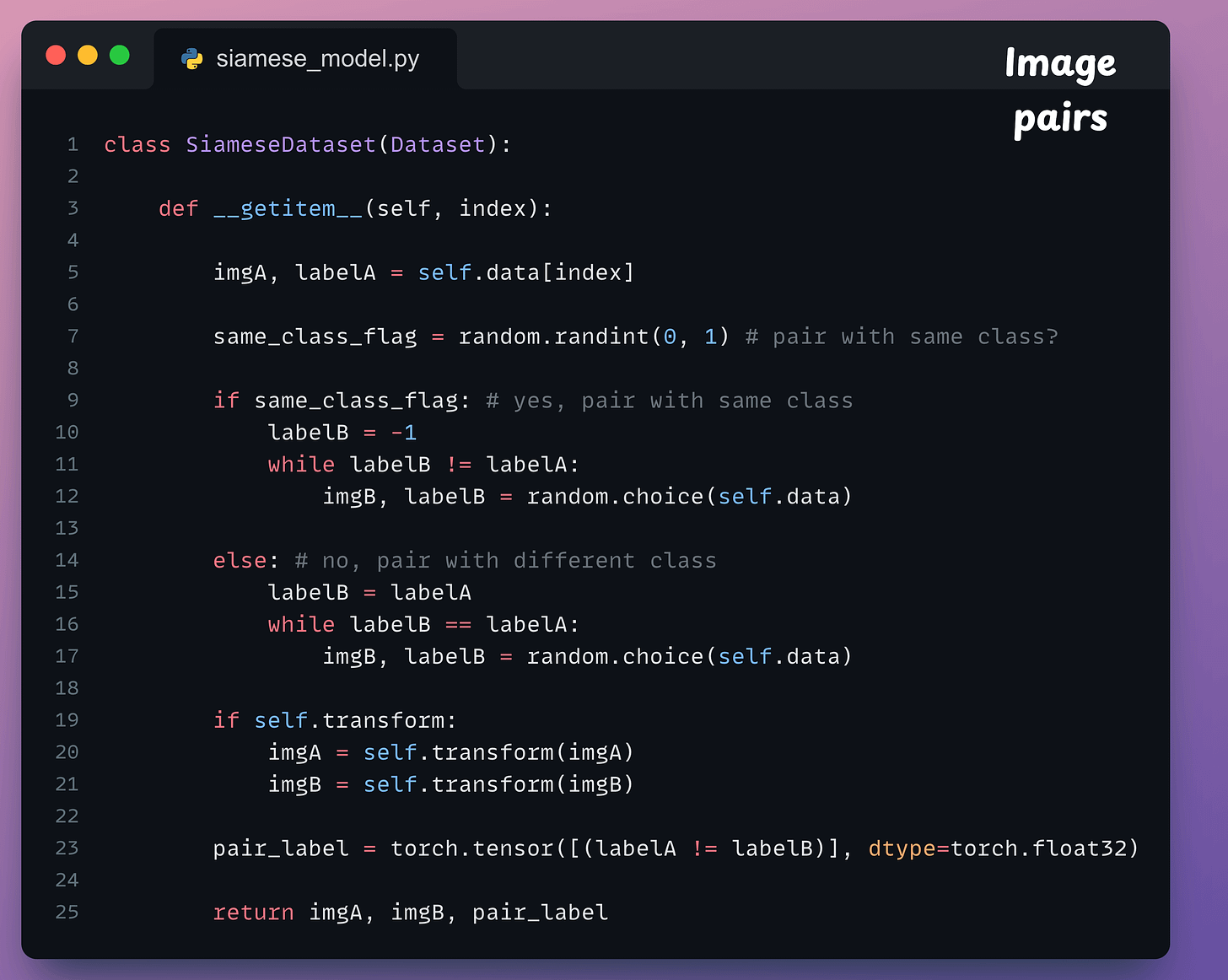
- Line 5: We obtain the current instance.
- Line 7: We randomly decide whether this instance should be paired with the same class or not.
- Lines 9-12: If
flag=1
, continue to find an instance until we get an instance of the same class. - Lines 14-17: If
flag=0
, continue to find an instance until we get an instance of a different class. - Line 19-21: Apply transform if needed.
- Line 23:
- If the two labels are different, the true label for the pair will be 1.
- If the two labels are the same, the true label for the pair will be 0.
After defining the class, we create the dataset objects below:
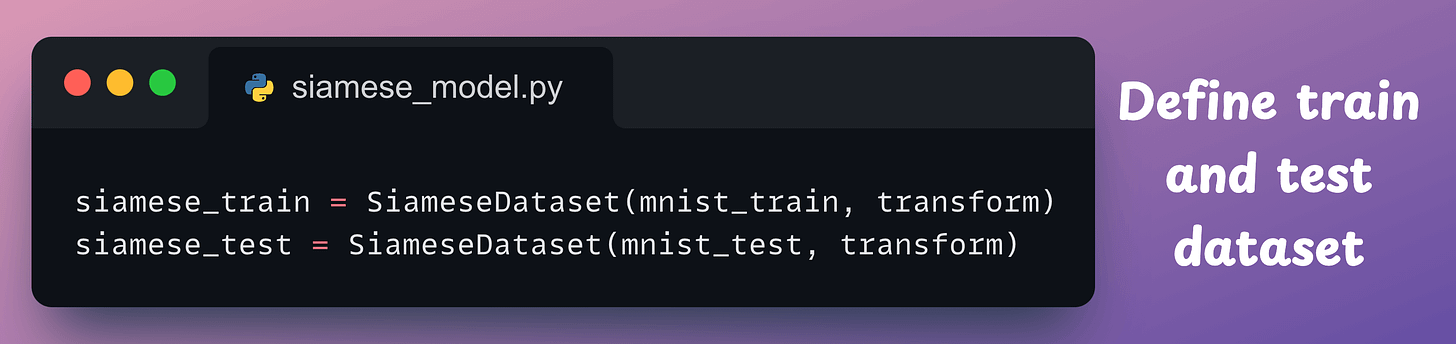
Next, we define the neural network:
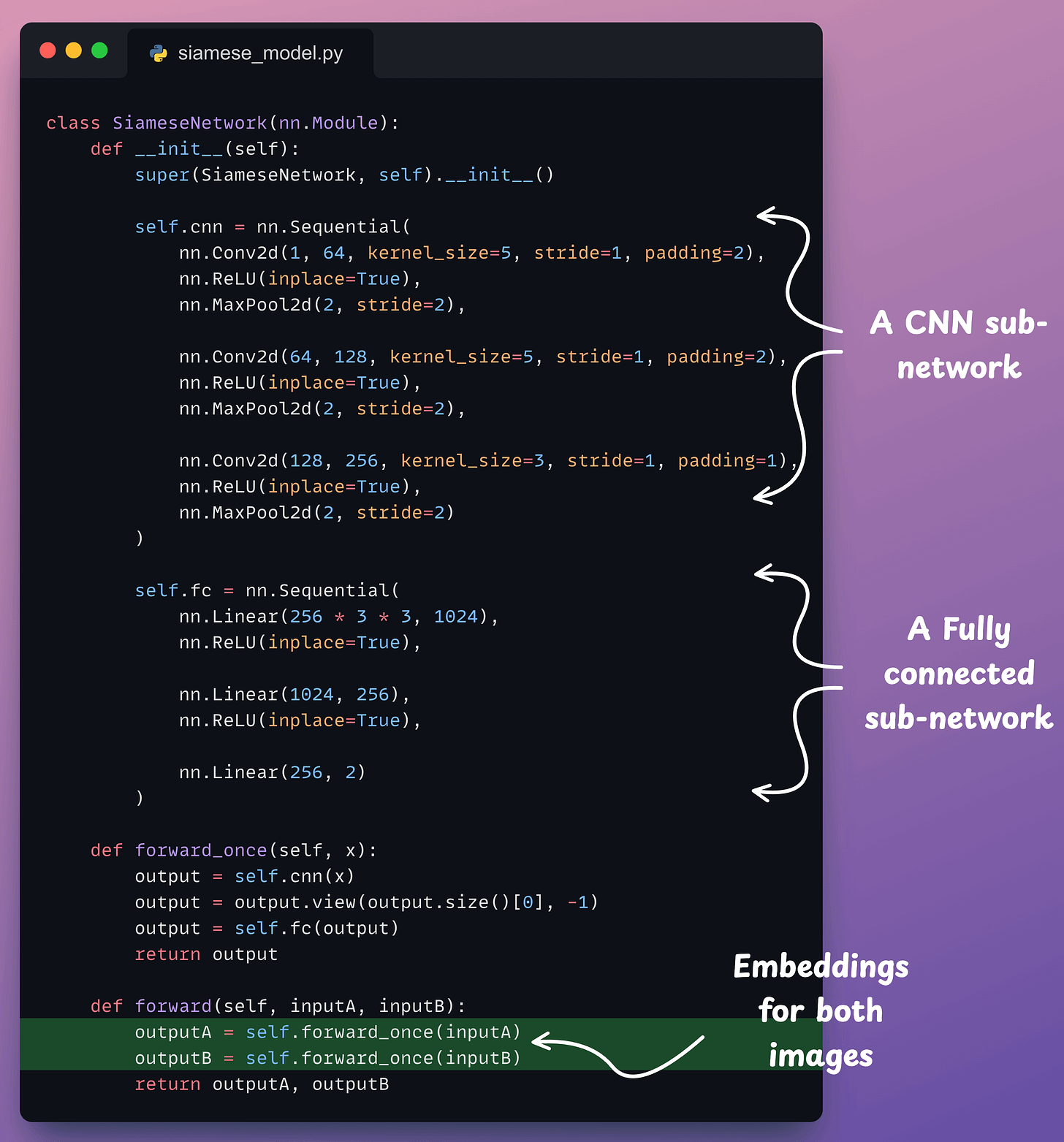
- The two input images are fed through the same network to generate an embedding (
outputA
andoutputB
).
Moving on, we define the contrastive loss:
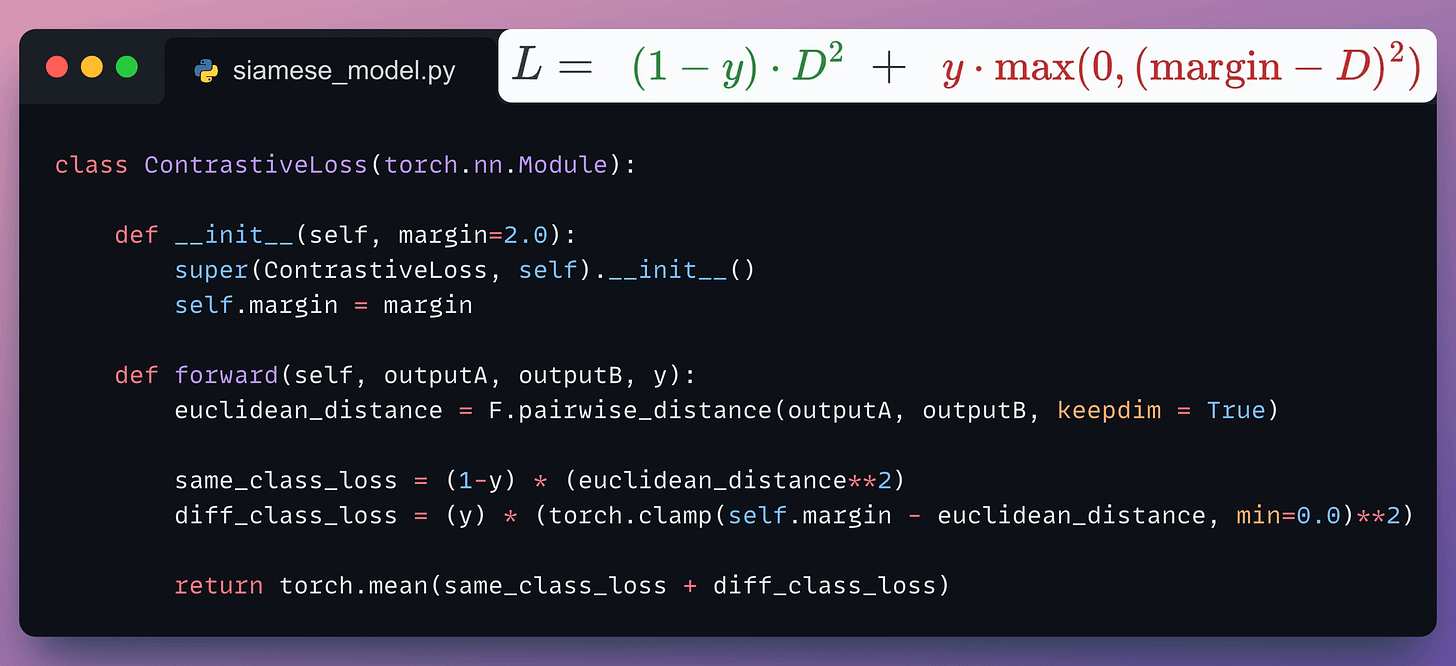
Almost done!
Next, we define the dataloader, the model, the optimizer, and the loss function:
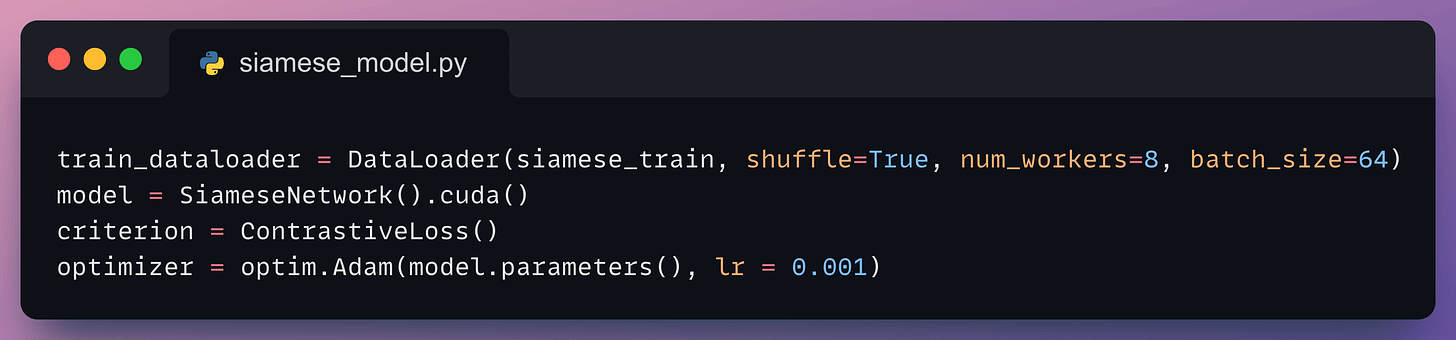
Finally, we train it:
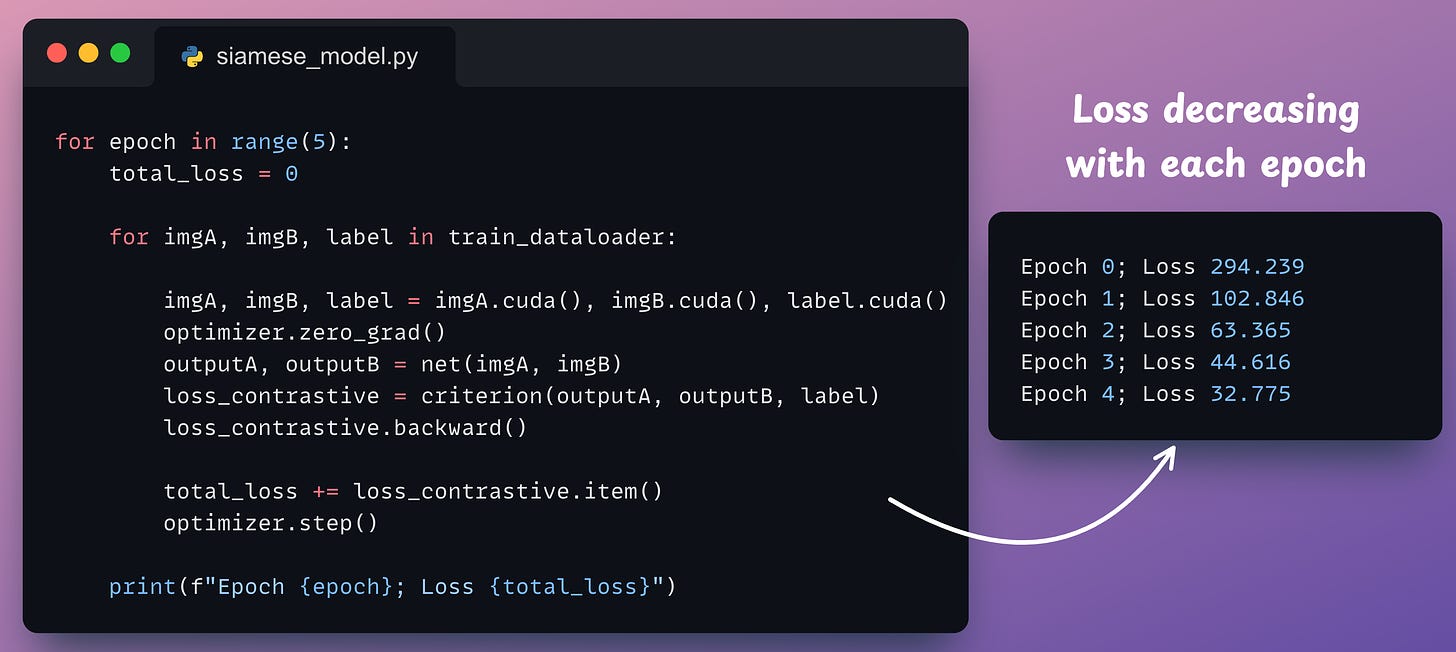
And with that, we have successfully implemented a Siamese Network using PyTorch.
Results
Let’s look at some results using images in the test dataset:
We can generate a similarity score as follows:

- Image pair #1: Similarity is high since both images depict the same digit:
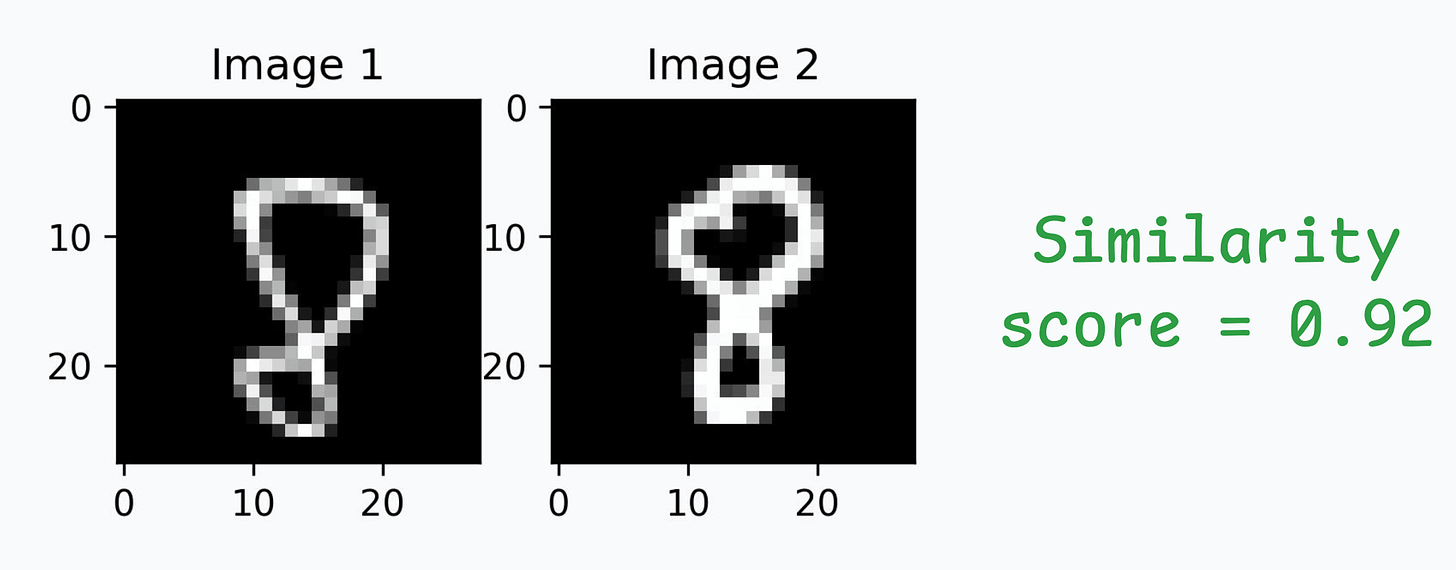
- Image pair #2: Similarity is low since both images depict different digits:
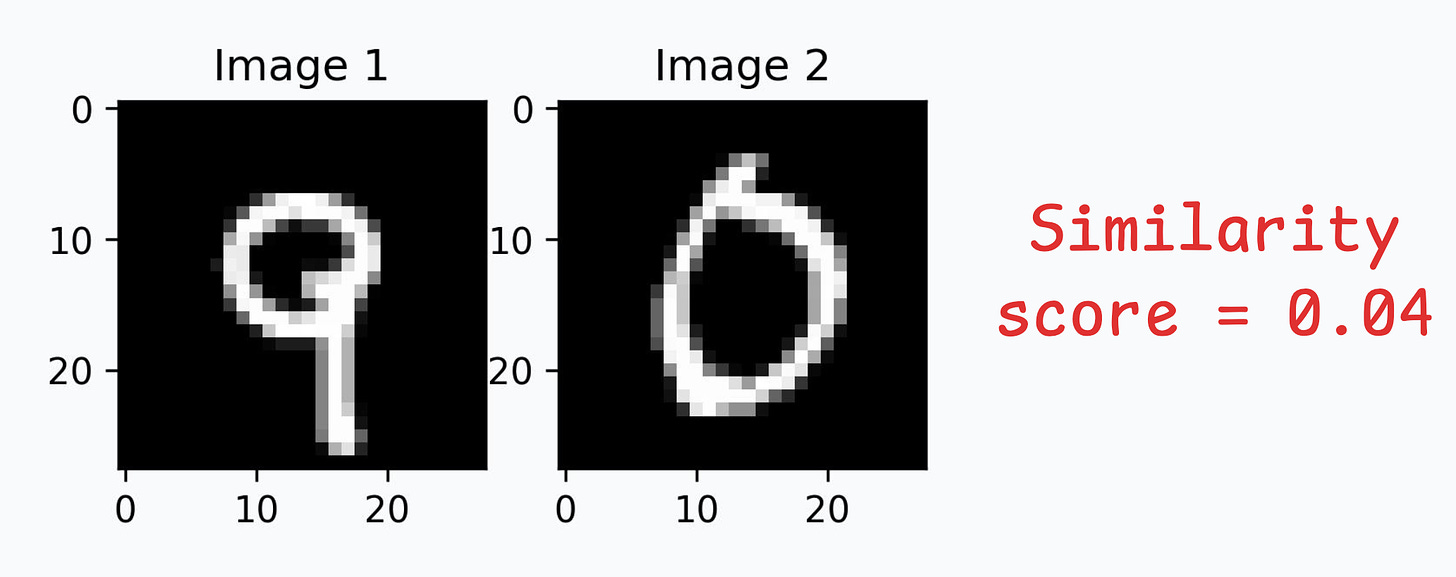
- Image pair #3: Similarity is high since both images depict the same digit:
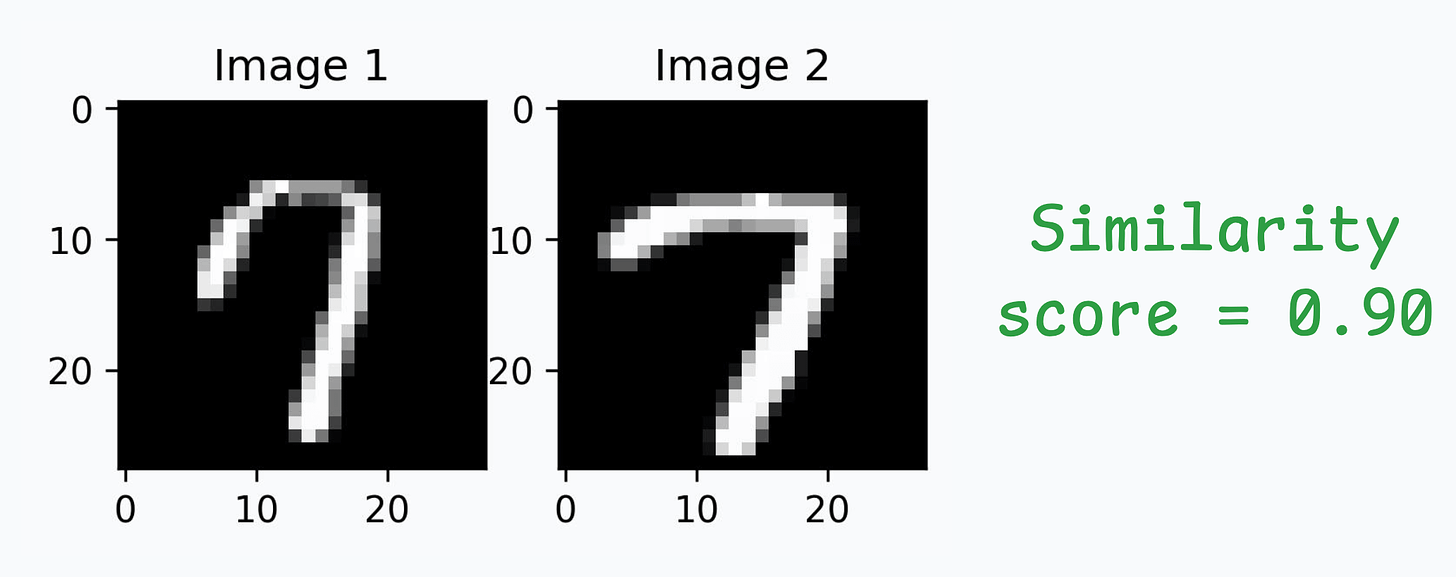
- Image pair #4: Similarity is low since both images depict different digits:
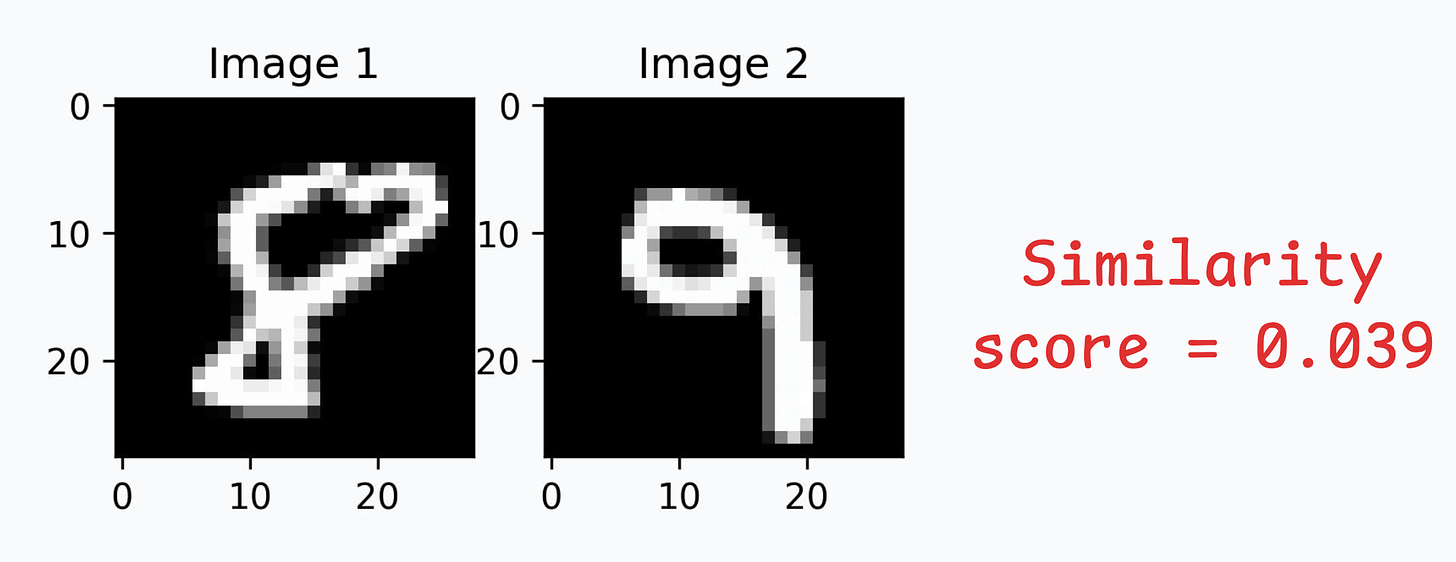
Great, it works as expected!
And with that, we have successfully implemented and verified the predictions of a Siamese Network.
That said, there’s one thing to note.
Since during the data preparation step, we paired the instance either with the same or a different class…
…this inherently meant that this approach demands labeled data.
There are several techniques to get around this, which we shall discuss soon.
👉 In the meantime, it’s over to you: Can you tell how you would handle unlabeled data in this case?
The code for today’s issue is available here: Siamese Network Implementation.
ROADMAP
From local ML to production ML​​​
Once a model has been trained, we move to productionizing and deploying it.
If ideas related to production and deployment intimidate you, here’s a quick roadmap for you to upskill (assuming you know how to train a model):
- First, you would have to compress the model and productionize it. Read these guides:
- Reduce their size with ​Model Compression techniques​.
- ​Supercharge ​​PyTorch Models​​ With TorchScript.​
- If you use sklearn, learn how to ​optimize them with tensor operations​.
- Next, you move to deployment. ​Here’s a beginner-friendly hands-on guide​ that teaches you how to deploy a model, manage dependencies, set up model registry, etc.
- Although you would have tested the model locally, it is still wise to test it in production. There are risk-free (or low-risk) methods to do that. ​Learn what they are and how to implement them here​.
This roadmap should set you up pretty well, even if you have NEVER deployed a single model before since everything is practical and implementation-driven.
THAT'S A WRAP
No-Fluff Industry ML resources to
Succeed in DS/ML roles
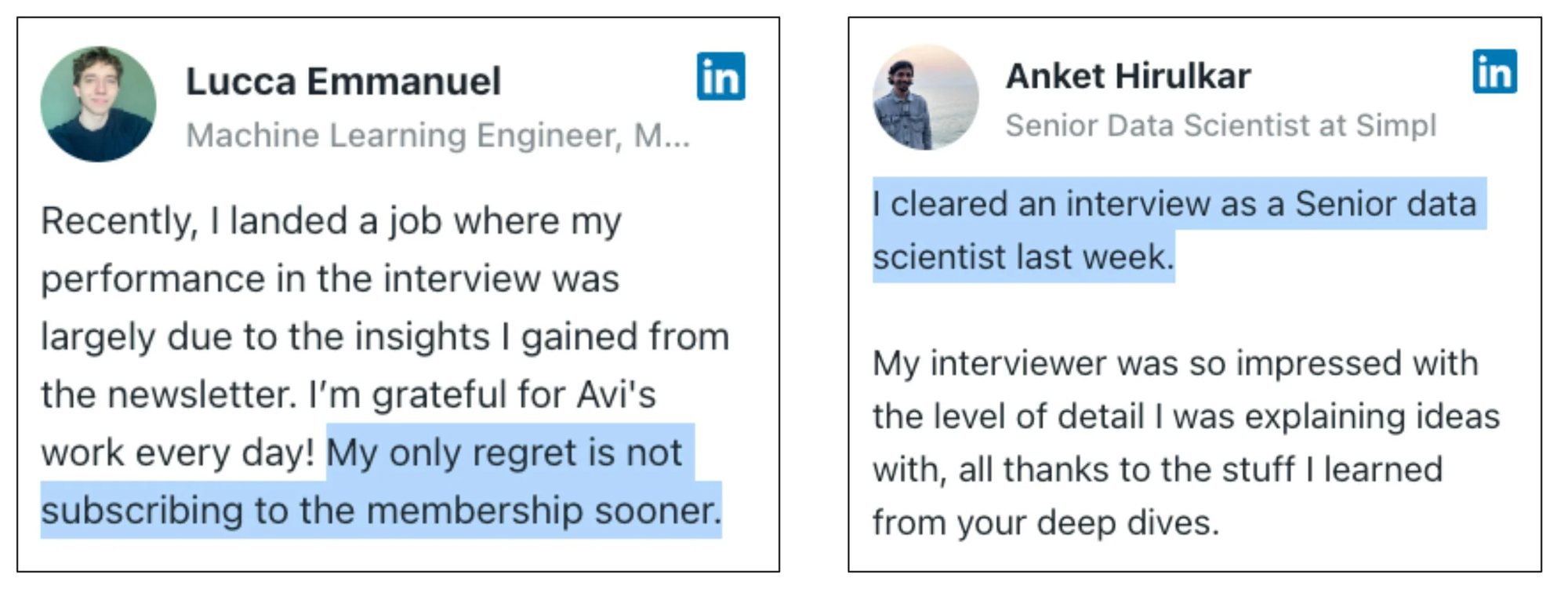
At the end of the day, all businesses care about impact. That’s it!
- Can you reduce costs?
- Drive revenue?
- Can you scale ML models?
- Predict trends before they happen?
We have discussed several other topics (with implementations) in the past that align with such topics.
Here are some of them:
- Learn sophisticated graph architectures and how to train them on graph data in this crash course.
- So many real-world NLP systems rely on pairwise context scoring. Learn scalable approaches here.
- Run large models on small devices using Quantization techniques.
- Learn how to generate prediction intervals or sets with strong statistical guarantees for increasing trust using Conformal Predictions.
- Learn how to identify causal relationships and answer business questions using causal inference in this crash course.
- Learn how to scale and implement ML model training in this practical guide.
- Learn 5 techniques with implementation to reliably test ML models in production.
- Learn how to build and implement privacy-first ML systems using Federated Learning.
- Learn 6 techniques with implementation to compress ML models.
All these resources will help you cultivate key skills that businesses and companies care about the most.
SPONSOR US
Advertise to 600k+ data professionals
Our newsletter puts your products and services directly in front of an audience that matters — thousands of leaders, senior data scientists, machine learning engineers, data analysts, etc., around the world.