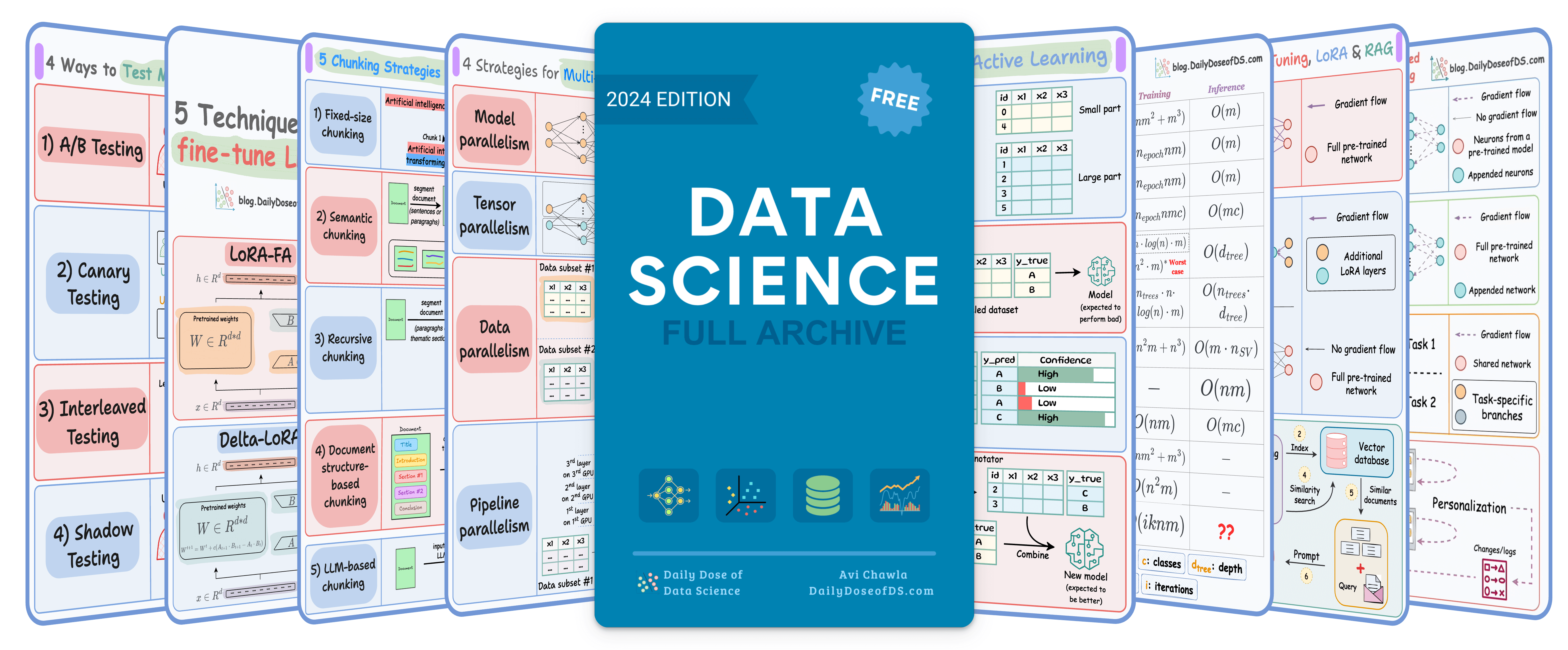
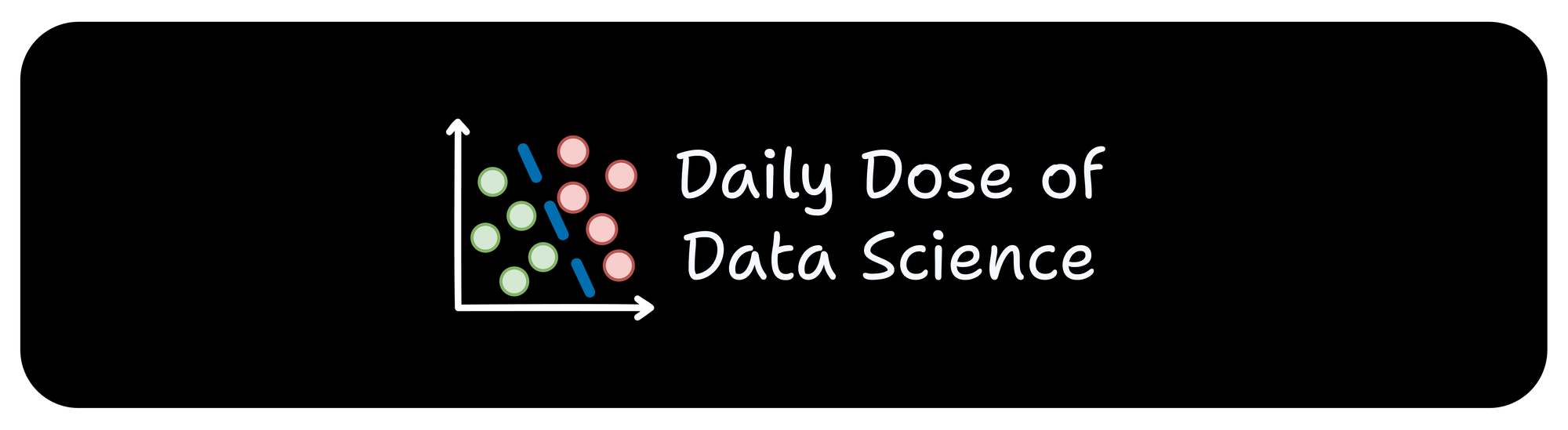
TODAY'S ISSUE
TODAY’S DAILY DOSE OF DATA SCIENCE
5 Powerful MCP Server
Integrating a tool/API with Agents demands:
- reading docs
- writing code
- updating the code, etc.
To simplify this, platforms now offer MCP servers. Developers can plug them with Agents and use their APIs instantly. We also covered it in a recent newsletter issue (read here).
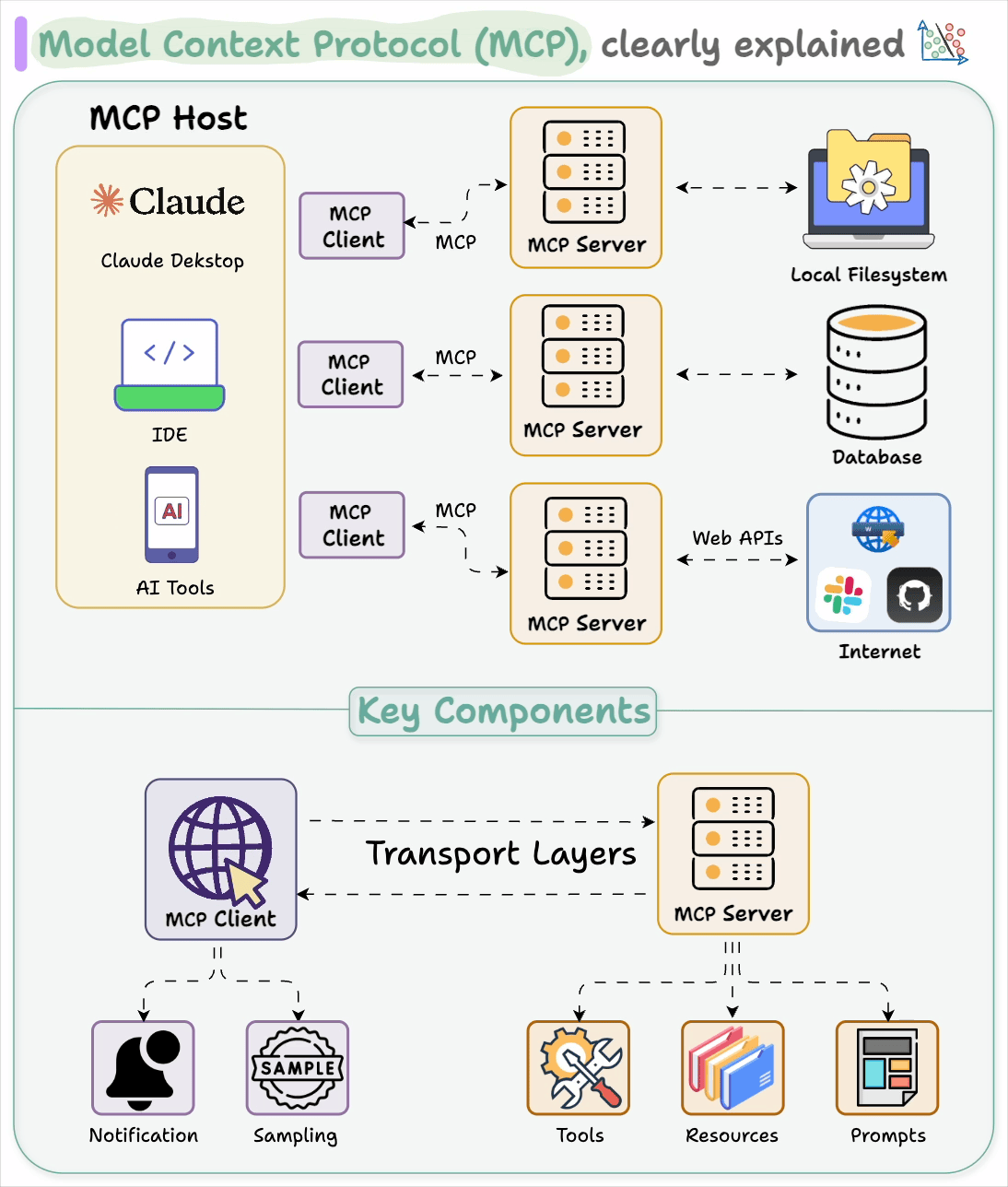
Below, let's look at 5 incredibly powerful MCP servers.
#1) Firecrawl MCP server
This adds powerful web scraping capabilities to Cursor, Claude, and any other LLM clients using Firecrawl.
Tools include:
- Scraping
- Crawling
- Deep research
- Extracting structured data
- and more
Here’s a demo:
#2) Browserbase MCP server
This allows Agents to initiate a browser session with Browserbase.
Tools include:
- Create browser session
- Navigate to a URL
- Take screenshot
- and more
Here’s a demo:
#3) Opik MCP server
This enables traceability into AI Agents and lets you monitor your LLM applications, by Comet.
Tools include:
- Creating projects
- Enable tracing
- Getting tracing stats
- and more
Here’s a demo:
#4) Brave MCP server
This enables Agents to use the Brave Search API for both web and local search capabilities.
Tools include:
- Brave web search
- Brave local search
#5) Sequential thinking
This enables dynamic and reflective problem-solving through a structured thinking process.
Which ones are your favorite MCP servers? Let us know!
IN CASE YOU MISSED IT
​​KV caching in LLMs, explained visually​
KV caching is a popular technique to speed up LLM inference.
To get some perspective, look at the inference speed difference from our demo:
- with KV caching → 9 seconds
- without KV caching → 40 seconds (~4.5x slower, and this gap grows as more tokens are produced).
The visual explains how it works:
ROADMAP
​16 techniques to build real-world RAG systems​
On paper, implementing a RAG system seems simple—connect a vector database, process documents, embed the data, embed the query, query the vector database, and prompt the LLM.
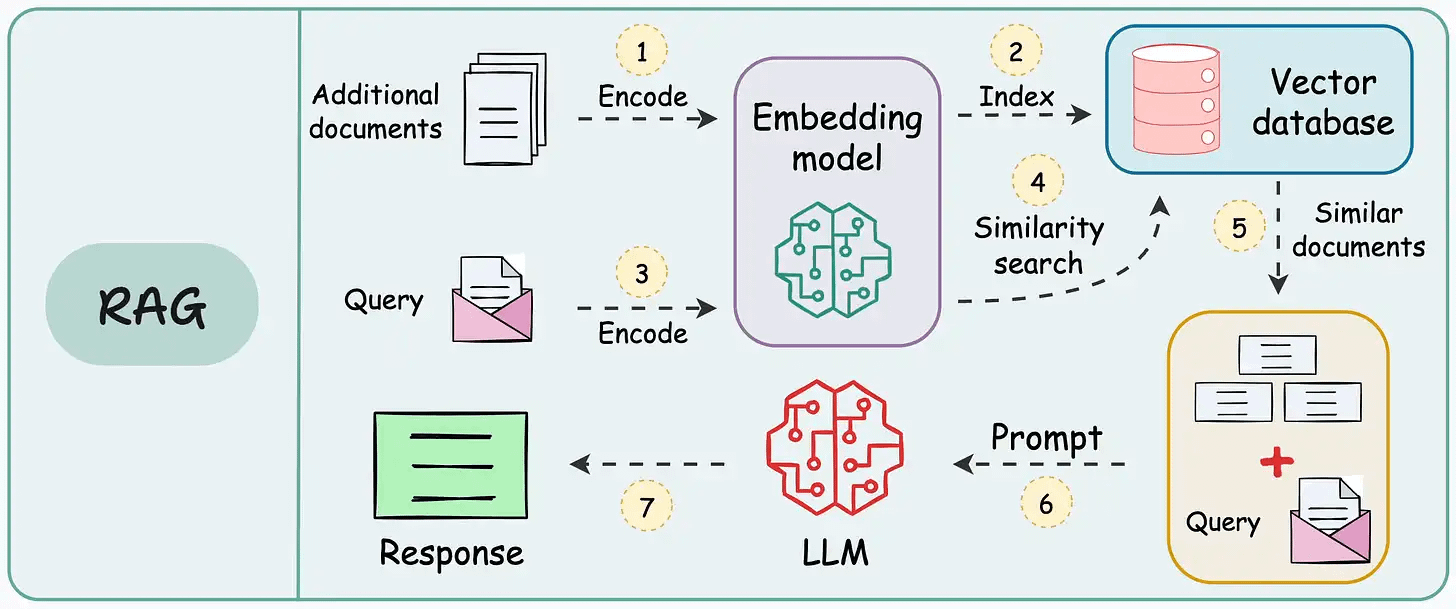
But in practice, turning a prototype into a high-performance application is an entirely different challenge.
We published a two-part guide that covers 16 practical techniques to build real-world RAG systems:
THAT'S A WRAP
No-Fluff Industry ML resources to
Succeed in DS/ML roles
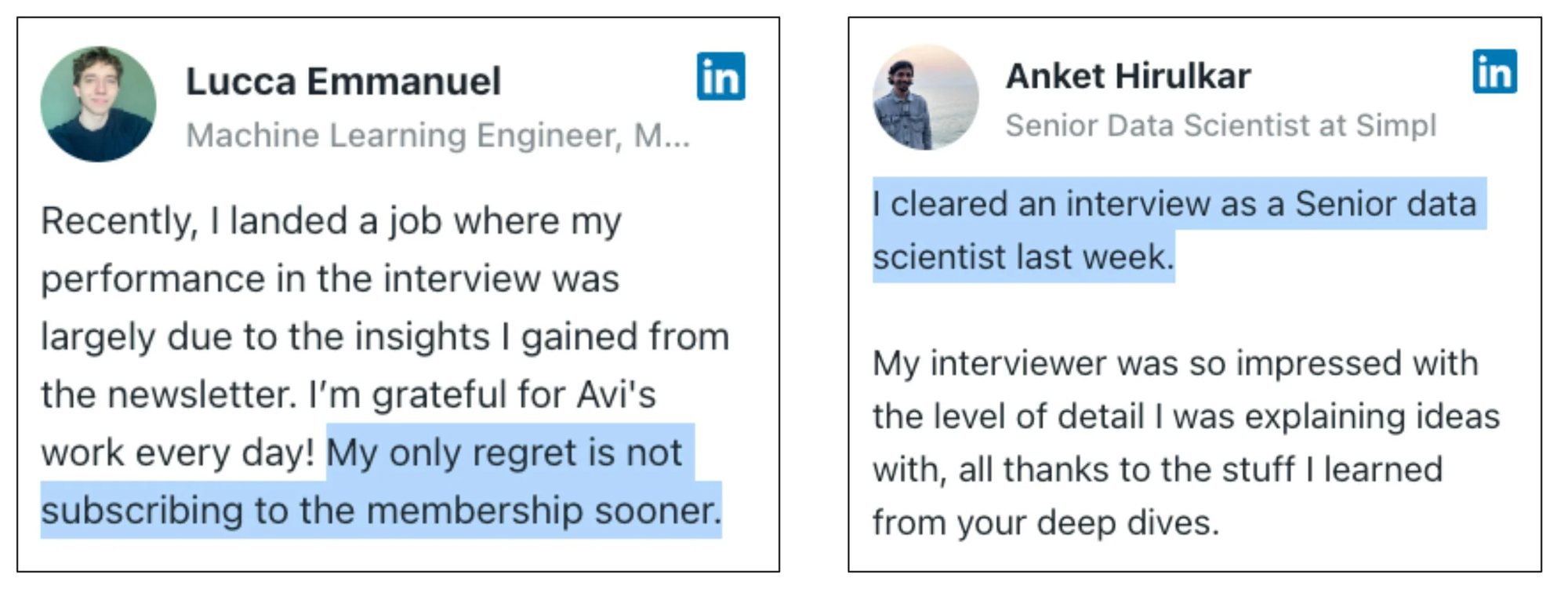
At the end of the day, all businesses care about impact. That’s it!
- Can you reduce costs?
- Drive revenue?
- Can you scale ML models?
- Predict trends before they happen?
We have discussed several other topics (with implementations) in the past that align with such topics.
Here are some of them:
- Learn sophisticated graph architectures and how to train them on graph data in this crash course.
- So many real-world NLP systems rely on pairwise context scoring. Learn scalable approaches here.
- Run large models on small devices using Quantization techniques.
- Learn how to generate prediction intervals or sets with strong statistical guarantees for increasing trust using Conformal Predictions.
- Learn how to identify causal relationships and answer business questions using causal inference in this crash course.
- Learn how to scale and implement ML model training in this practical guide.
- Learn 5 techniques with implementation to reliably test ML models in production.
- Learn how to build and implement privacy-first ML systems using Federated Learning.
- Learn 6 techniques with implementation to compress ML models.
All these resources will help you cultivate key skills that businesses and companies care about the most.
SPONSOR US
Advertise to 600k+ data professionals
Our newsletter puts your products and services directly in front of an audience that matters — thousands of leaders, senior data scientists, machine learning engineers, data analysts, etc., around the world.