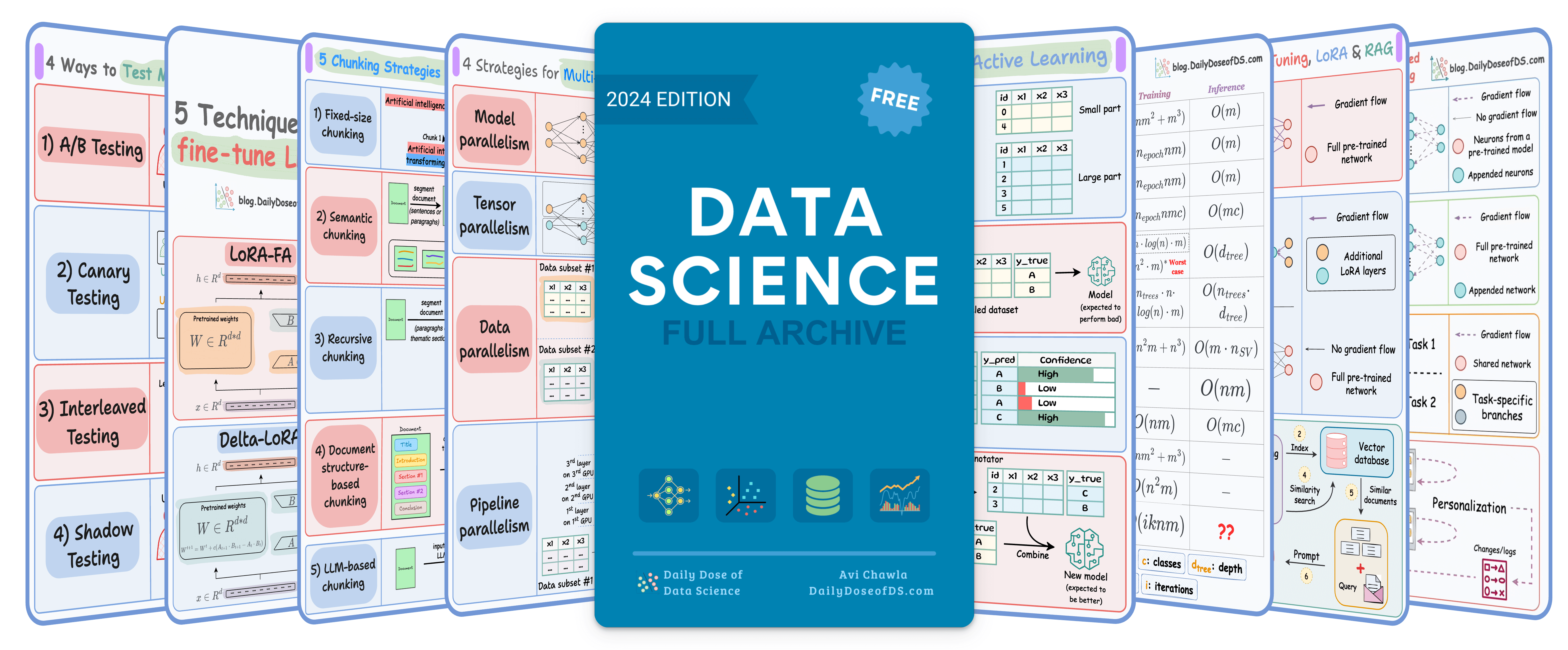
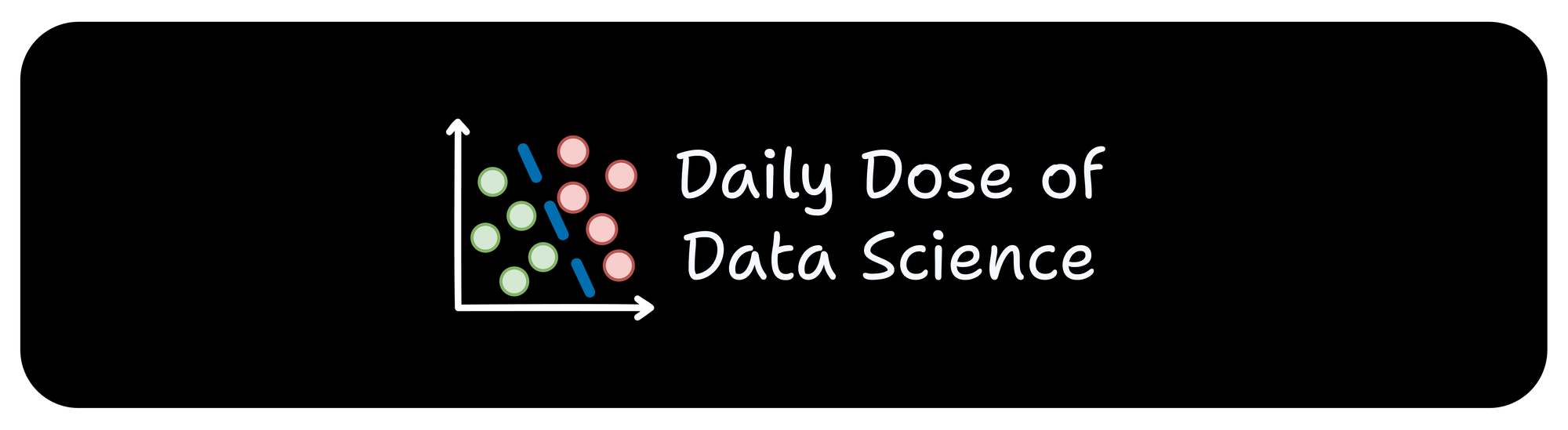
TODAY'S ISSUE
TODAY’S DAILY DOSE OF DATA SCIENCE
Visual Guide to Model Context Protocol (MCP)
Lately, there has been a lot of buzz around Model Context Protocol (MCP). You must have heard about it.
Today, let’s understand what it is.
Intuitively speaking, MCP is like a USB-C port for your AI applications.
Just as USB-C offers a standardized way to connect devices to various accessories, MCP standardizes how your AI apps connect to different data sources and tools.
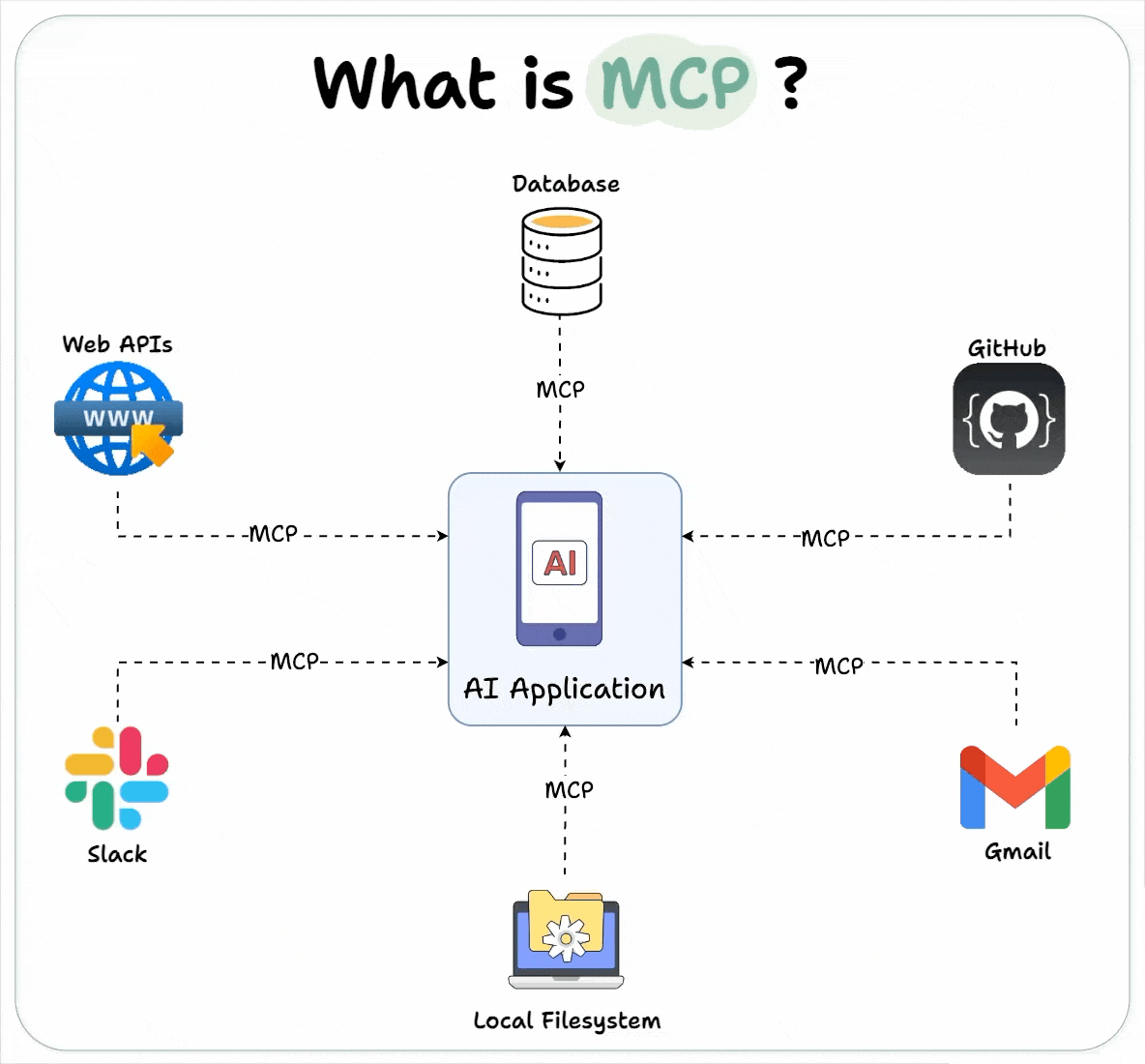
Let’s dive in a bit more technically.
At its core, MCP follows a client-server architecture where a host application can connect to multiple servers.
It has three key components
- Host
- Client
- Server
Here’s an overview before we dig deep👇
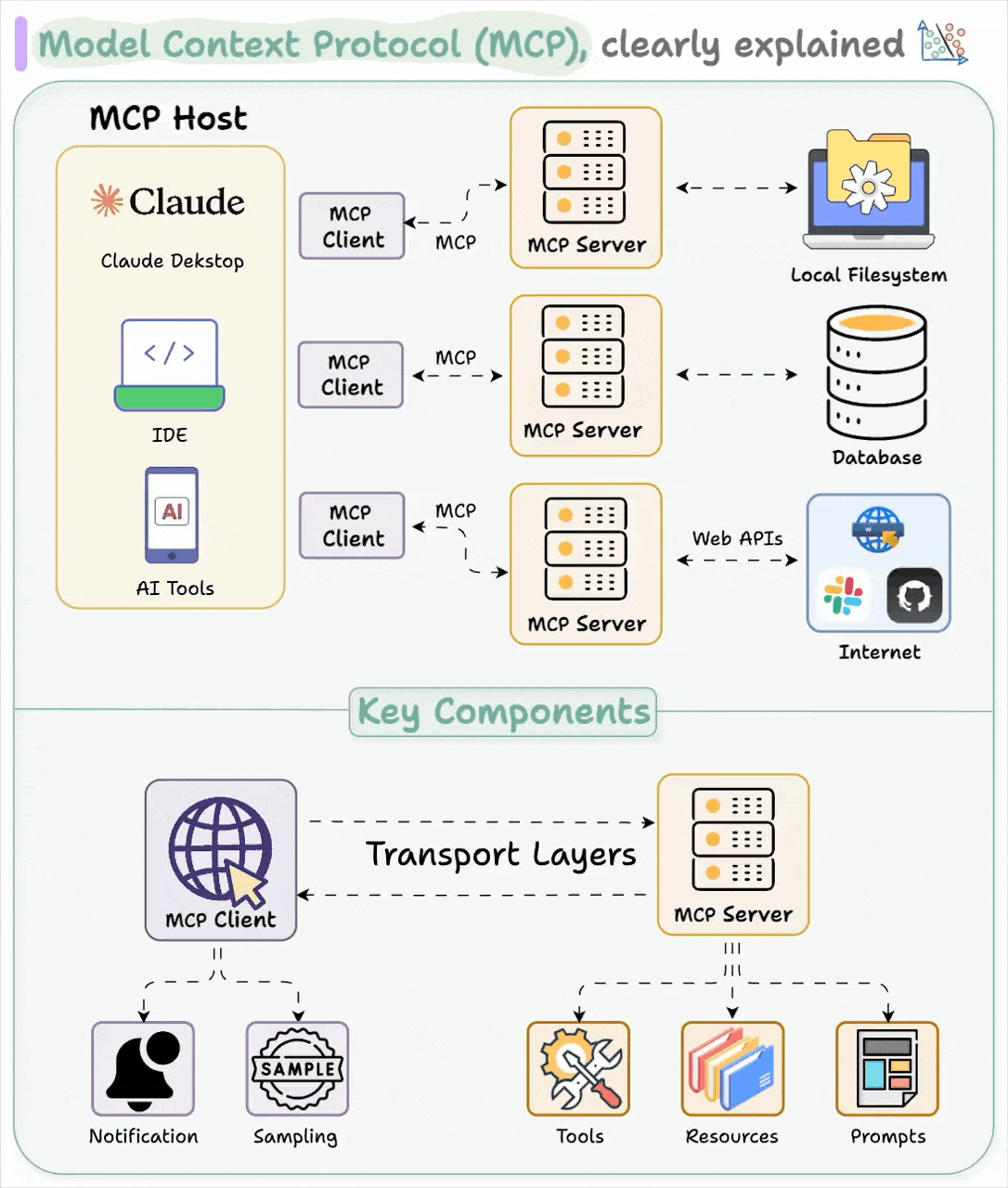
Host represents any AI app (Claude desktop, Cursor) that provides an environment for AI interactions, accesses tools and data, and runs the MCP Client.
MCP Client operates within the host to enable communication with MCP servers.
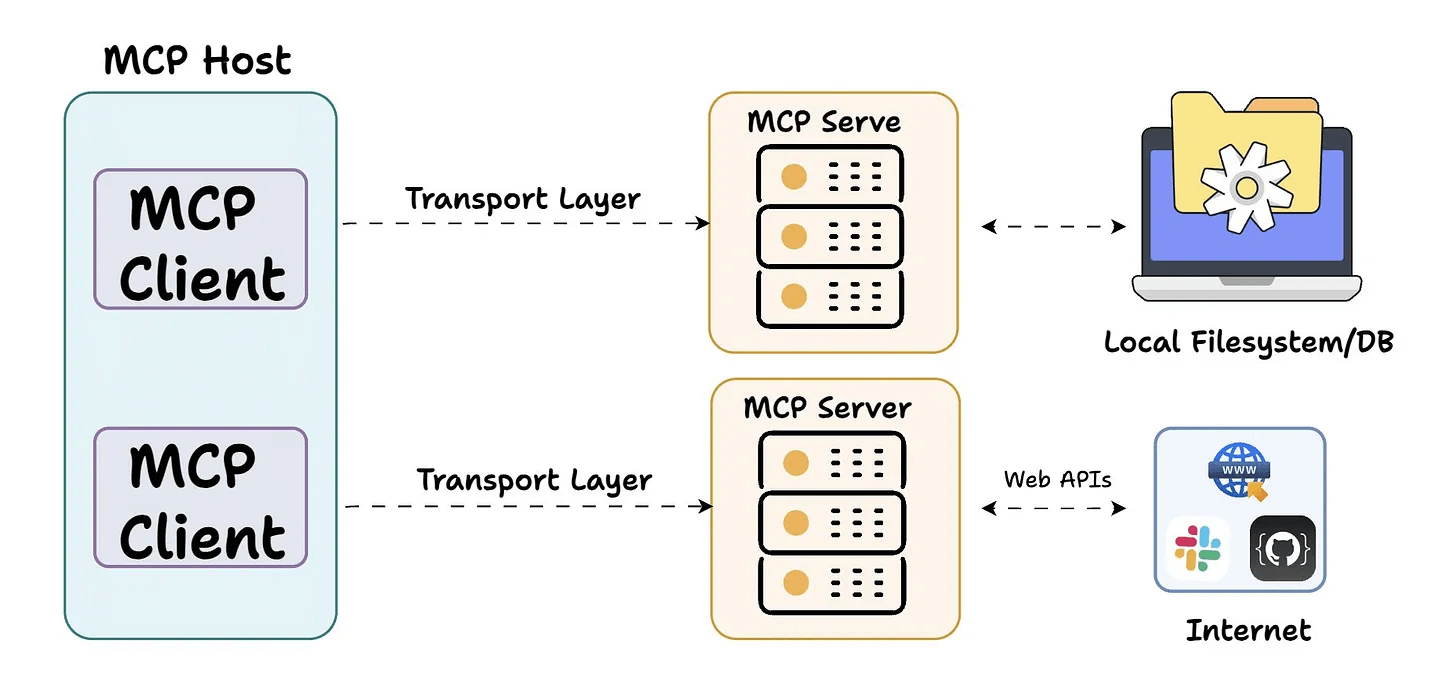
Finally, the MCP Server exposes specific capabilities and provides access to data like:
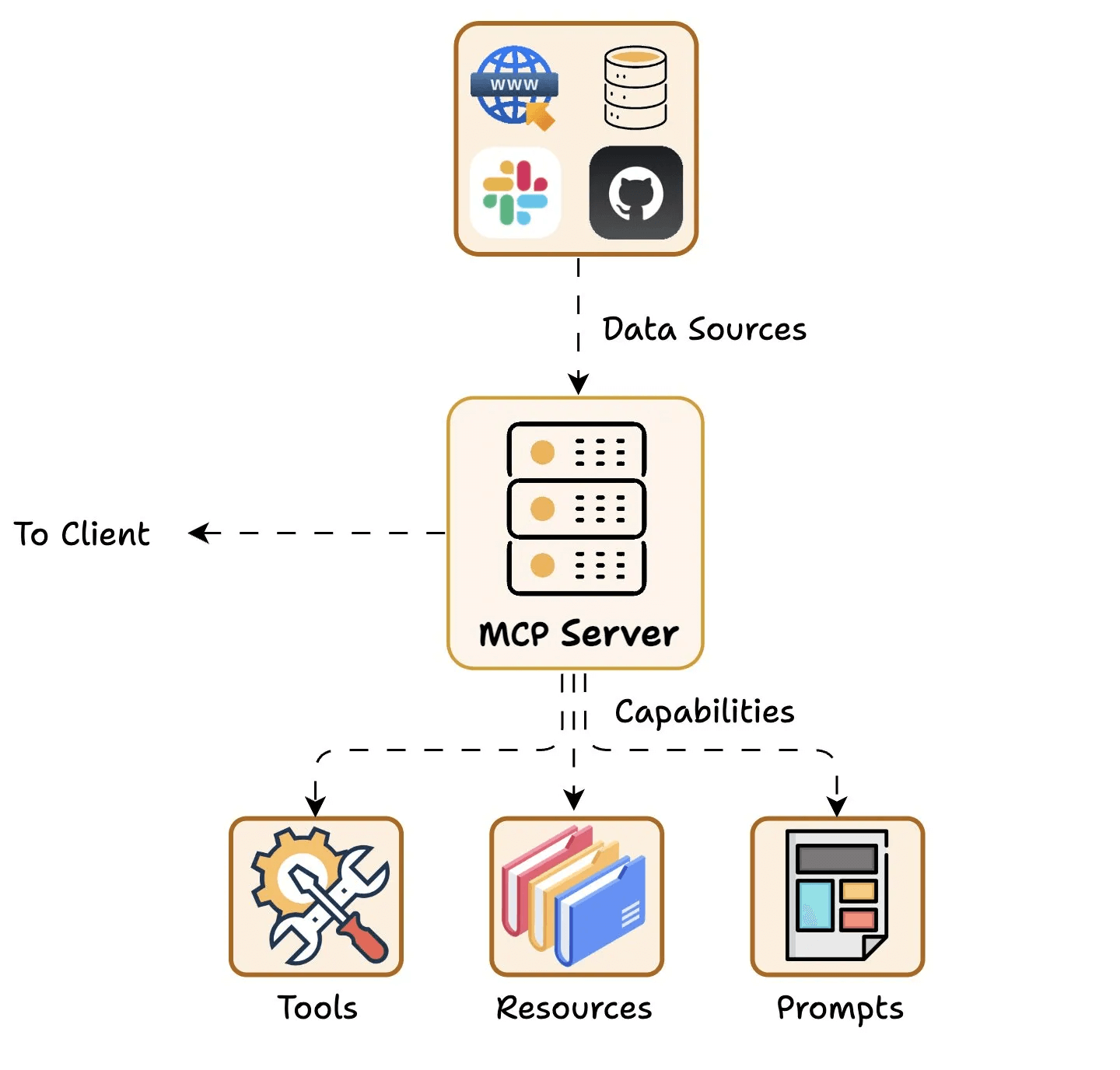
- Tools: Enable LLMs to perform actions through your server.
- Resources: Expose data and content from your servers to LLMs.
- Prompts: Create reusable prompt templates and workflows.
Understanding client-server communication is essential for building your own MCP client-server.
So let’s understand how the client and the server communicate.
Here’s an illustration before we break it down step by step...
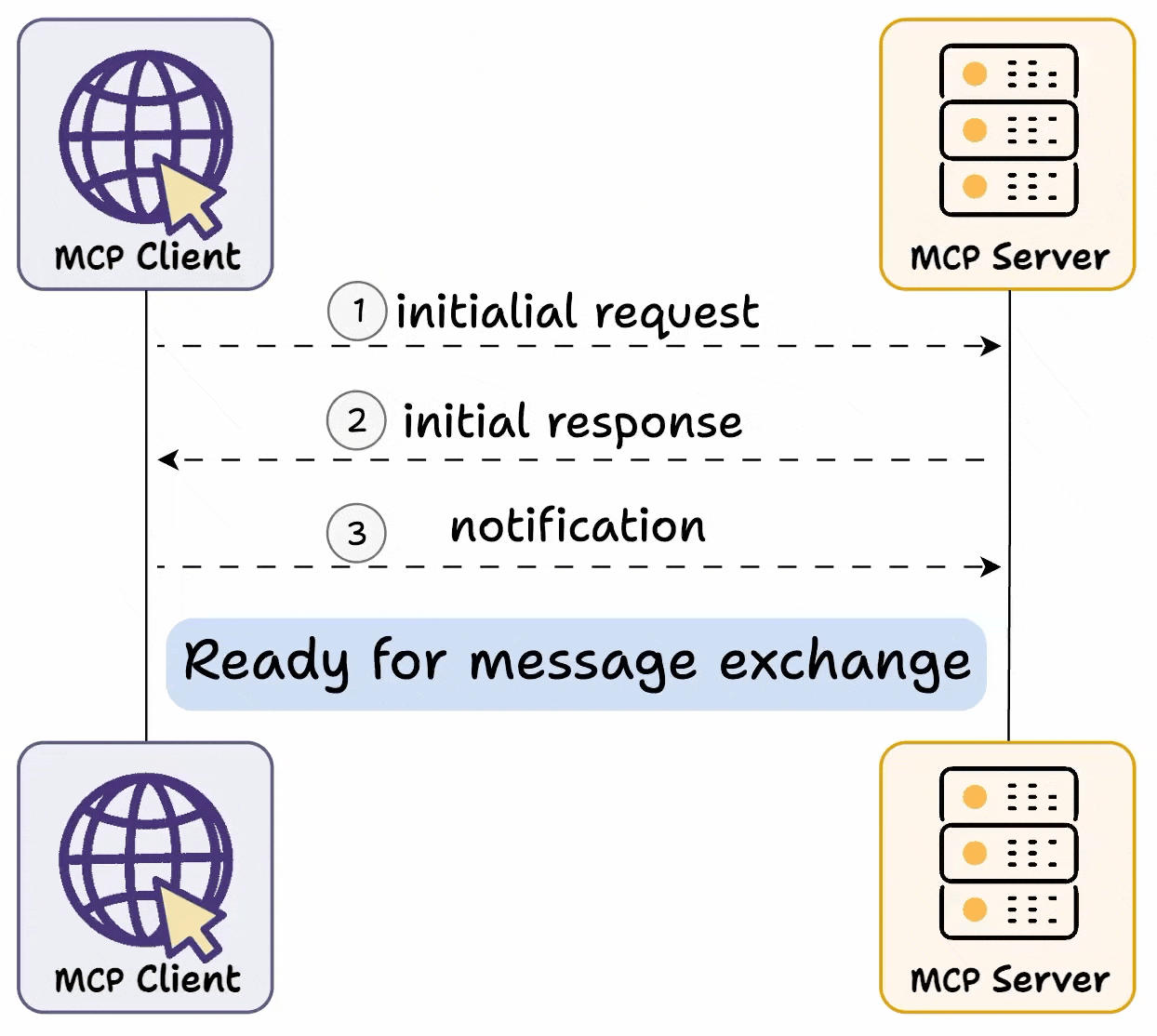
First, we have capability exchange, where:
- The client sends an initial request to learn server capabilities.
- The server then responds with its capability details.
- For instance, a Weather API server, when invoked, can reply back with available “tools”, “prompts templates”, and any other resources for the client to use.
Once this exchange is done, Client acknowledges the successful connection and further message exchange continues.
Here’s one of the reasons this setup can be so powerful:
In a traditional API setup:
- If your API initially requires two parameters (e.g.,
location
anddate
for a weather service), users integrate their applications to send requests with those exact parameters.
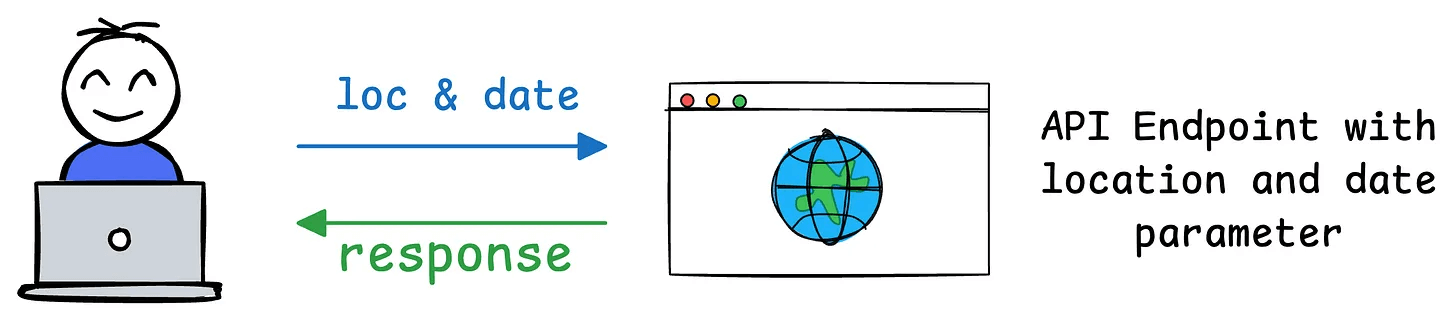
- Later, if you decide to add a third required parameter (e.g.,
unit
for temperature units like Celsius or Fahrenheit), the API’s contract changes.
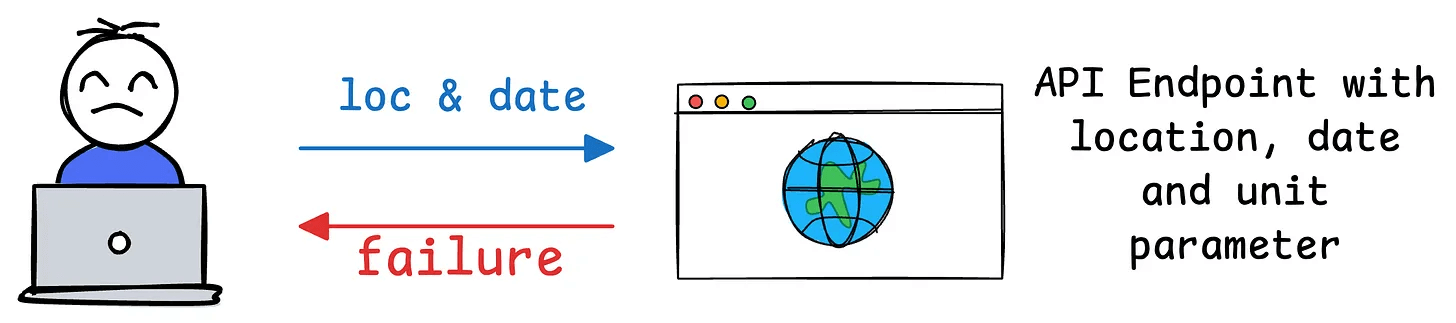
- This means all users of your API must update their code to include the new parameter. If they don’t update, their requests might fail, return errors, or provide incomplete results.
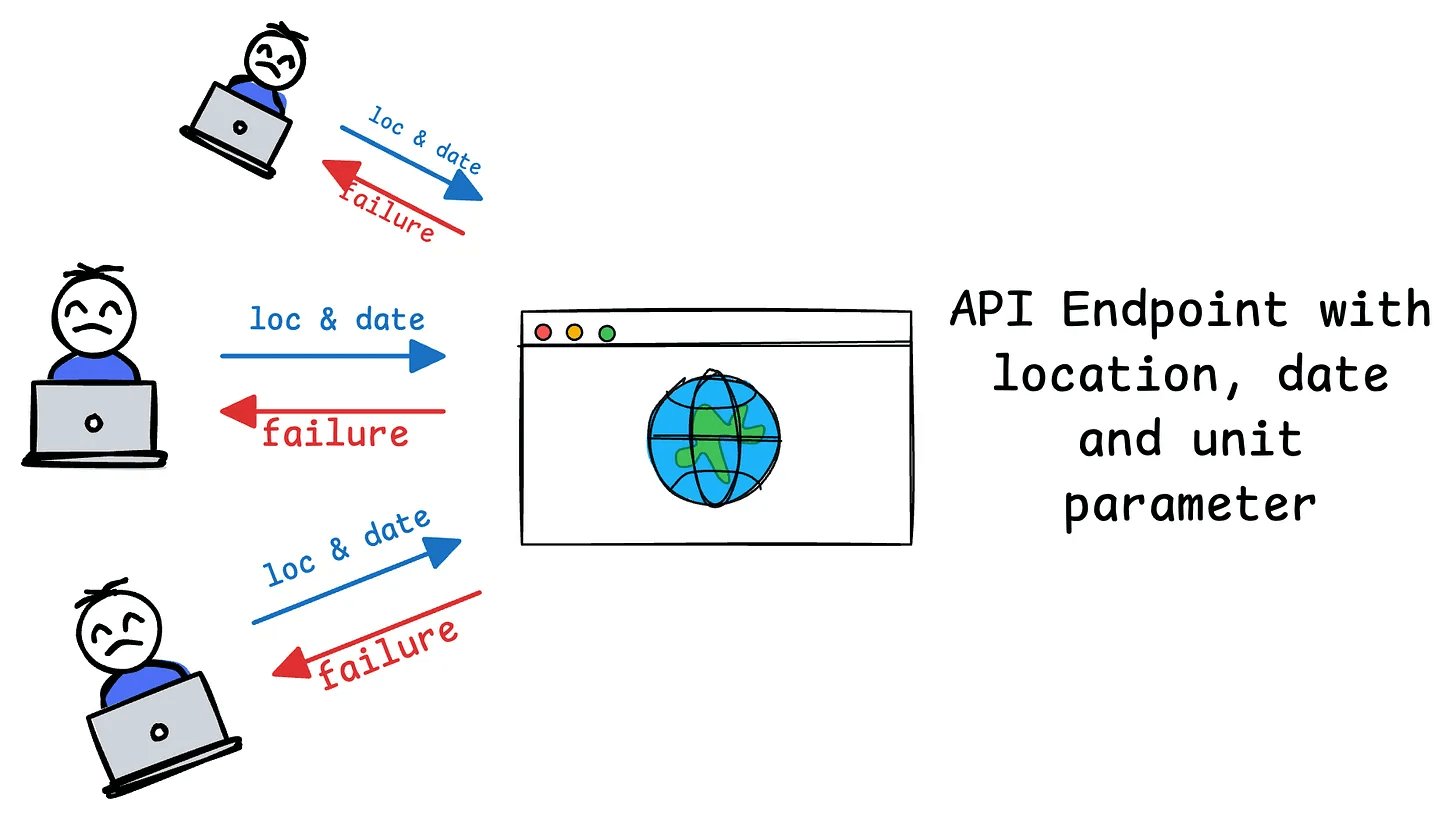
MCP’s design solves this as follows:
- MCP introduces a dynamic and flexible approach that contrasts sharply with traditional APIs.
- For instance, when a client (e.g., an AI application like Claude Desktop) connects to an MCP server (e.g., your weather service), it sends an initial request to learn the server’s capabilities.
- The server responds with details about its available tools, resources, prompts, and parameters. For example, if your weather API initially supports
location
anddate
, the server communicates these as part of its capabilities.
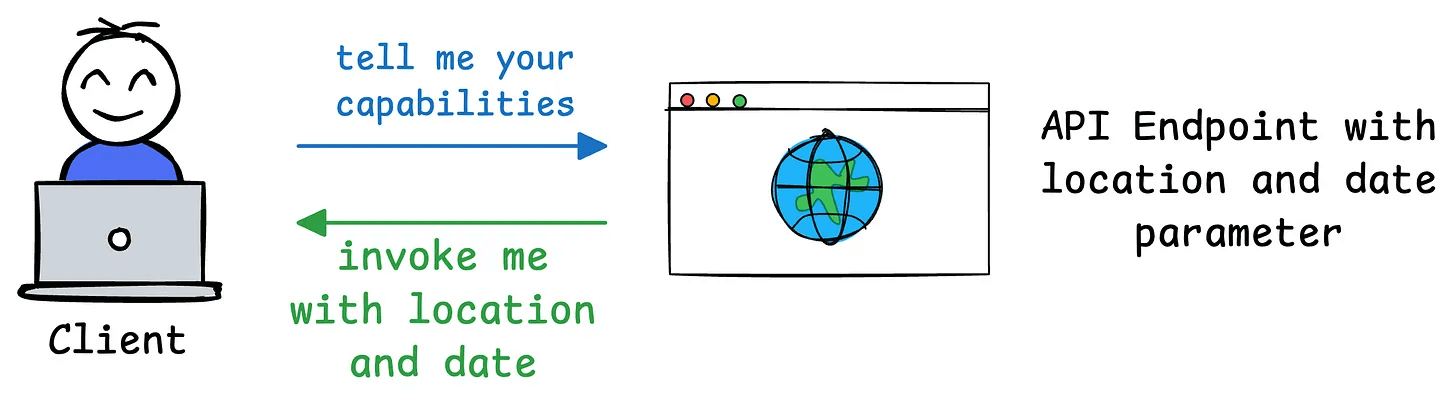
- If you later add a
unit
parameter, the MCP server can dynamically update its capability description during the next exchange. The client doesn’t need to hardcode or predefine the parameters—it simply queries the server’s current capabilities and adapts accordingly.
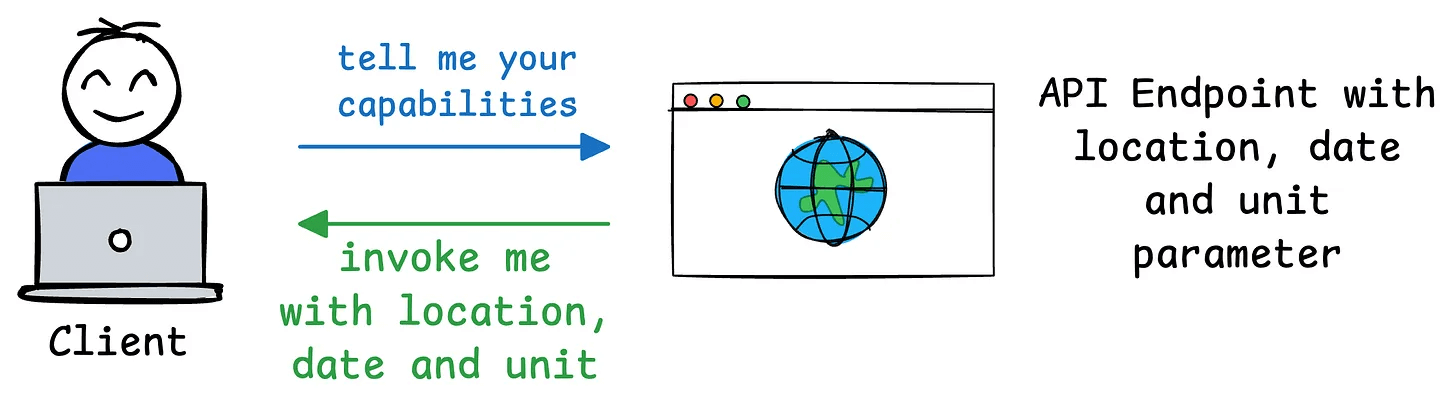
- This way, the client can then adjust its behavior on-the-fly, using the updated capabilities (e.g., including
unit
in its requests) without needing to rewrite or redeploy code.
We hope this clarifies what MCP does.
In the future, we shall explore creating custom MCP servers and building hands-on demos around them. Stay tuned!
👉 Over to you: Do you think MCP is more powerful than traditional API setup?
IN CASE YOU MISSED IT
​Prompting vs. RAG vs. Fine-tuning​
If you are building real-world LLM-based apps, it is unlikely you can start using the model right away without adjustments. To maintain high utility, you either need:
- Prompt engineering
- Fine-tuning
- RAG
- Or a hybrid approach (RAG + fine-tuning)
The following visual will help you decide which one is best for you:
​Read more in-depth insights into Prompting vs. RAG vs. Fine-tuning here →
ROADMAP
From local ML to production ML
Once a model has been trained, we move to productionizing and deploying it.
If ideas related to production and deployment intimidate you, here’s a quick roadmap for you to upskill (assuming you know how to train a model):
- First, you would have to compress the model and productionize it. Read these guides:
- Reduce their size with ​Model Compression techniques​.
- ​Supercharge ​​PyTorch Models​​ With TorchScript.​
- If you use sklearn, learn how to ​optimize them with tensor operations​.
- Next, you move to deployment. ​Here’s a beginner-friendly hands-on guide​ that teaches you how to deploy a model, manage dependencies, set up model registry, etc.
- Although you would have tested the model locally, it is still wise to test it in production. There are risk-free (or low-risk) methods to do that. ​Learn what they are and how to implement them here​.
This roadmap should set you up pretty well, even if you have NEVER deployed a single model before since everything is practical and implementation-driven.
THAT'S A WRAP
No-Fluff Industry ML resources to
Succeed in DS/ML roles
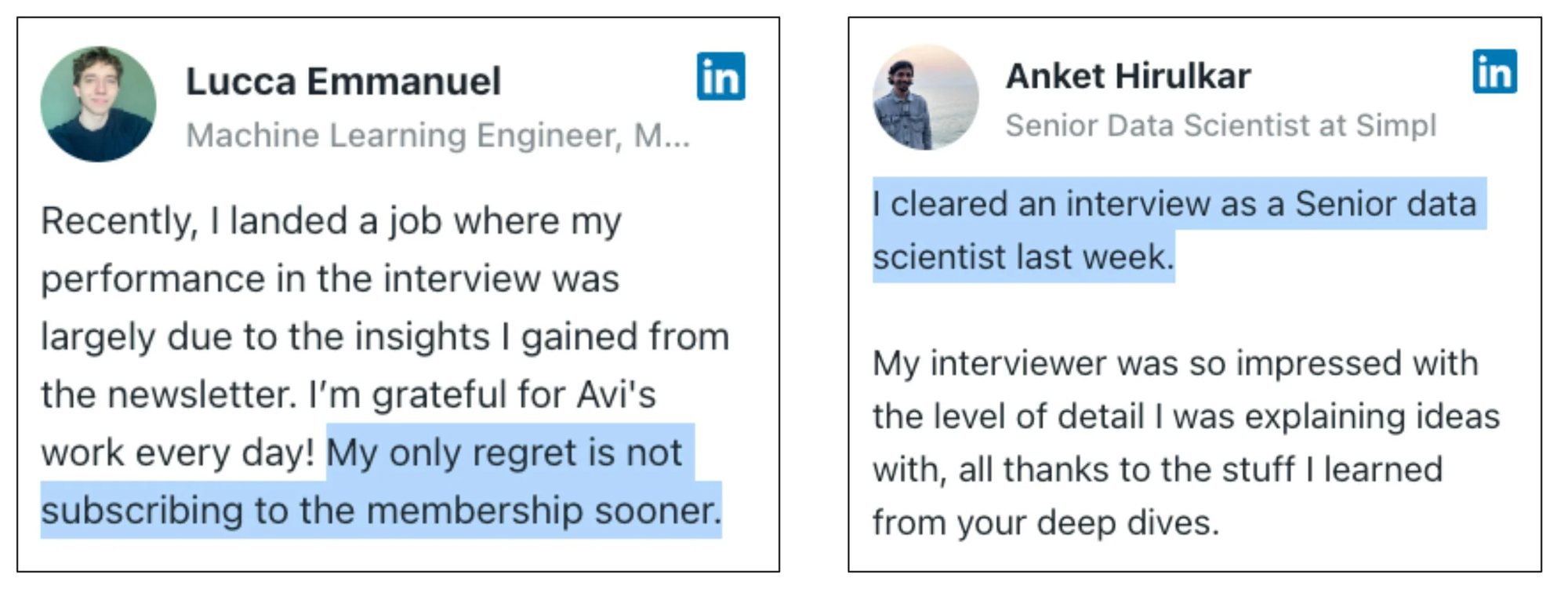
At the end of the day, all businesses care about impact. That’s it!
- Can you reduce costs?
- Drive revenue?
- Can you scale ML models?
- Predict trends before they happen?
We have discussed several other topics (with implementations) in the past that align with such topics.
Here are some of them:
- Learn sophisticated graph architectures and how to train them on graph data in this crash course.
- So many real-world NLP systems rely on pairwise context scoring. Learn scalable approaches here.
- Run large models on small devices using Quantization techniques.
- Learn how to generate prediction intervals or sets with strong statistical guarantees for increasing trust using Conformal Predictions.
- Learn how to identify causal relationships and answer business questions using causal inference in this crash course.
- Learn how to scale and implement ML model training in this practical guide.
- Learn 5 techniques with implementation to reliably test ML models in production.
- Learn how to build and implement privacy-first ML systems using Federated Learning.
- Learn 6 techniques with implementation to compress ML models.
All these resources will help you cultivate key skills that businesses and companies care about the most.
SPONSOR US
Advertise to 600k+ data professionals
Our newsletter puts your products and services directly in front of an audience that matters — thousands of leaders, senior data scientists, machine learning engineers, data analysts, etc., around the world.