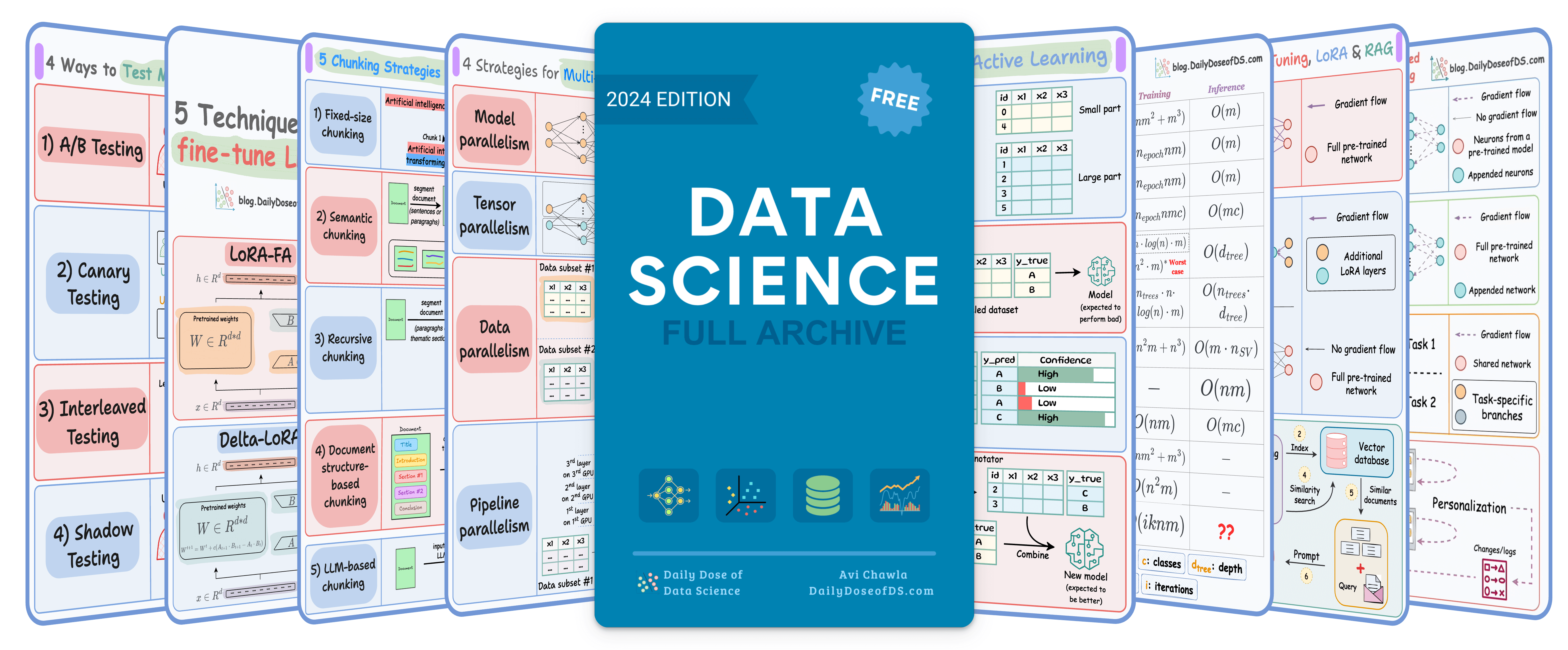
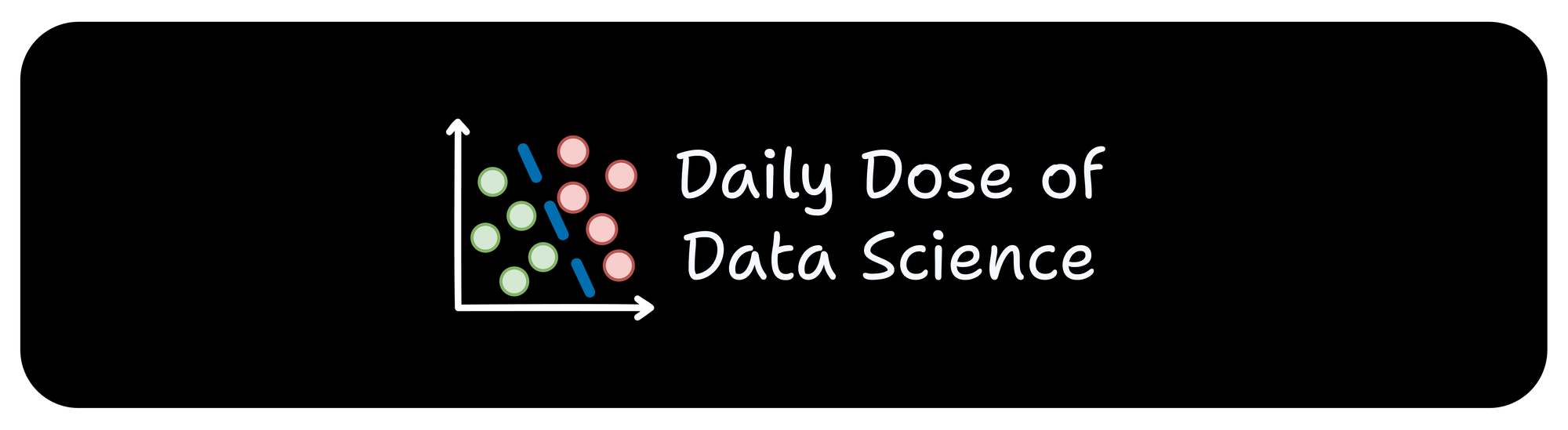
TODAY'S ISSUE
TODAY’S DAILY DOSE OF DATA SCIENCE
​Shuffle Feature Importance
There are so many techniques to measure feature importance.
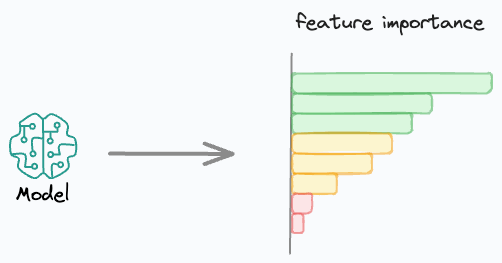
I often find “Shuffle Feature Importance” to be a handy and intuitive technique to measure feature importance.
Let’s understand this today!
As the name suggests, it observes how shuffling a feature influences the model performance.
The visual below illustrates this technique in four simple steps:
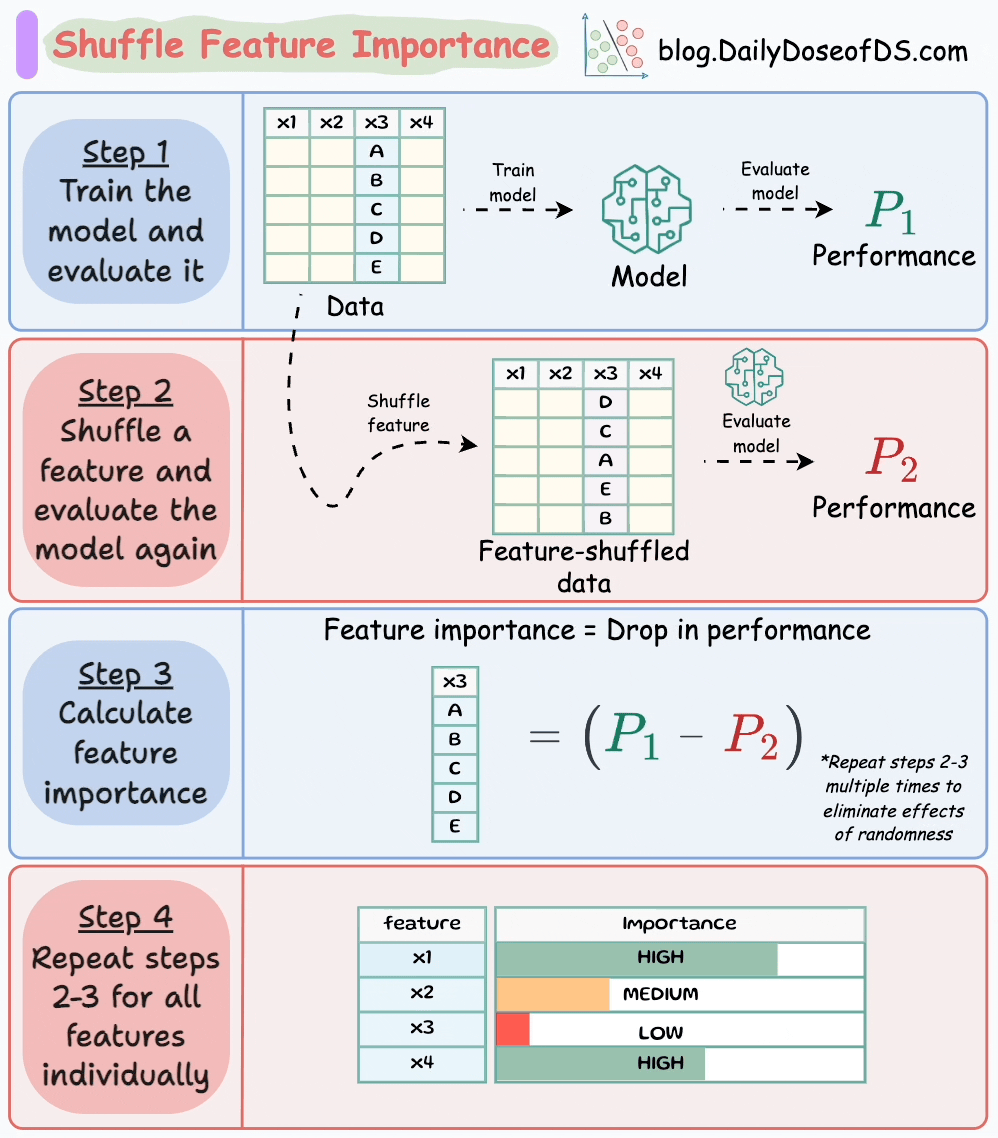
Here’s how it works:
- Train the model and measure its performance →
P1
. - Shuffle one feature randomly and measure performance again →
P2
(model is NOT trained again). - Measure feature importance using performance drop = (
P1-P2
). - Repeat for all features.
This makes intuitive sense as well, doesn’t it?
Simply put, if we randomly shuffle just one feature and everything else stays the same, then the performance drop will indicate how important that feature is.
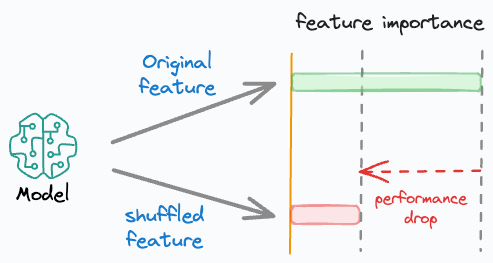
- If the performance drop is low → This means the feature has a very low influence on the model’s predictions.
- If the performance drop is high → This means that the feature has a very high influence on the model’s predictions.
Do note that to eliminate any potential effects of randomness during feature shuffling, it is recommended to:
- Shuffle the same feature multiple times
- Measure average performance drop.
A few things that I love about this technique are:
- It requires no repetitive model training. Just train the model once and measure the feature importance.
- It is pretty simple to use and quite intuitive to interpret.
- This technique can be used for all ML models that can be evaluated.
Of course, there is one caveat as well.
Say two features are highly correlated, and one of them is permuted/shuffled.
In this case, the model will still have access to the feature through its correlated feature.
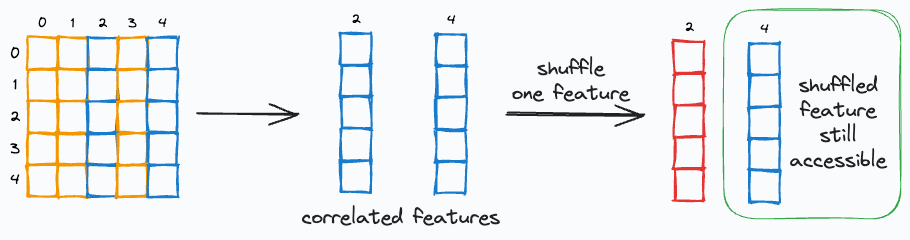
This will result in a lower importance value for both features.
One way to handle this is to cluster highly correlated features and only keep one feature from each cluster.
👉 Over to you: What other reliable feature importance techniques do you use frequently?
TREE-BASED MODEL BUILDING
Formulating and Implementing XGBoost From Scratch​
If you consider the last decade (or 12-13 years) in ML, neural networks have dominated the narrative in most discussions.
In contrast, tree-based methods tend to be perceived as more straightforward, and as a result, they don't always receive the same level of admiration.
However, in practice, tree-based methods frequently outperform neural networks, particularly in structured data tasks.
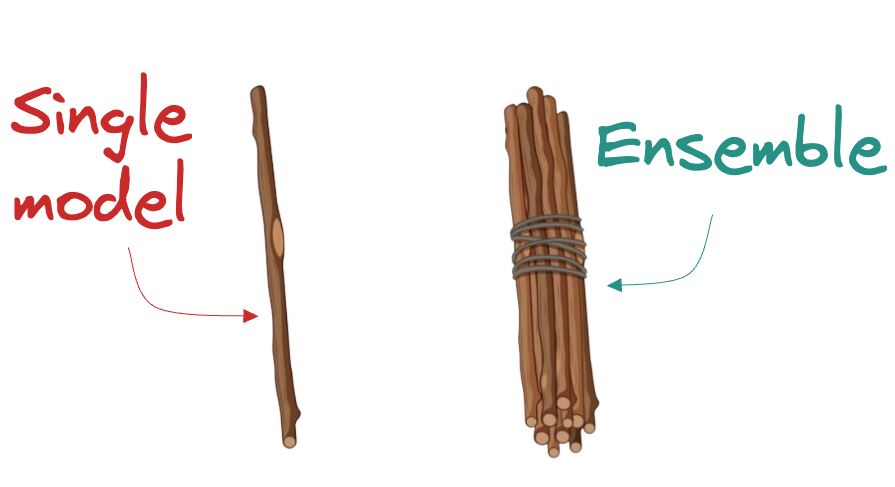
This is a well-known fact among Kaggle competitors, where ​XGBoost​ has become the tool of choice for top-performing submissions.
One would spend a fraction of the time they would otherwise spend on models like linear/logistic regression, SVMs, etc., to achieve the same performance as XGBoost.
​Learn about its internal details by formulating and implementing it from scratch here →
MODEL OPTIMIZATION
Model compression to optimize models for production
Model accuracy alone (or an equivalent performance metric) rarely determines which model will be deployed.
Much of the engineering effort goes into making the model production-friendly.
Because typically, the model that gets shipped is NEVER solely determined by performance — a misconception that many have.
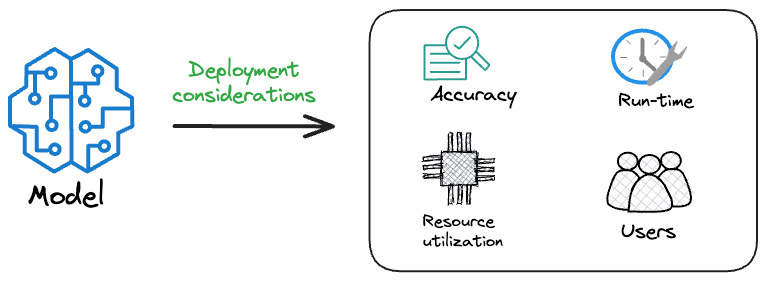
Instead, we also consider several operational and feasibility metrics, such as:
- Inference Latency: Time taken by the model to return a prediction.
- Model size: The memory occupied by the model.
- Ease of scalability, etc.
For instance, consider the image below. It compares the accuracy and size of a large neural network I developed to its pruned (or reduced/compressed) version:
Looking at these results, don’t you strongly prefer deploying the model that is 72% smaller, but is still (almost) as accurate as the large model?
Of course, this depends on the task but in most cases, it might not make any sense to deploy the large model when one of its largely pruned versions performs equally well.
We discussed and implemented 6 model compression techniques in the article ​here​, which ML teams regularly use to save 1000s of dollars in running ML models in production.
​Learn how to compress models before deployment with implementation →
THAT'S A WRAP
No-Fluff Industry ML resources to
Succeed in DS/ML roles
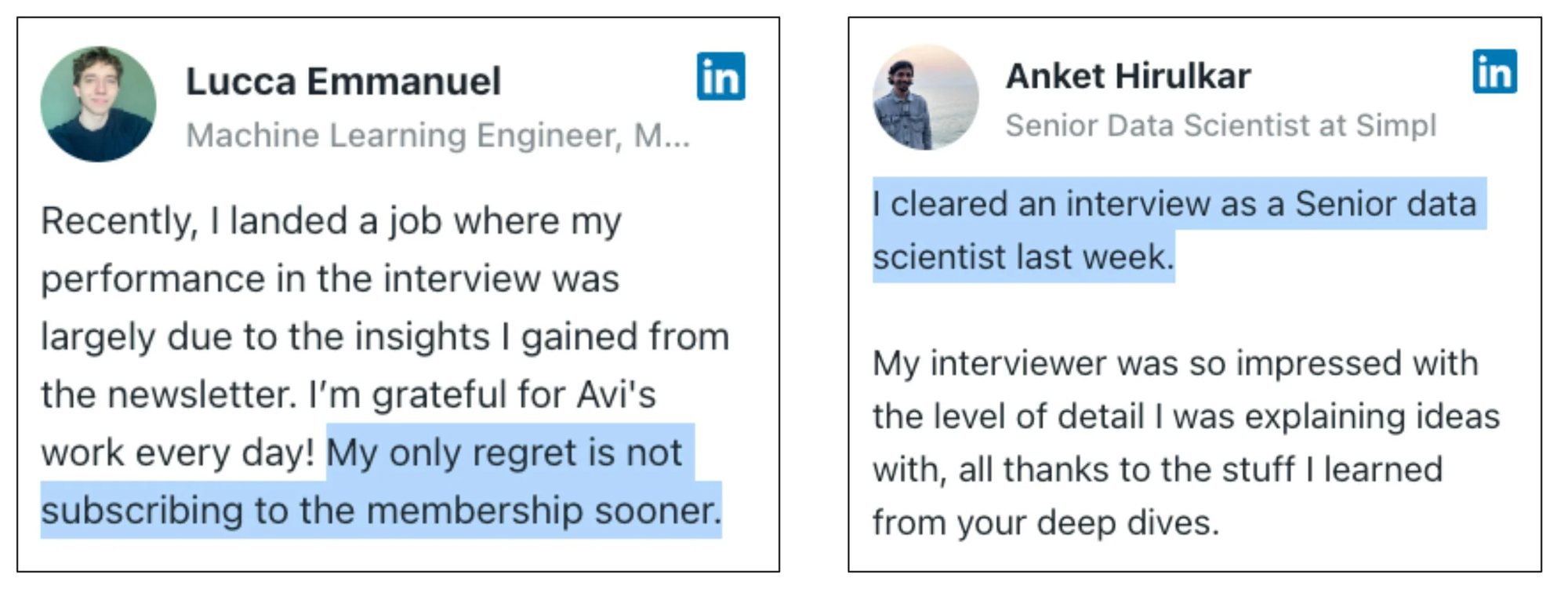
At the end of the day, all businesses care about impact. That’s it!
- Can you reduce costs?
- Drive revenue?
- Can you scale ML models?
- Predict trends before they happen?
We have discussed several other topics (with implementations) in the past that align with such topics.
Here are some of them:
- Learn sophisticated graph architectures and how to train them on graph data in this crash course.
- So many real-world NLP systems rely on pairwise context scoring. Learn scalable approaches here.
- Run large models on small devices using Quantization techniques.
- Learn how to generate prediction intervals or sets with strong statistical guarantees for increasing trust using Conformal Predictions.
- Learn how to identify causal relationships and answer business questions using causal inference in this crash course.
- Learn how to scale and implement ML model training in this practical guide.
- Learn 5 techniques with implementation to reliably test ML models in production.
- Learn how to build and implement privacy-first ML systems using Federated Learning.
- Learn 6 techniques with implementation to compress ML models.
All these resources will help you cultivate key skills that businesses and companies care about the most.
SPONSOR US
Advertise to 600k+ data professionals
Our newsletter puts your products and services directly in front of an audience that matters — thousands of leaders, senior data scientists, machine learning engineers, data analysts, etc., around the world.