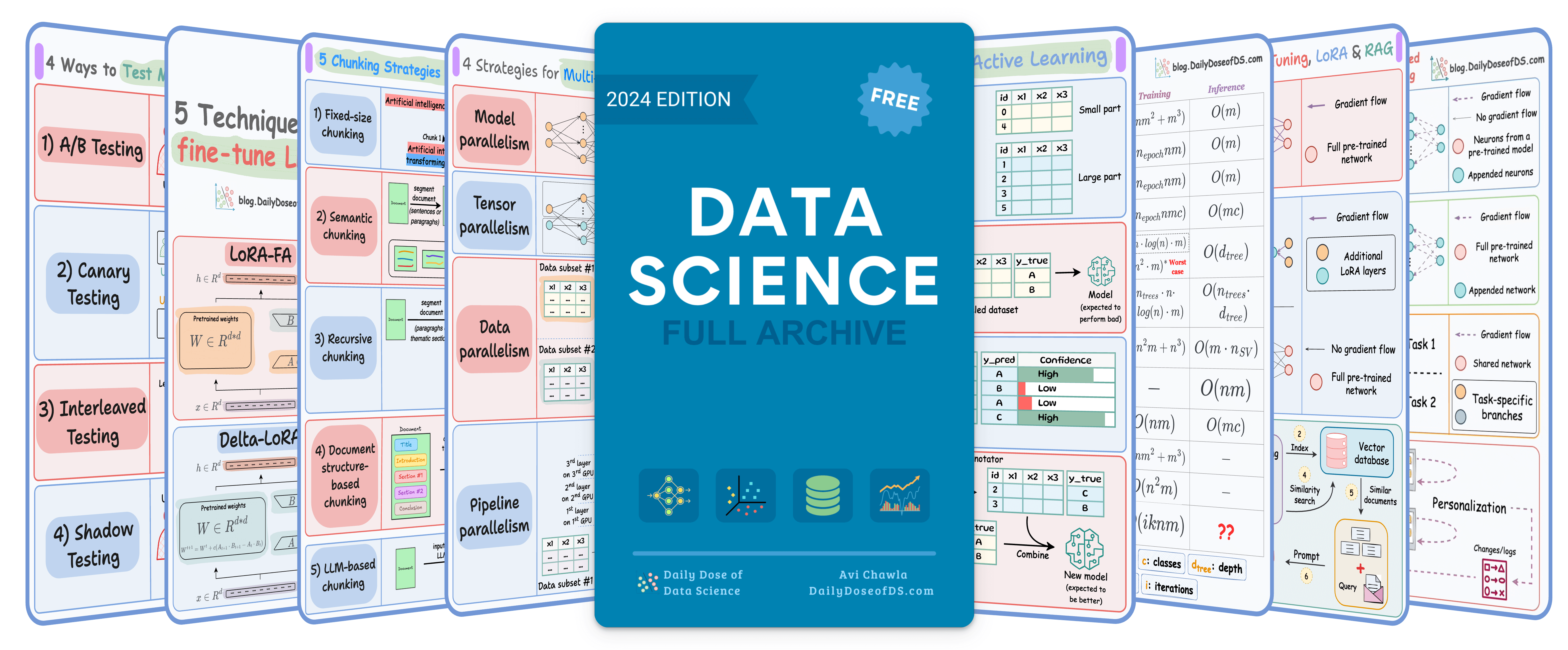
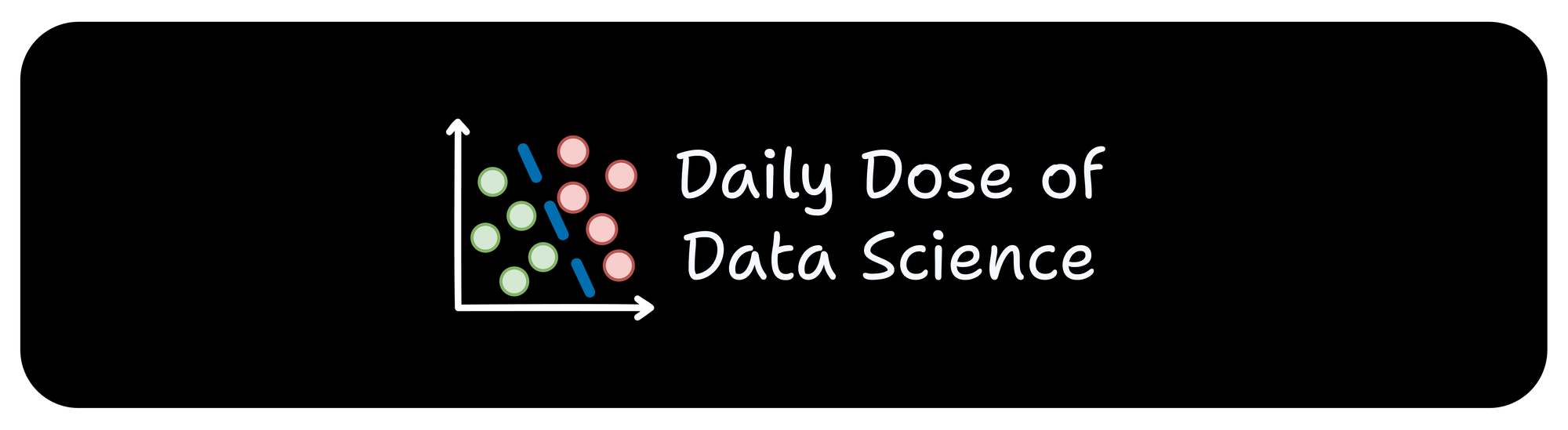
TODAY'S ISSUE
TODAY’S DAILY DOSE OF DATA SCIENCE
Intro to ReAct (Reasoning and Action) Agents
ReAct agents, short for Reasoning and Action agents, is a framework that combines the reasoning power of LLMs with the ability to take action!
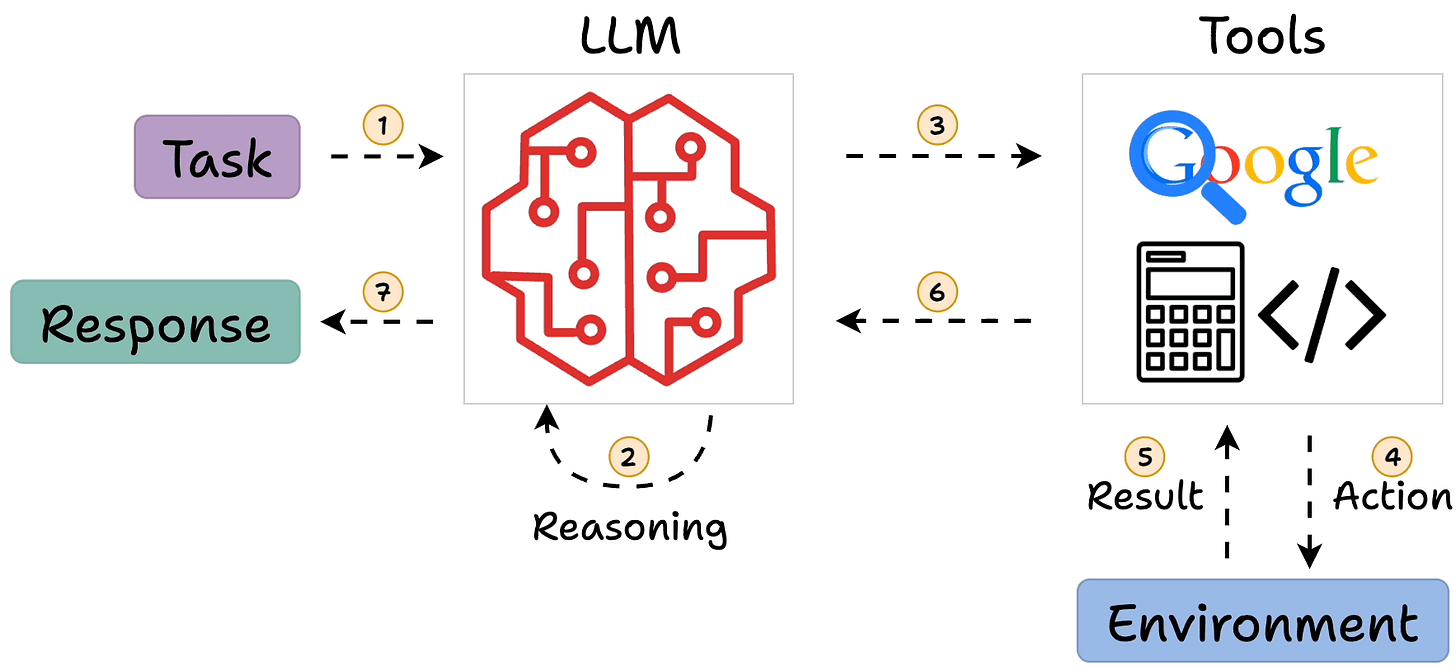
Today, let’s understand what ReAct agents are in detail and how to build one using Dynamiq.
What are ReACT agents?
ReACT agents integrate the thinking and reasoning capabilities of LLMs with actionable steps.
This allows the AI to comprehend, plan, and interact with the real world.
Simply put, it's like giving AI both a brain to figure out what to do and hands to actually do the work!
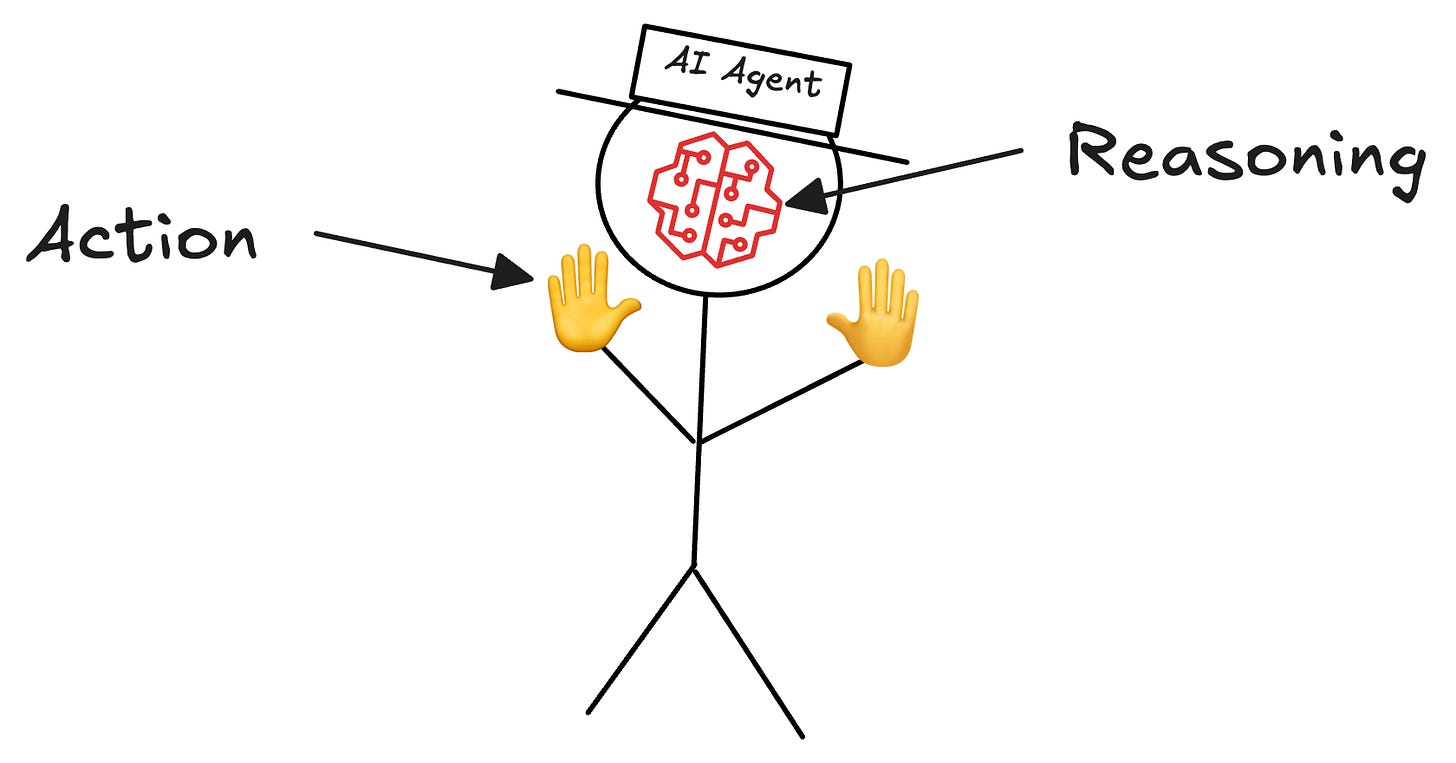
The video below explains this idea in much more depth.
Let’s get a bit more practical.
A real-world example
Imagine a ReACT agent helping a user decide whether to carry an umbrella.
Here's how the logical flow an agent should follow to answer this query →
I am in London, do I need an umbrella today?
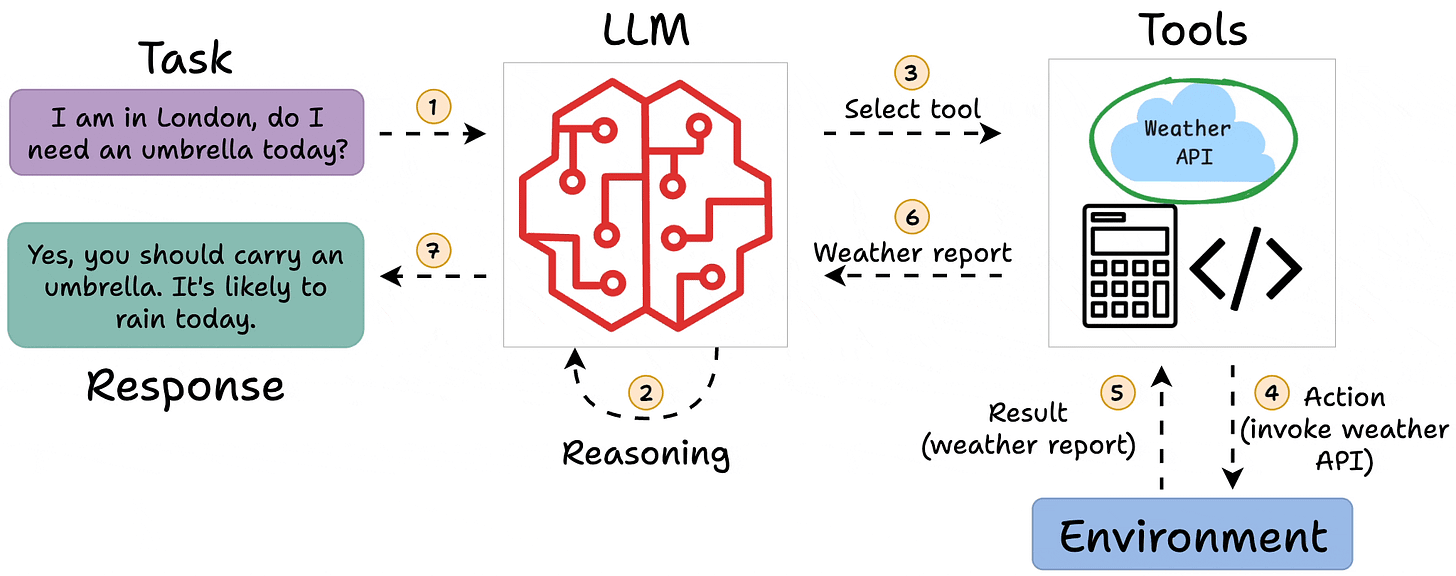
- Understand the query (language understanding)
- Select the tools it would need to generate a response (the weather API).
- Gather the weather report.
- Understand if it’s going to rain and produce an answer accordingly.
Next, let’s use Dynamiq to build a simple ReAct agent.
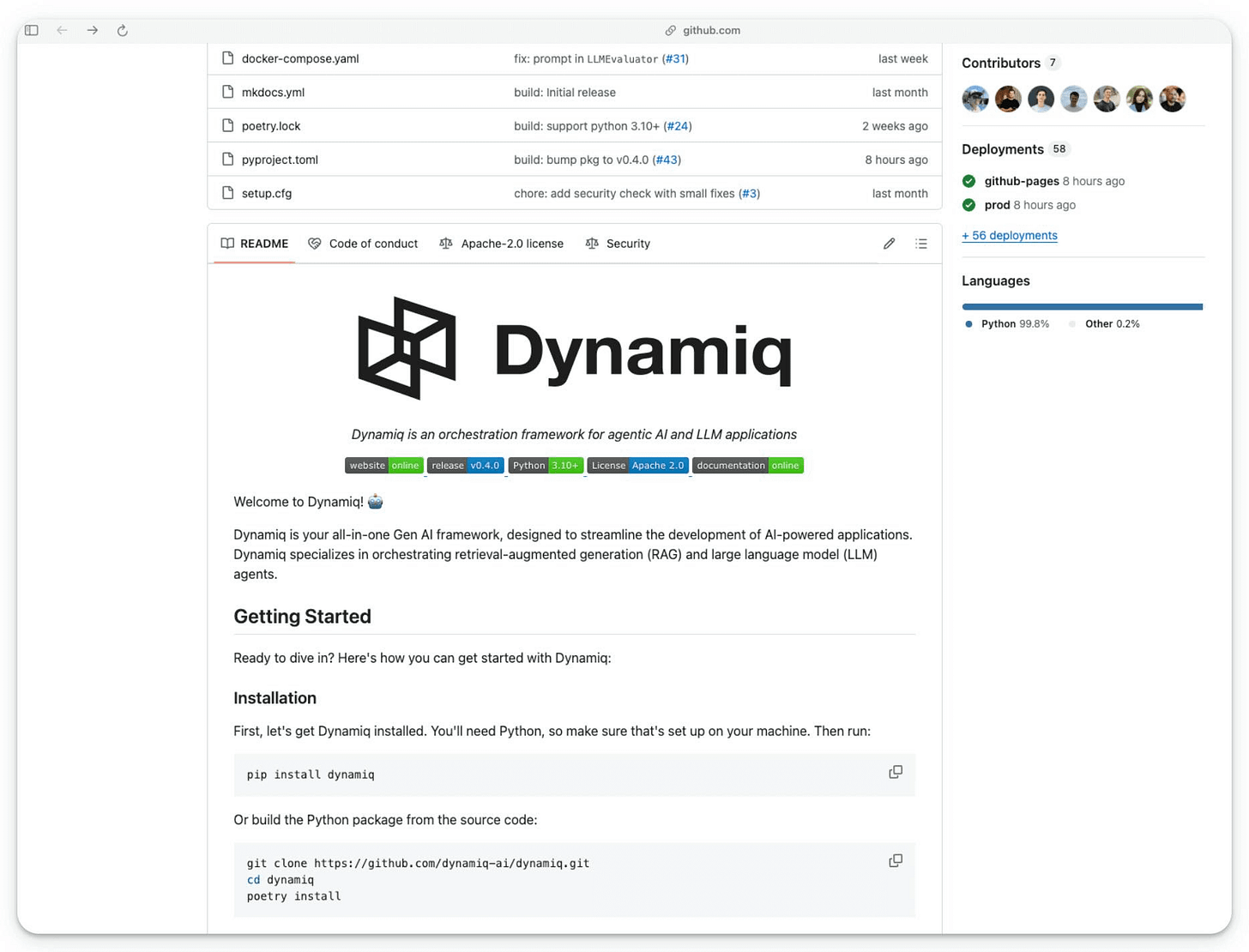
Dynamiq is a completely open-source, low-code, and all-in-one Gen AI framework for developing LLM applications with AI Agents and RAGs.
Here’s what stood out for me about Dynamiq:
- It seamlessly orchestrates multiple AI agents.
- It facilitates RAG applications.
- It easily manages complex LLM workflows.
- It has a highly intuitive API, as you’ll see shortly.
Building a ReAct Agent
Next, let’s build a simple ReAct agent.
1) Installation and setup
Get started by installing Dynamiq (pip install dynamiq
) and importing the necessary libraries:
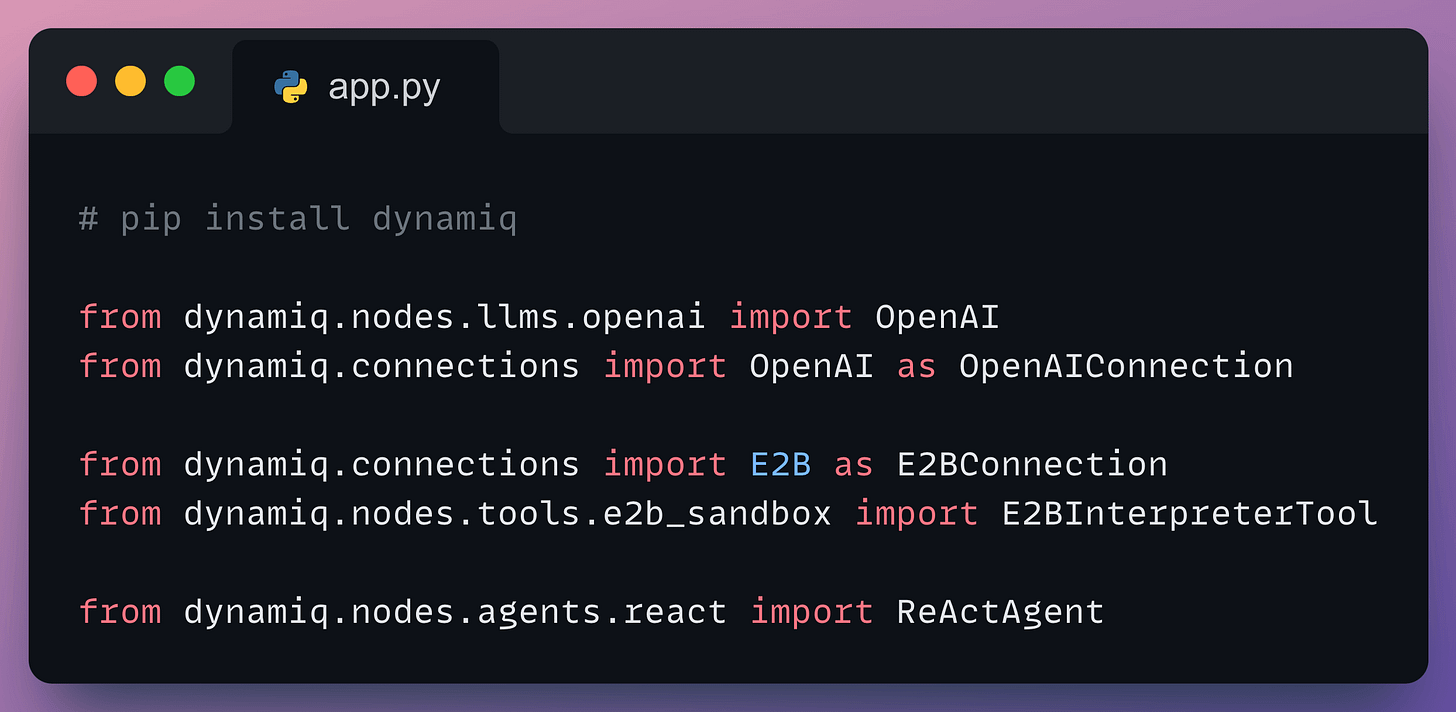
- We’ll use OpenAI for LLM.
- E2B is an open-source platform for running AI-generated code in cloud sandboxes. It lets the agent execute its own code (invoking weather API, for instance).
- The
ReActAgent
class orchestrates the ReAct agent.
2) Setup tools and LLM
Next, we set up E2B and an LLM as follows:
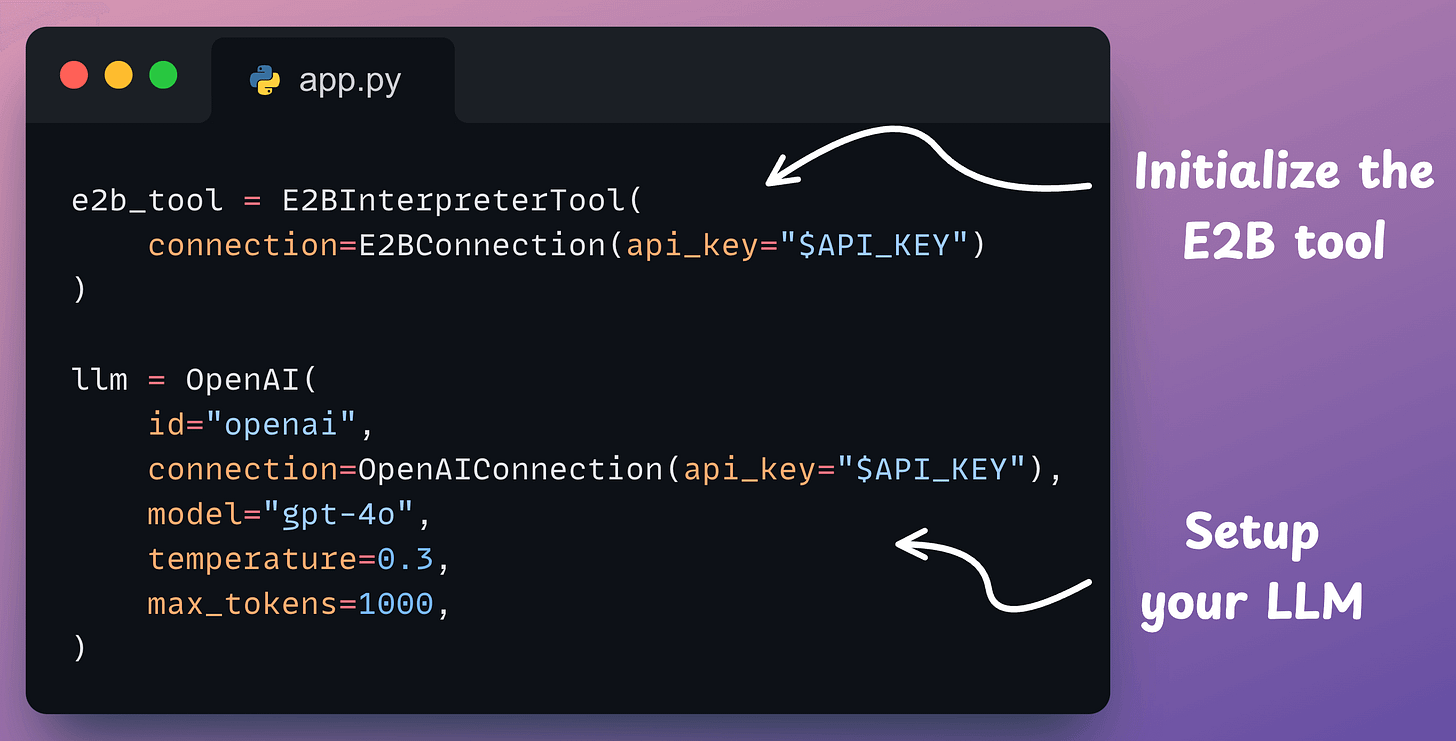
The code above is pretty self-explanatory.
3) Create an Agent
Last step!
Dynamiq provides a straightforward interface for creating an agent. You just need to specify the LLM, the tools it can access, and its role.
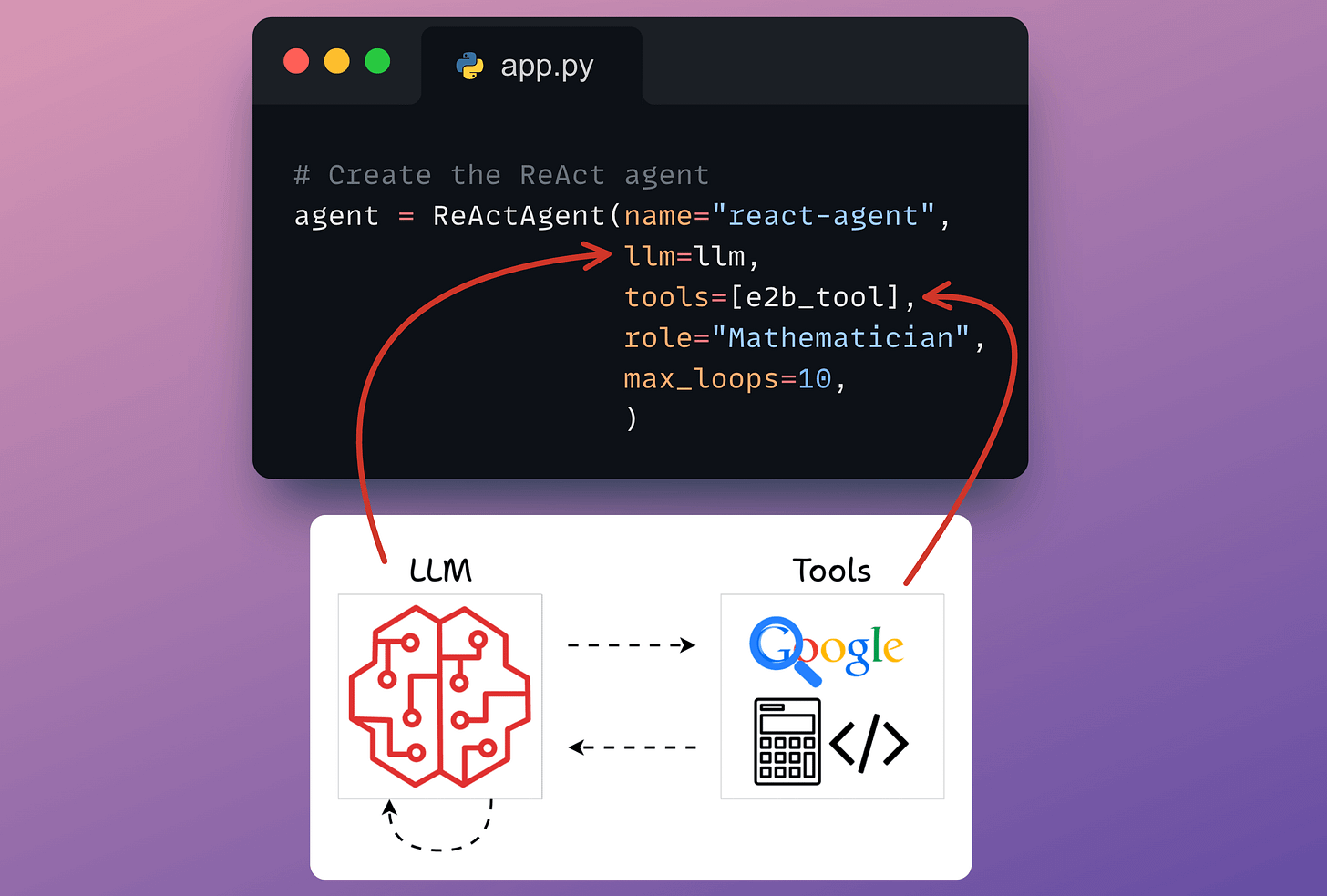
Done!
4) Run the Agent
Finally, when everything is set up.
We provide a query and run the agent as follows:
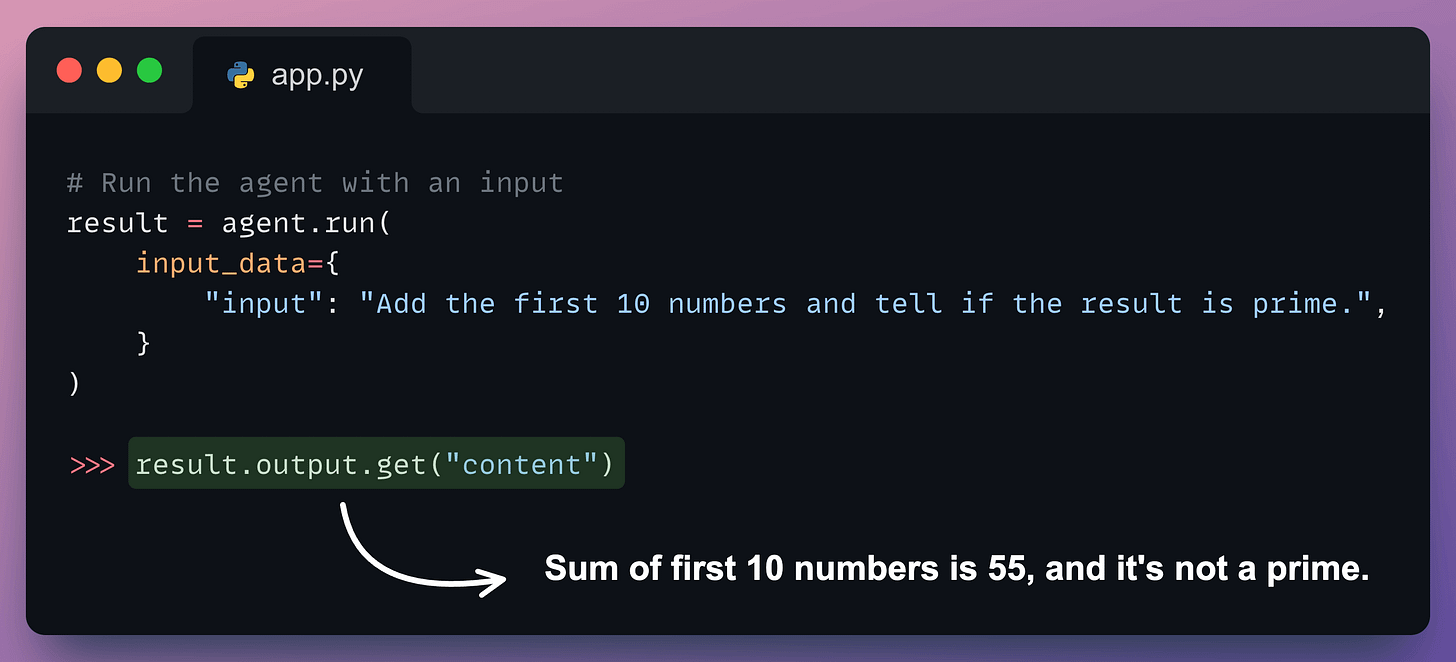
Perfect. It produces the correct output.
Conclusion
We have been using Dynamiq for a while now.
It is a fully Pythonic, open-source, low-code, and all-in-one Gen AI framework for developing LLM applications with AI Agents and RAGs.
Here’s what I like about Dynamiq:
- It seamlessly orchestrates multiple AI agents.
- It easily manages complex LLM workflows.
- It facilitates RAG applications.
- It has a highly intuitive API, as we saw in the above demo.
All this makes it 10x easier to build production-ready AI applications.
If you're an AI Engineer, Dynamiq will save you hours of tedious orchestrations!
Several demo examples of simple LLM flows, RAG apps, ReAct agents, multi-agent orchestration, etc., are available on Dynamiq's GitHub.
Support their work by starring this GitHub repo: Dynamiq's GitHub.
Thanks to ​Dynamiq for showing us their easy-to-use and highly powerful Gen AI framework and partnering with us on today's issue.
👉 Over to you: What else would you like to learn about AI agents?
Thanks for reading!
IN CASE YOU MISSED IT
​Prompting vs. RAG vs. Fine-tuning​
If you are building real-world LLM-based apps, it is unlikely you can start using the model right away without adjustments. To maintain high utility, you either need:
- Prompt engineering
- Fine-tuning
- RAG
- Or a hybrid approach (RAG + fine-tuning)
The following visual will help you decide which one is best for you:
​Read more in-depth insights into Prompting vs. RAG vs. Fine-tuning here →
ROADMAP
From local ML to production ML
Once a model has been trained, we move to productionizing and deploying it.
If ideas related to production and deployment intimidate you, here’s a quick roadmap for you to upskill (assuming you know how to train a model):
- First, you would have to compress the model and productionize it. Read these guides:
- Reduce their size with ​Model Compression techniques​.
- ​Supercharge ​​PyTorch Models​​ With TorchScript.​
- If you use sklearn, learn how to ​optimize them with tensor operations​.
- Next, you move to deployment. ​Here’s a beginner-friendly hands-on guide​ that teaches you how to deploy a model, manage dependencies, set up model registry, etc.
- Although you would have tested the model locally, it is still wise to test it in production. There are risk-free (or low-risk) methods to do that. ​Learn what they are and how to implement them here​.
This roadmap should set you up pretty well, even if you have NEVER deployed a single model before since everything is practical and implementation-driven.
THAT'S A WRAP
No-Fluff Industry ML resources to
Succeed in DS/ML roles
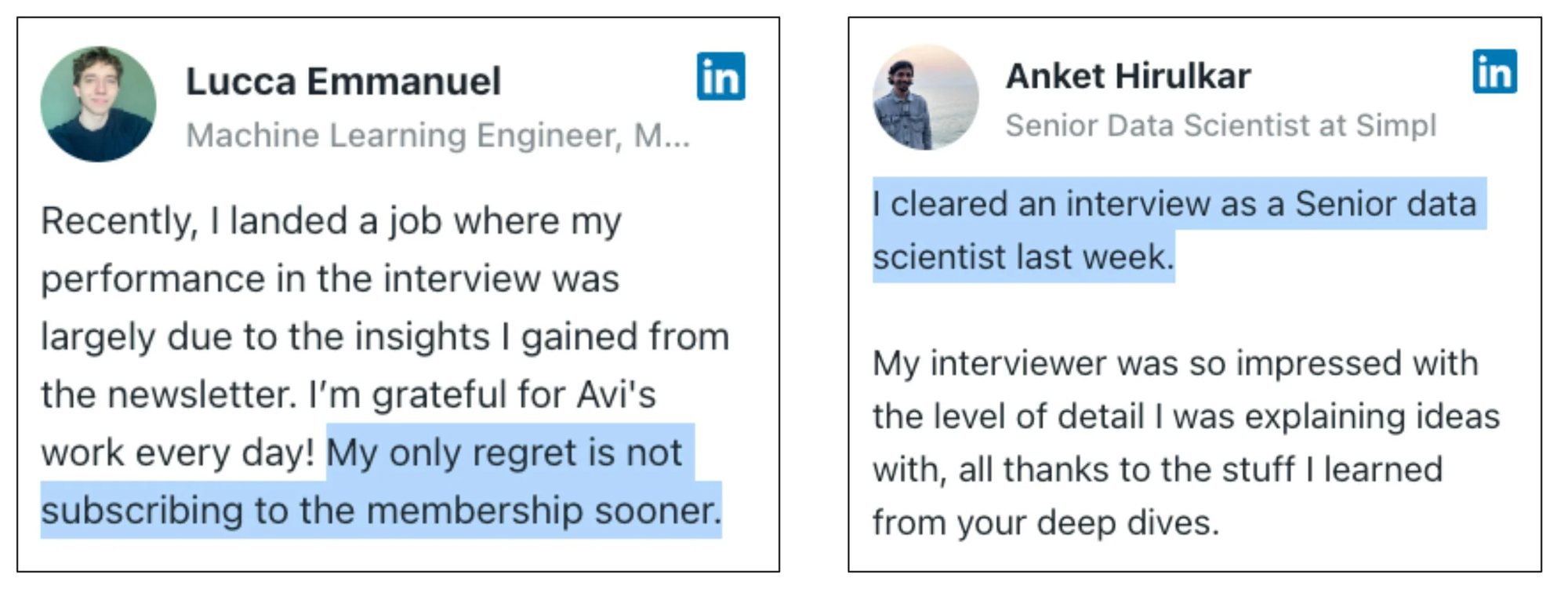
At the end of the day, all businesses care about impact. That’s it!
- Can you reduce costs?
- Drive revenue?
- Can you scale ML models?
- Predict trends before they happen?
We have discussed several other topics (with implementations) in the past that align with such topics.
Here are some of them:
- Learn sophisticated graph architectures and how to train them on graph data in this crash course.
- So many real-world NLP systems rely on pairwise context scoring. Learn scalable approaches here.
- Run large models on small devices using Quantization techniques.
- Learn how to generate prediction intervals or sets with strong statistical guarantees for increasing trust using Conformal Predictions.
- Learn how to identify causal relationships and answer business questions using causal inference in this crash course.
- Learn how to scale and implement ML model training in this practical guide.
- Learn 5 techniques with implementation to reliably test ML models in production.
- Learn how to build and implement privacy-first ML systems using Federated Learning.
- Learn 6 techniques with implementation to compress ML models.
All these resources will help you cultivate key skills that businesses and companies care about the most.
SPONSOR US
Advertise to 600k+ data professionals
Our newsletter puts your products and services directly in front of an audience that matters — thousands of leaders, senior data scientists, machine learning engineers, data analysts, etc., around the world.