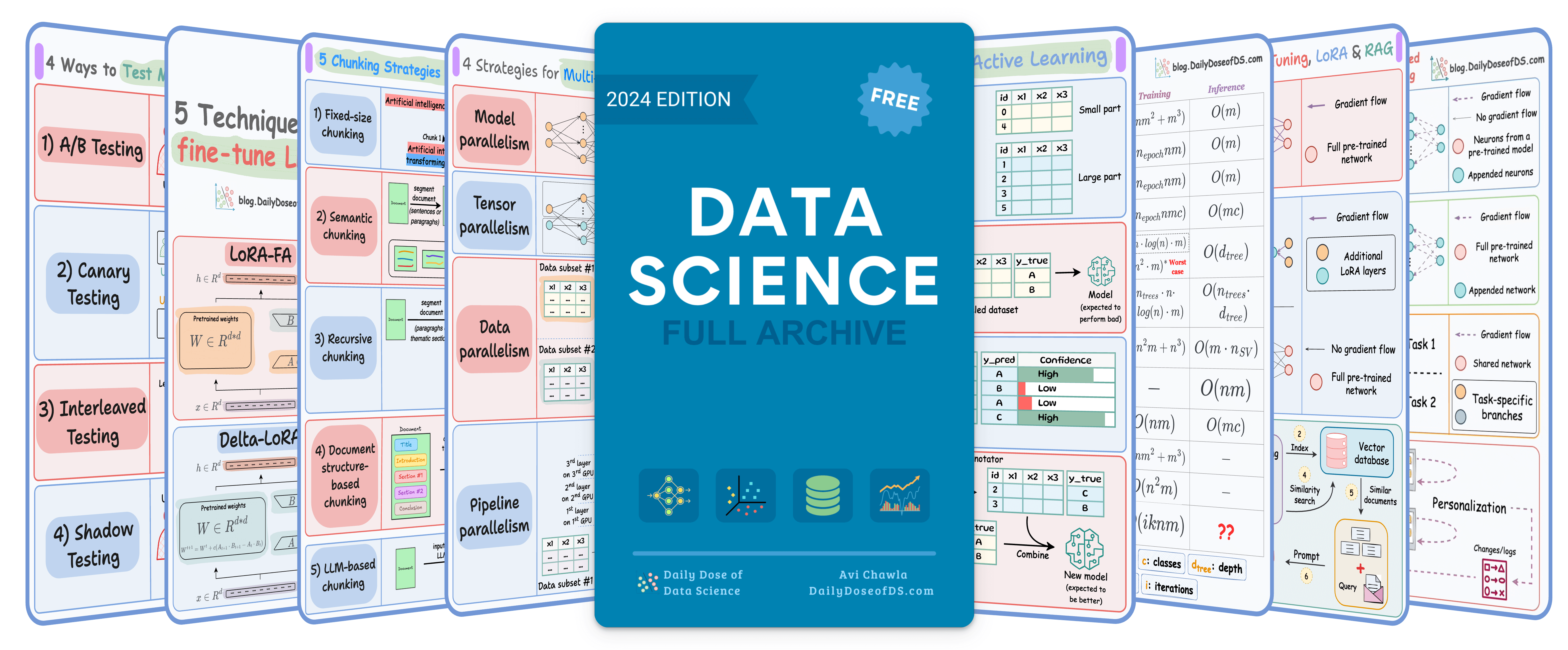
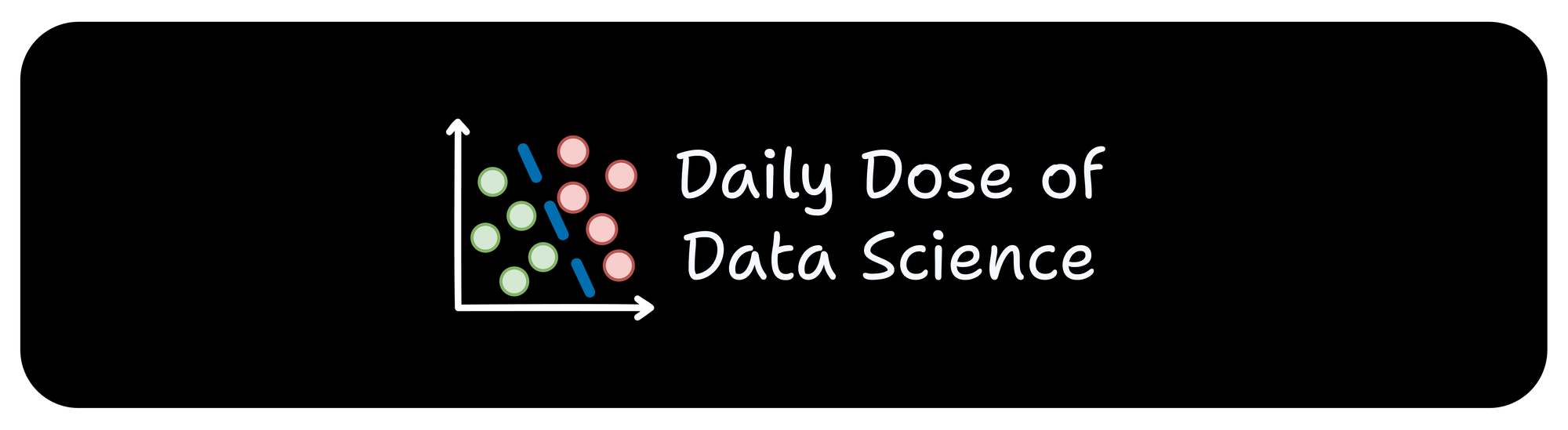
TODAY'S ISSUE
TODAY’S DAILY DOSE OF DATA SCIENCE
8 Classic Alternatives to Traditional Plots
Scatter plots, bar plots, line plots, box plots, and heatmaps are the most frequently used plots for data visualization.
Although they are simple and known to almost everyone, I believe they are not the right choice to cover every possible scenario.
Instead, many other plots originate from these standard plots that can be much more suitable, if used appropriately.
Therefore, today, let’s discuss a few alternatives to these popular plots.
I will also explain specific situations where they can be more useful over standard plots.
Let’s begin!
#1) Size-encoded heatmaps
A traditional heatmap represents the values using a color scale. Yet, mapping the cell color to exact numbers is still challenging.
Embedding a size component to heatmaps can be extremely helpful in such cases.
In essence, the bigger the size, the higher the absolute value:
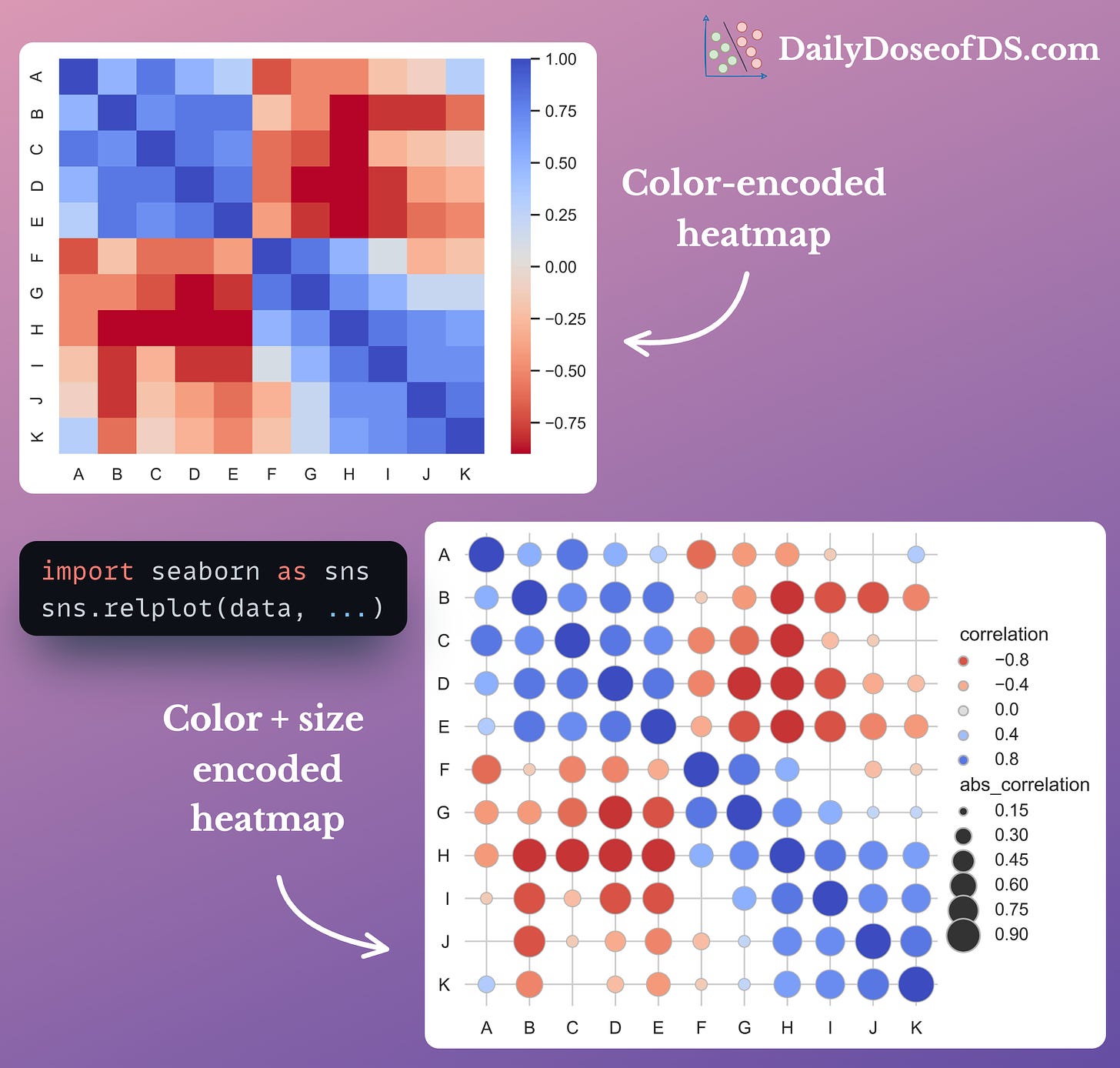
This is especially useful to make heatmaps cleaner, as many values nearer to zero will immediately shrink.
#2) Waterfall charts
To visualize the change in value over time, a line (or bar) plot may not always be an apt choice.
This is because a line plot (or bar plot) depicts the actual values in the chart. Thus, it is difficult to visually estimate the scale and direction of incremental changes.
Instead, you can use a waterfall chart.
It elegantly depicts these rolling differences, as depicted below:
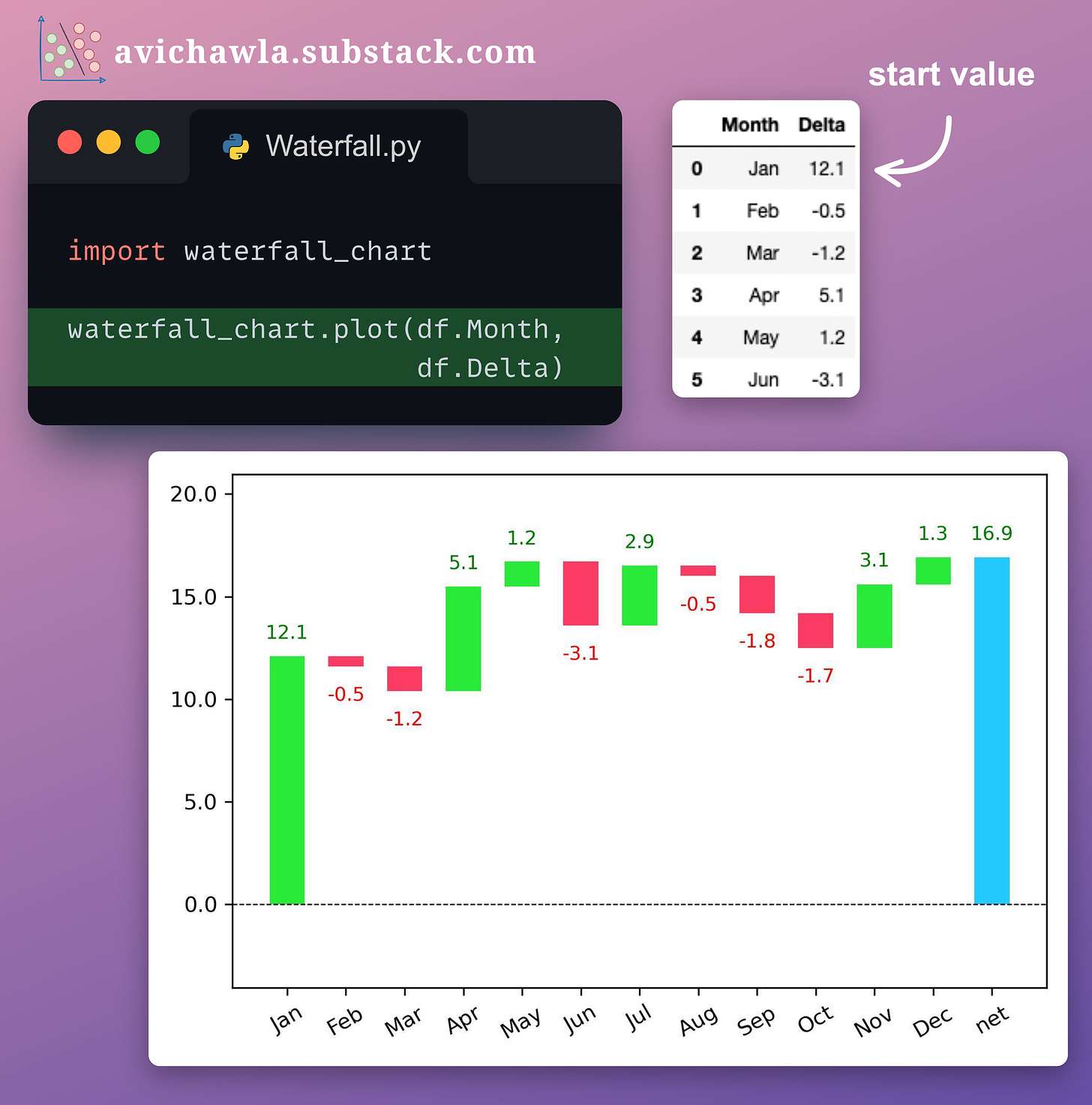
Here, the start and final values are represented by the first and last bars.
Also, the consecutive changes are automatically color-coded, making them easier to interpret.
#3) Bump charts
When visualizing the change in rank over time of multiple categories, using a bar chart may not be appropriate.
This is because bar charts quickly become cluttered with many categories.
Instead, try Bump Charts. They are specifically used to visualize the rank of different items over time.
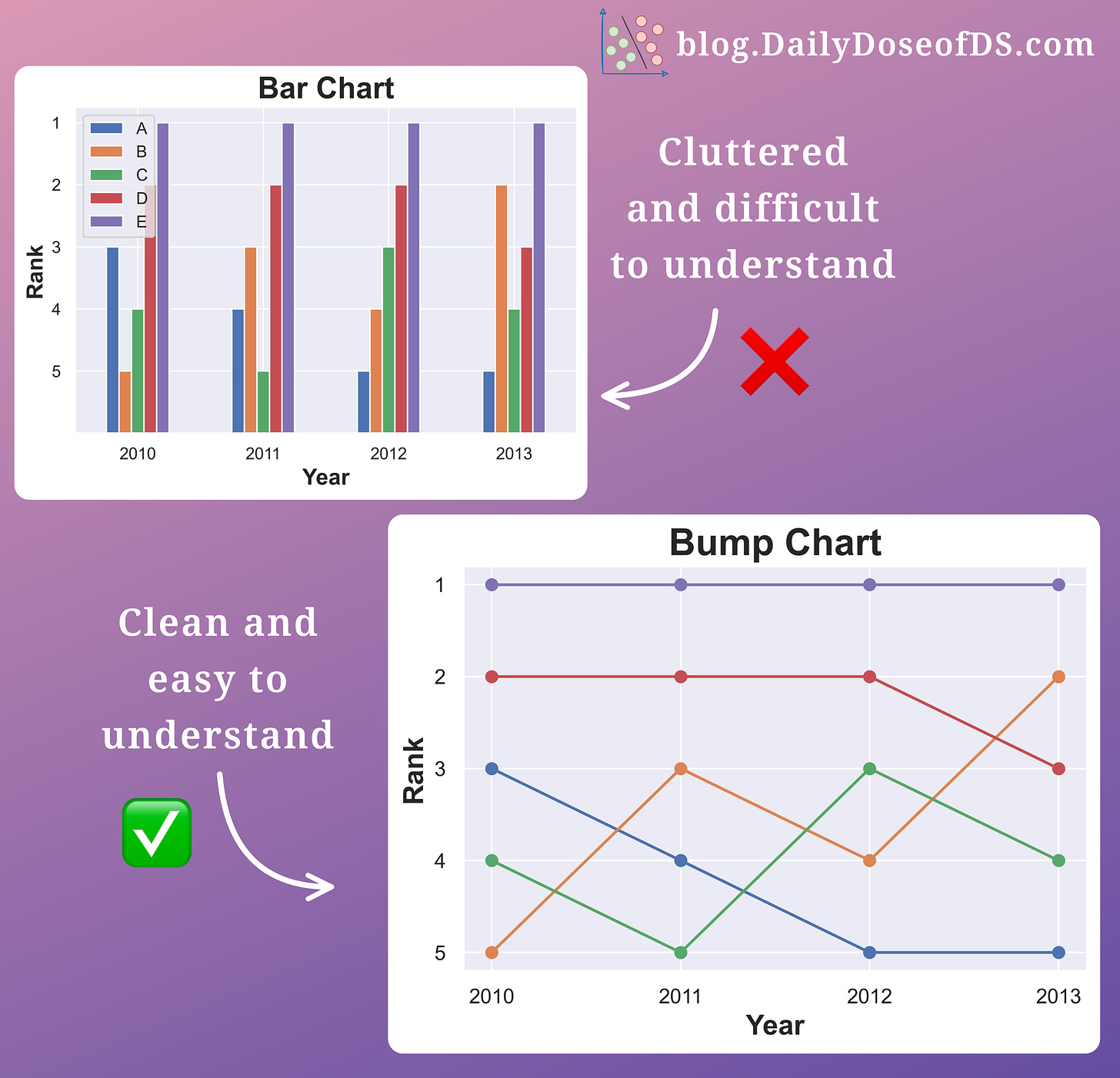
Comparing the bar chart and bump chart above, it is far easier to interpret the change in rank with a bump chart rather than a bar chart.
#4) Raincloud Plots
Visualizing data distributions using box plots and histograms can be misleading at times.
This is because:
- It is possible to get the same box plot with entirely different data.
- Altering the number of bins changes the shape of a histogram.
Thus, to avoid misleading conclusions, it is always recommended to plot the data distribution as precisely as possible.
These include:
- Box plots for data statistics.
- Strip plots for data overview.
- KDE plots for the probability distribution of data.
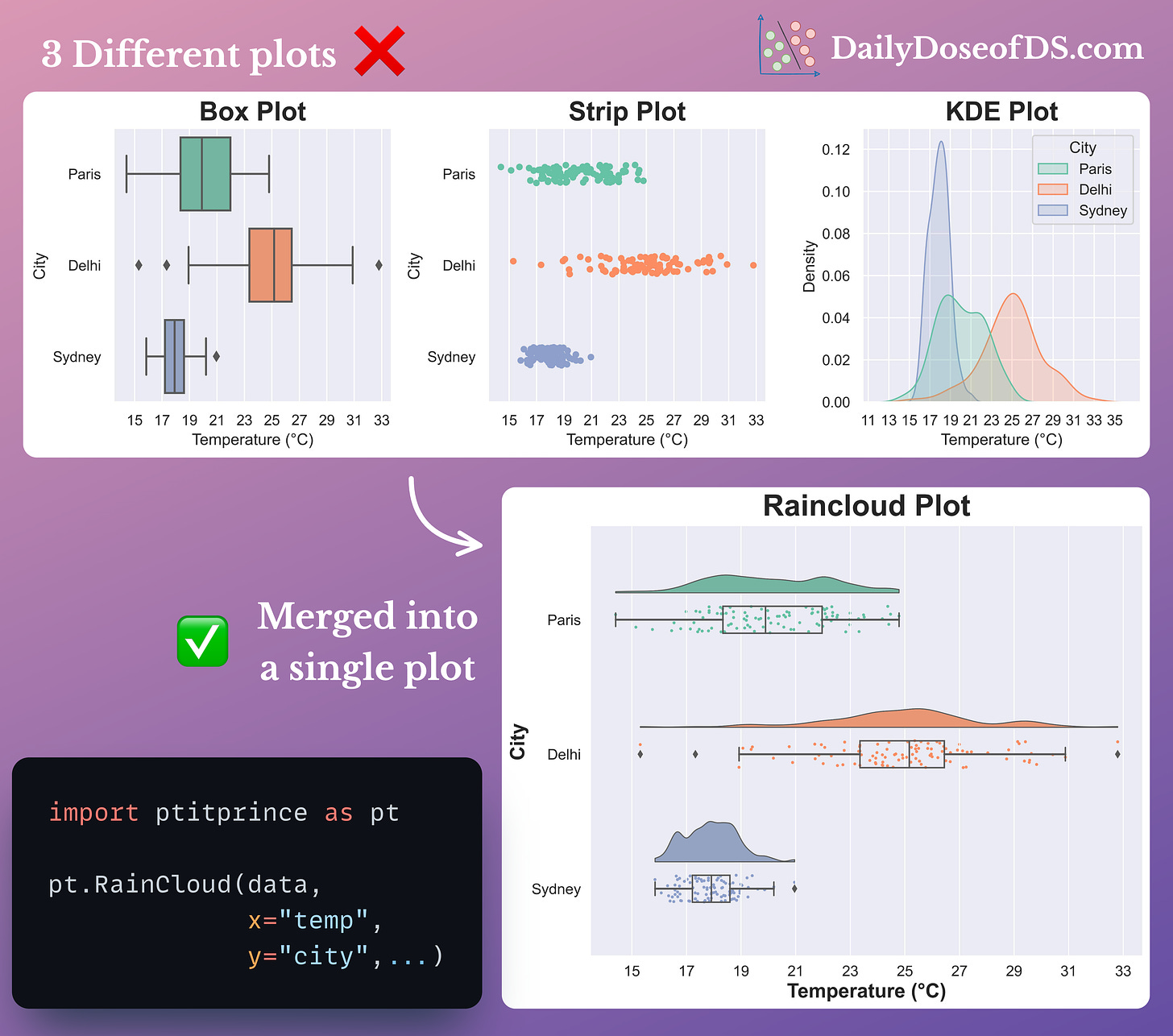
With Raincloud plots, you can:
- Combine multiple plots to prevent incorrect/misleading conclusions
- Reduce clutter and enhance clarity
- Improve comparisons between groups
- Capture different aspects of the data through a single plot
#5-6) Hexbin and Density Plots
Scatter plots can get too dense to interpret when you have thousands of data points.
Instead, you can replace them with Hexbin plots.
Hexbin plots bin the area of a chart into hexagonal regions. Each region is assigned a color intensity based on the method of aggregation used (the number of points, for instance).
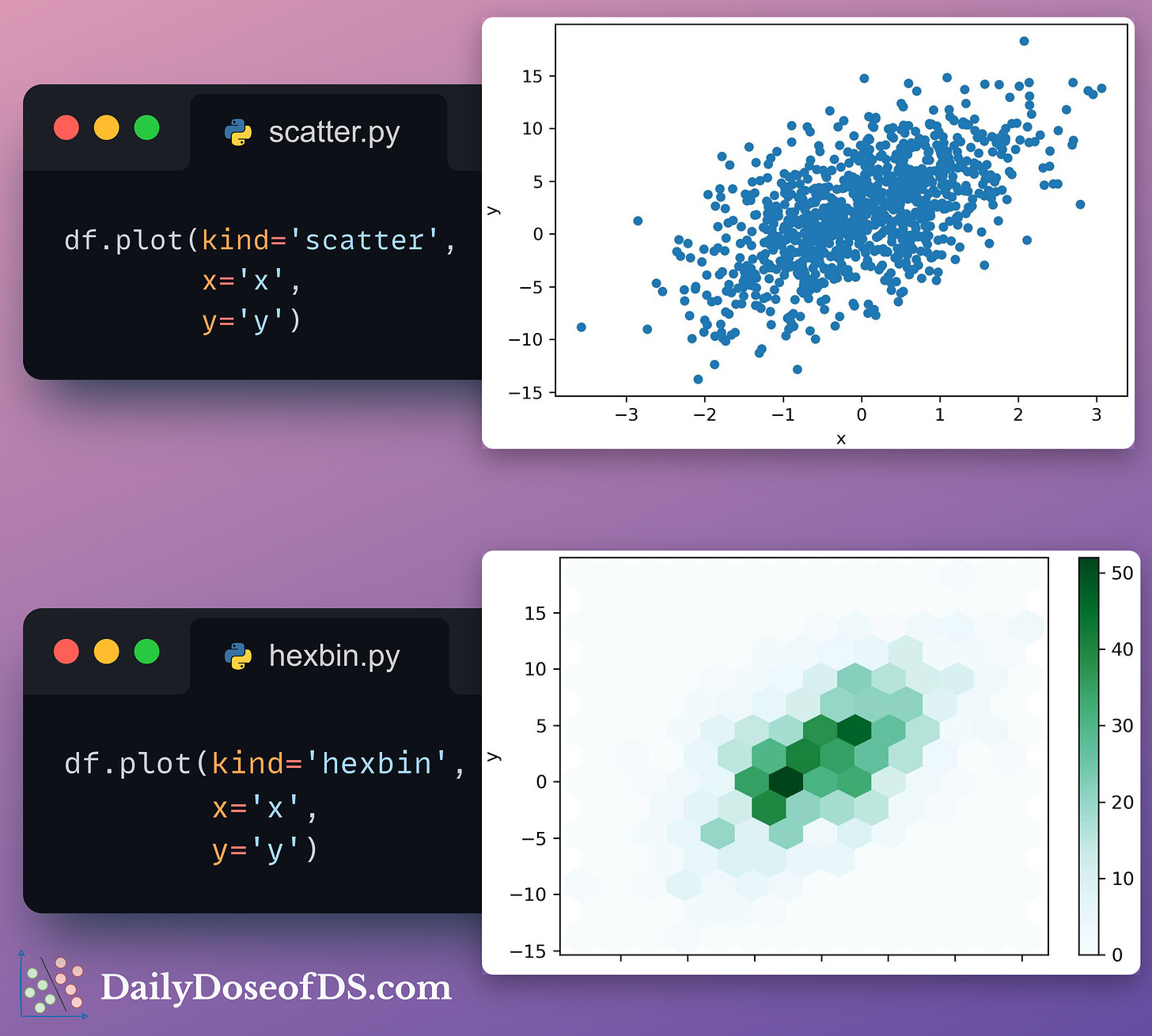
Another choice is a density plot, which illustrates the distribution of points in a two-dimensional space.
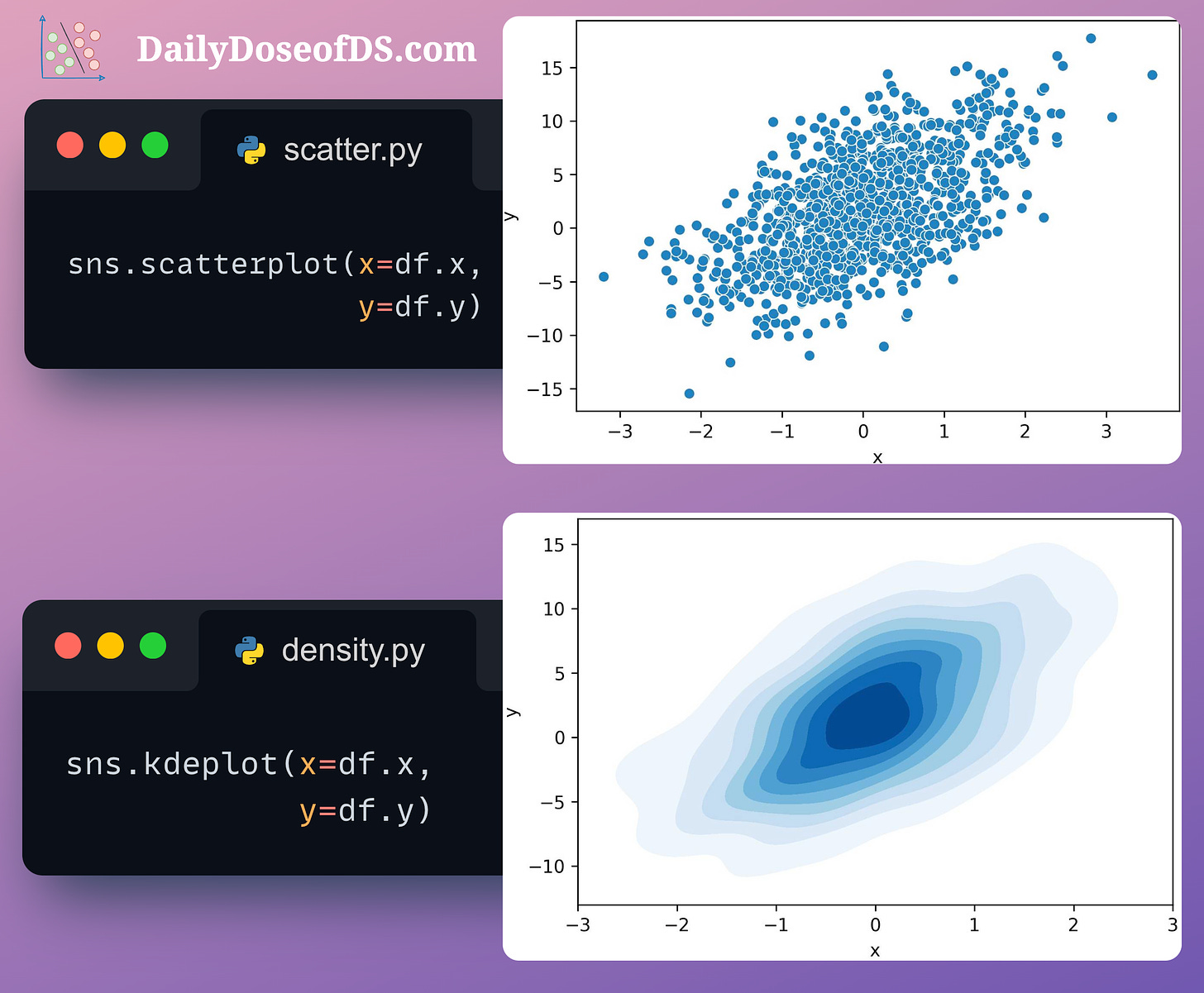
A contour is created by connecting points of equal density. In other words, a single contour line depicts an equal density of data points.
#7-8) Bubble charts and Dot plots
As discussed above, bar plots quickly get messy and cluttered as the number of categories increases.
A bubble plot is often a better alternative in such cases.
They are like scatter plots but:
- with one categorical axis
- and one continuous axis
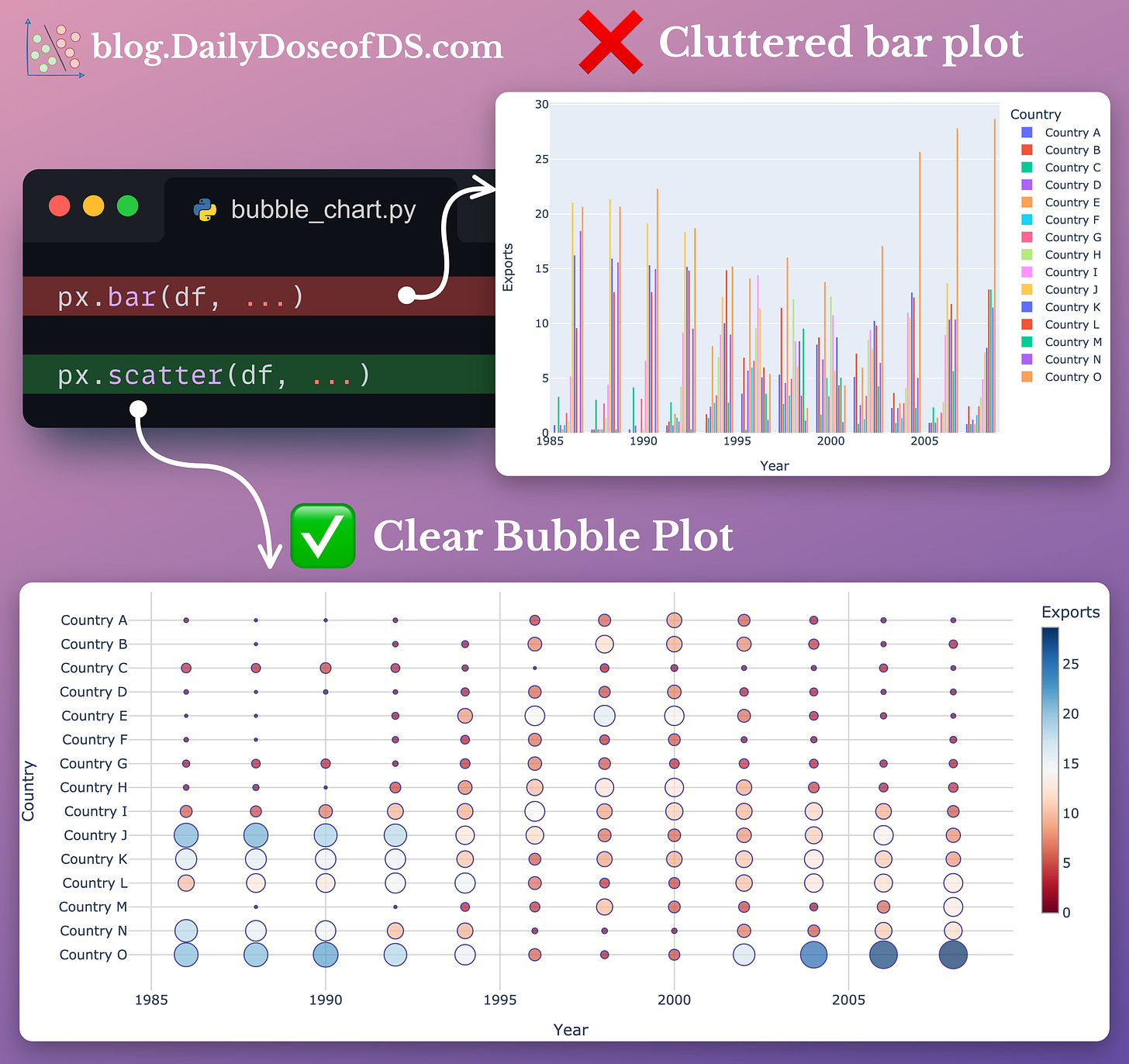
As depicted above:
- It is difficult to interpret the bar plot because it has too many bars packed into a small space,
- But size-encoded bubbles make it pretty easy to visualize the change over time.
Another alternative to bar plots in such situations is dot plots.
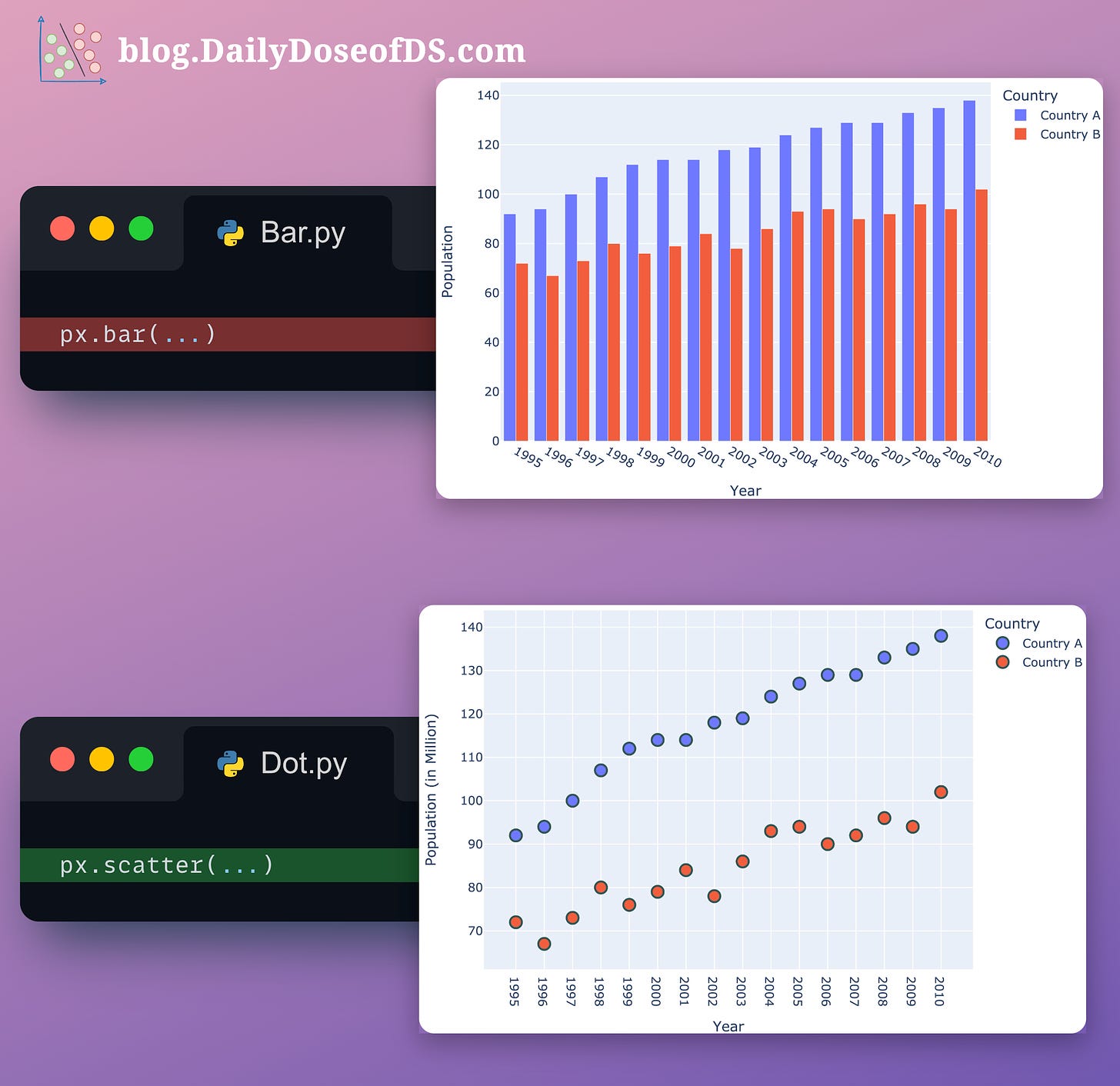
Both dot plots and bubble charts are based on the idea that, at times, when we have a bar plot with many bars, we’re often not paying attention to the individual bar lengths.
Instead, we mostly consider the individual endpoints that denote the total value.
These plots precisely help us depict that while also eliminating the long bars of little to no use.
IN CASE YOU MISSED IT
​Traditional RAG vs. HyDE​
One critical problem with the traditional ​​​​RAG system​​​​ is that questions are not semantically similar to their answers.
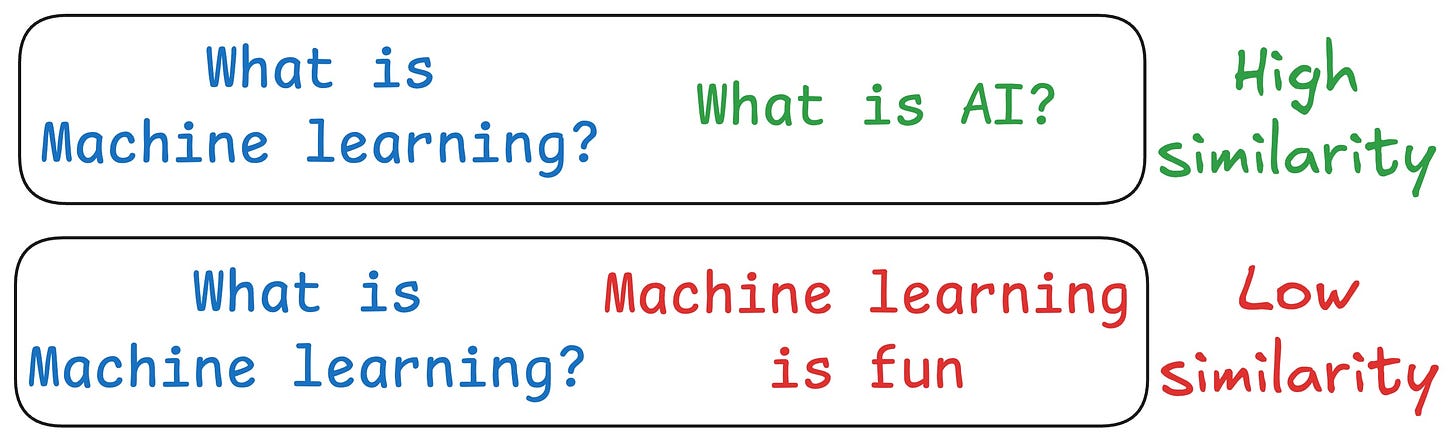
As a result, several irrelevant contexts get retrieved during the retrieval step due to a higher cosine similarity than the documents actually containing the answer.
HyDE solves this.
The following visual depicts how it differs from traditional RAG and HyDE.
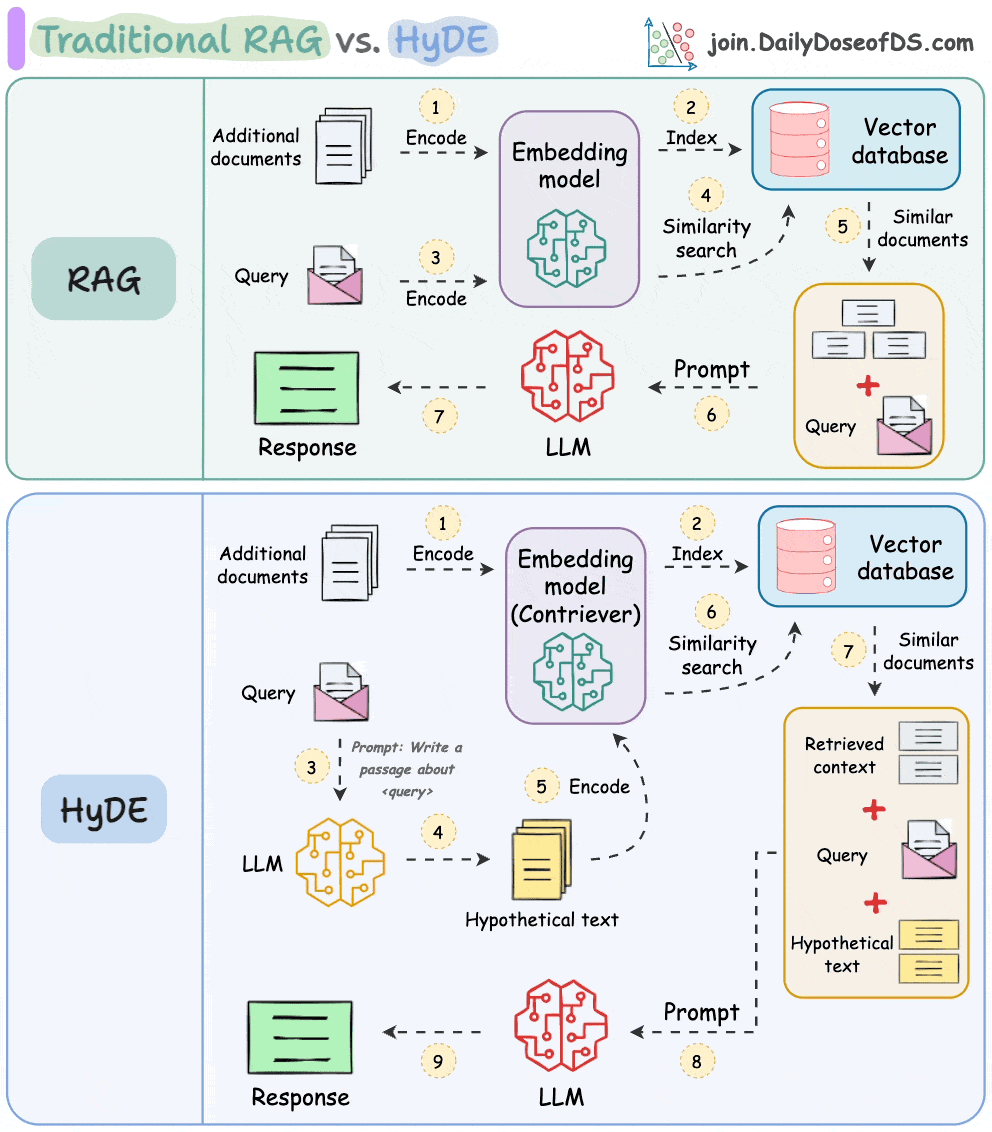
​We covered this in detail in a newsletter issue published last week →​
TRULY REPRODUCIBLE ML
Data Version Control​
Versioning GBs of datasets is practically impossible with GitHub because it imposes an upper limit on the file size we can push to its remote repositories.
That is why Git is best suited for versioning codebase, which is primarily composed of lightweight files.
However, ML projects are not solely driven by code.
Instead, they also involve large data files, and across experiments, these datasets can vastly vary.
To ensure proper reproducibility and experiment traceability, it is also necessary to ​version datasets​.
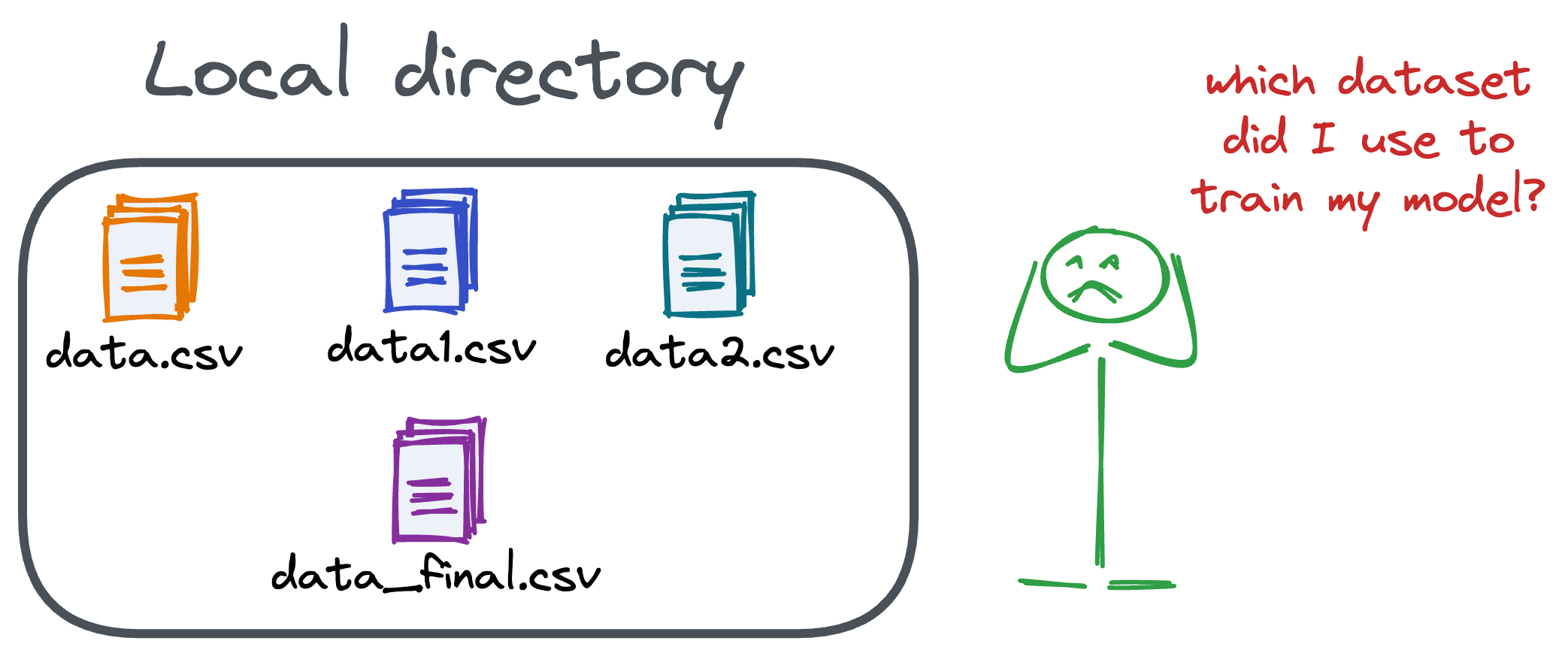
Data version control (DVC) solves this problem.
The core idea is to integrate another version controlling system with Git, specifically used for large files.
​Here's everything you need to know ​​(with implementation)​​ about building 100% reproducible ML projects →​
THAT'S A WRAP
No-Fluff Industry ML resources to
Succeed in DS/ML roles
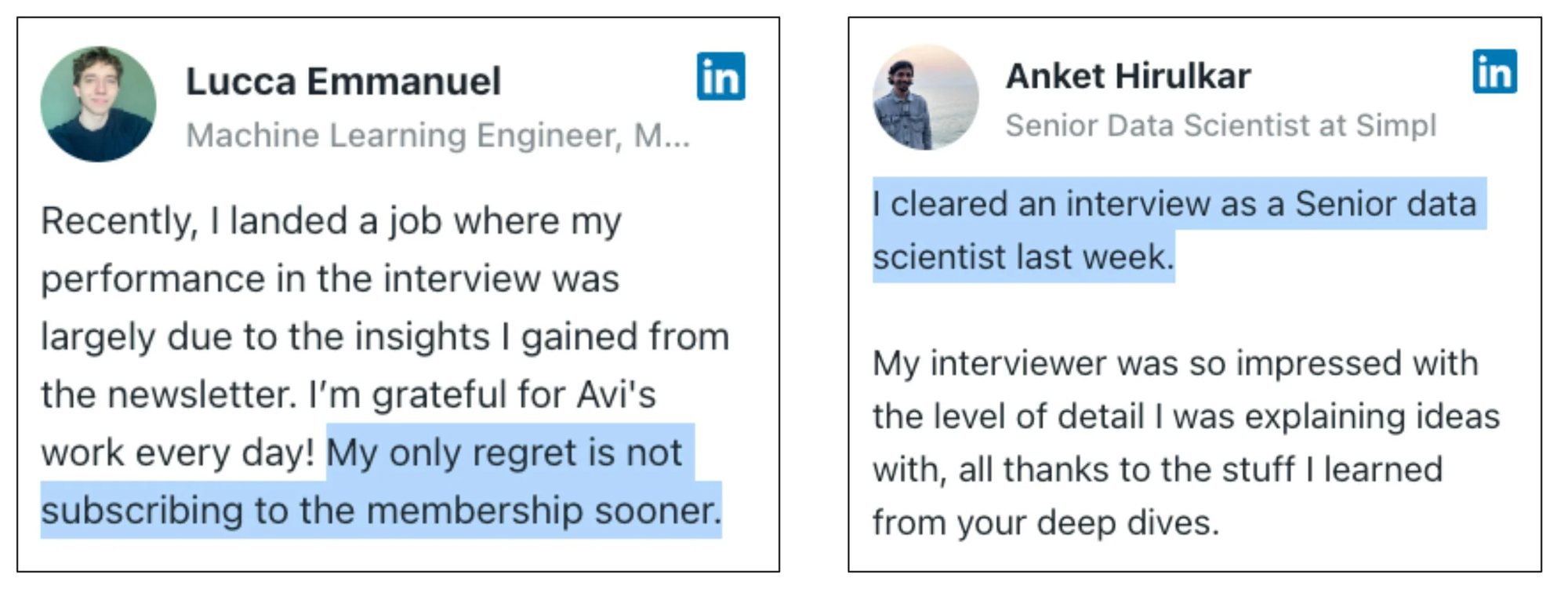
At the end of the day, all businesses care about impact. That’s it!
- Can you reduce costs?
- Drive revenue?
- Can you scale ML models?
- Predict trends before they happen?
We have discussed several other topics (with implementations) in the past that align with such topics.
Here are some of them:
- Learn sophisticated graph architectures and how to train them on graph data in this crash course.
- So many real-world NLP systems rely on pairwise context scoring. Learn scalable approaches here.
- Run large models on small devices using Quantization techniques.
- Learn how to generate prediction intervals or sets with strong statistical guarantees for increasing trust using Conformal Predictions.
- Learn how to identify causal relationships and answer business questions using causal inference in this crash course.
- Learn how to scale and implement ML model training in this practical guide.
- Learn 5 techniques with implementation to reliably test ML models in production.
- Learn how to build and implement privacy-first ML systems using Federated Learning.
- Learn 6 techniques with implementation to compress ML models.
All these resources will help you cultivate key skills that businesses and companies care about the most.
SPONSOR US
Advertise to 600k+ data professionals
Our newsletter puts your products and services directly in front of an audience that matters — thousands of leaders, senior data scientists, machine learning engineers, data analysts, etc., around the world.