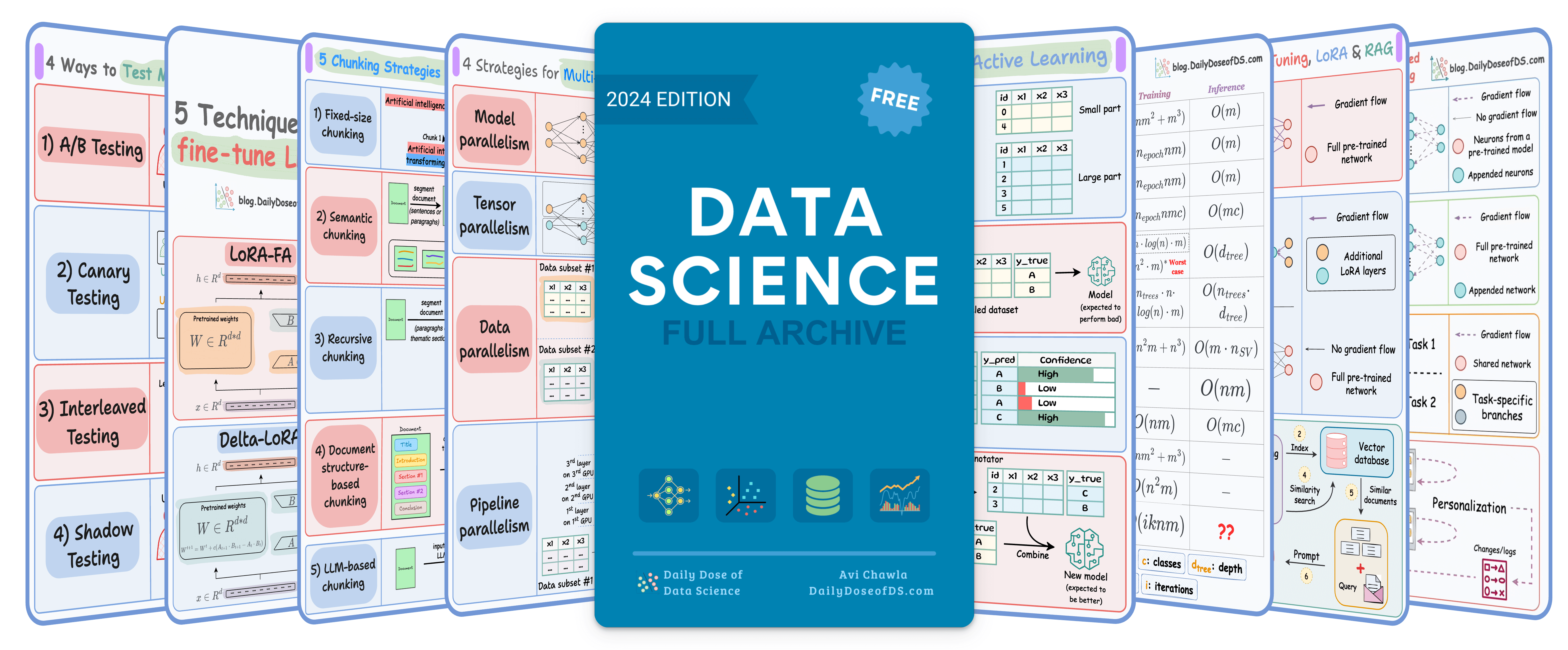
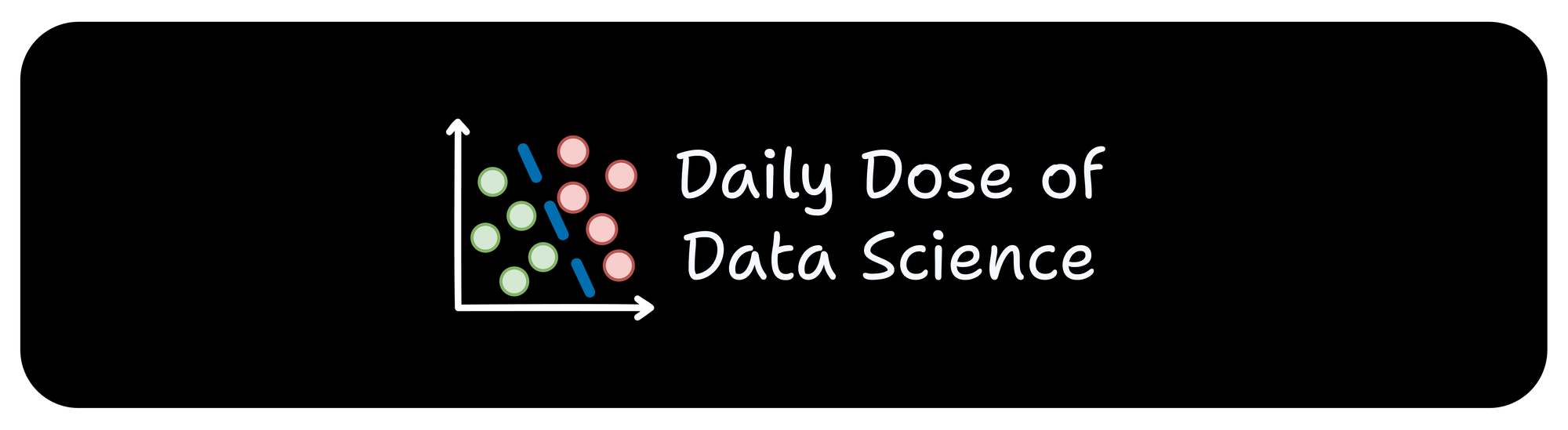
TODAY'S ISSUE
TOGETHER WITH BRILLIANT
AI isn’t magic. It’s math.
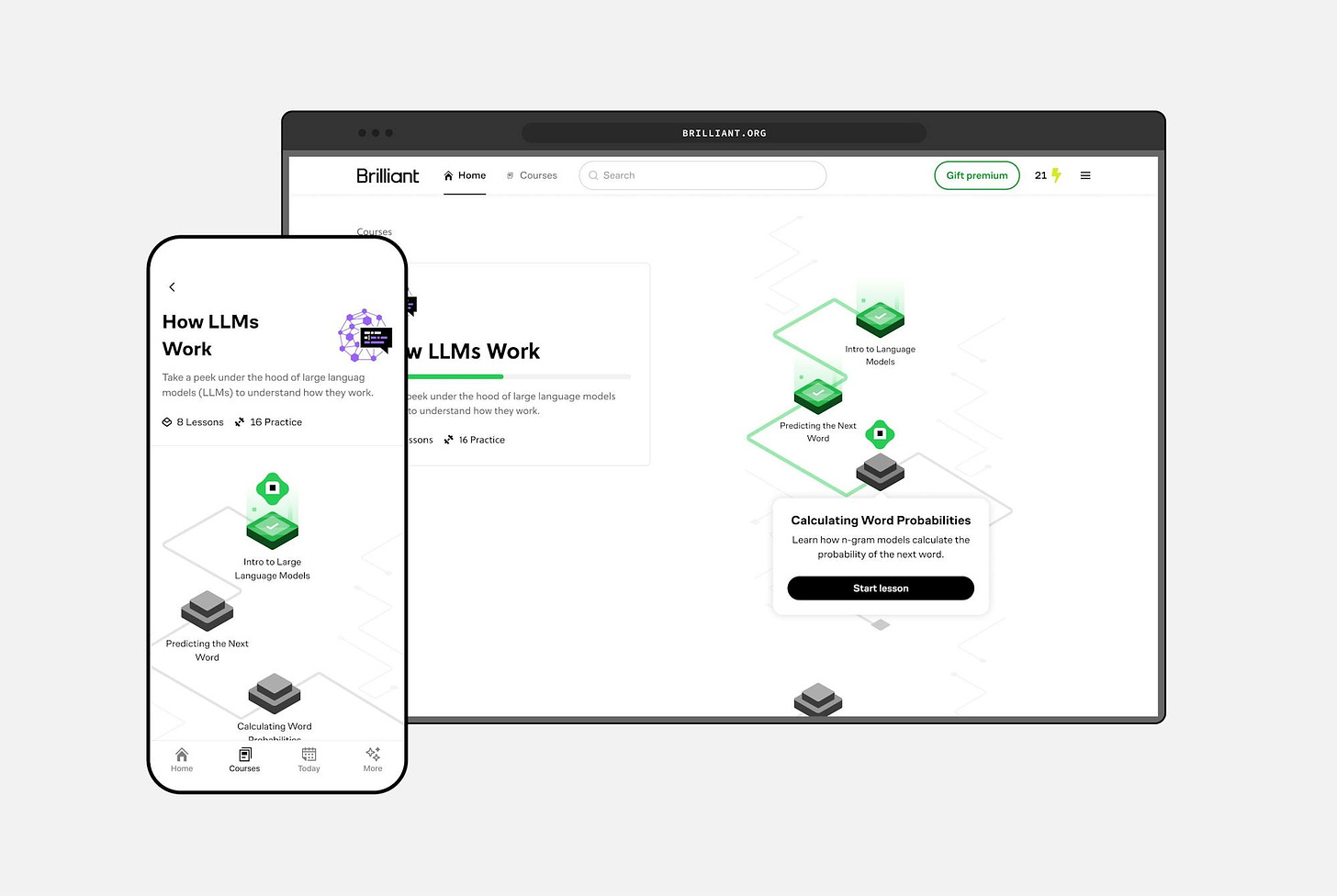
Understand the concepts powering technology like ChatGPT in minutes a day with Brilliant.
Thousands of quick, interactive lessons in AI, programming, logic, data science, and more make it easy. Try it free for 30 days.
Thanks to Brilliant for sponsoring today’s issue.
TODAY’S DAILY DOSE OF DATA SCIENCE
From PyTorch to PyTorch Fabric
PyTorch gives so much flexibility and control. But it leads to a ton of boilerplate code.
​PyTorch Lightning, however:
- Massively reduces the boilerplate code.
- Allow us to use distributed training features like DDP, FSDP, DeepSpeed, mixed precision training, and more, by directly specifying parameters:
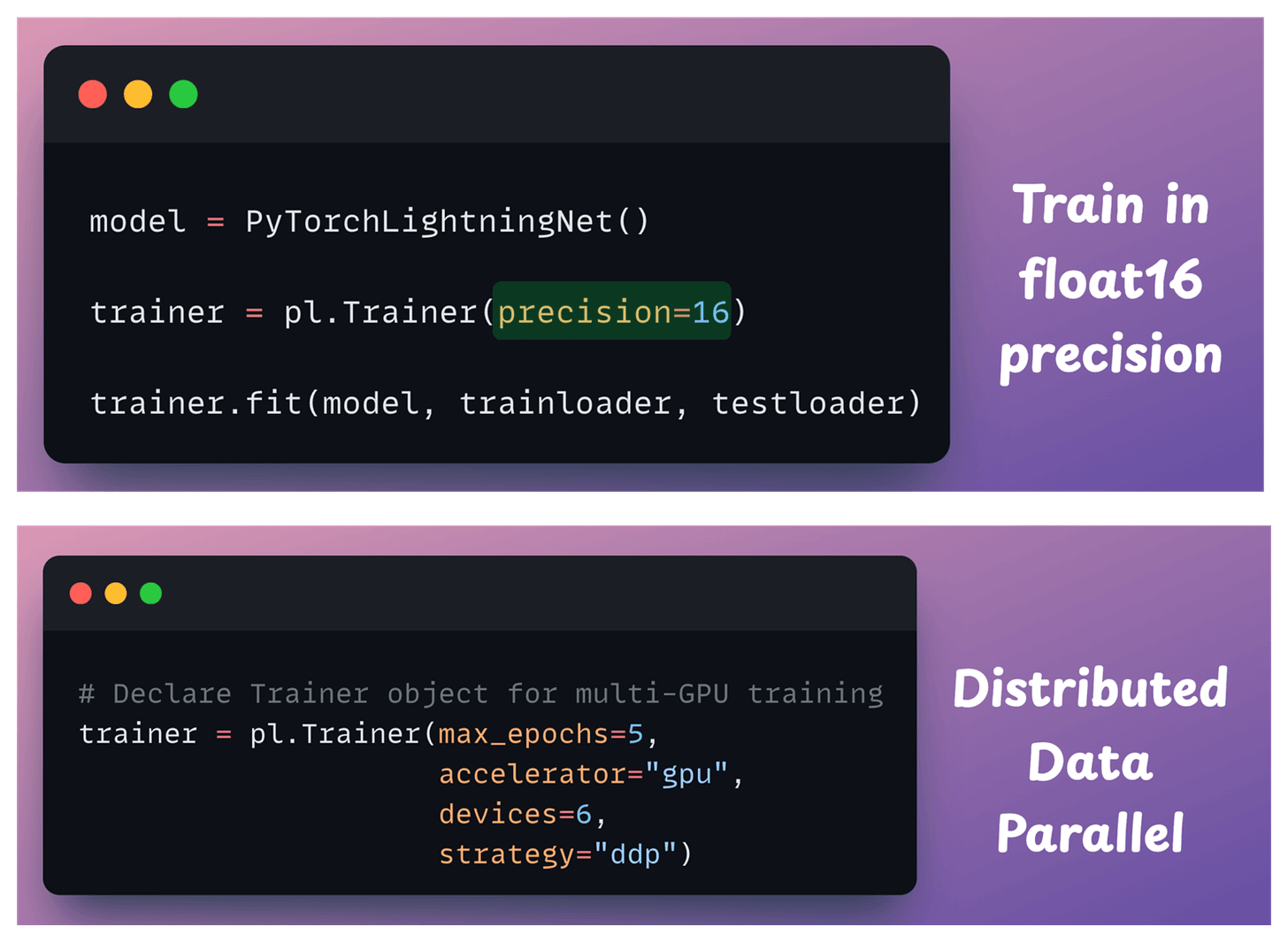
But it isn’t as flexible as PyTorch to write manual training loops and stuff.
Lately, I have been experimenting with Lightning Fabric, which brings together:
- The flexibility of PyTorch.
- Direct access to distributed training features like PyTorch Lightning does.
Today’s issue covers the 4 small changes you can make to your existing PyTorch code to easily scale it to the largest billion-parameter models/LLMs.
This is summarized in a single frame below:
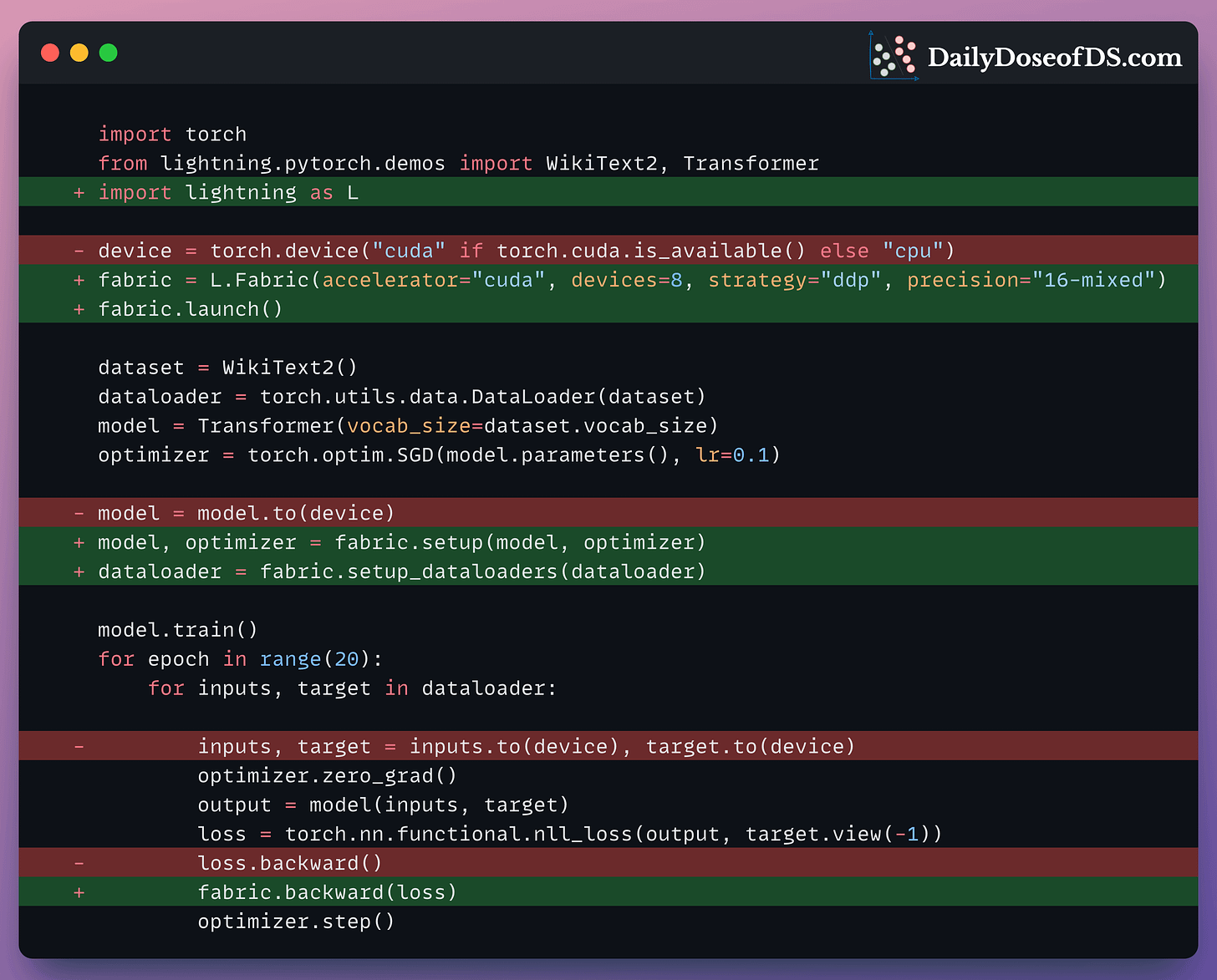
Let’s distill it!
Make these 4 changes
Begin by importing the lightning module (install using pip install lightning
), creating a Fabric
object and launching it.
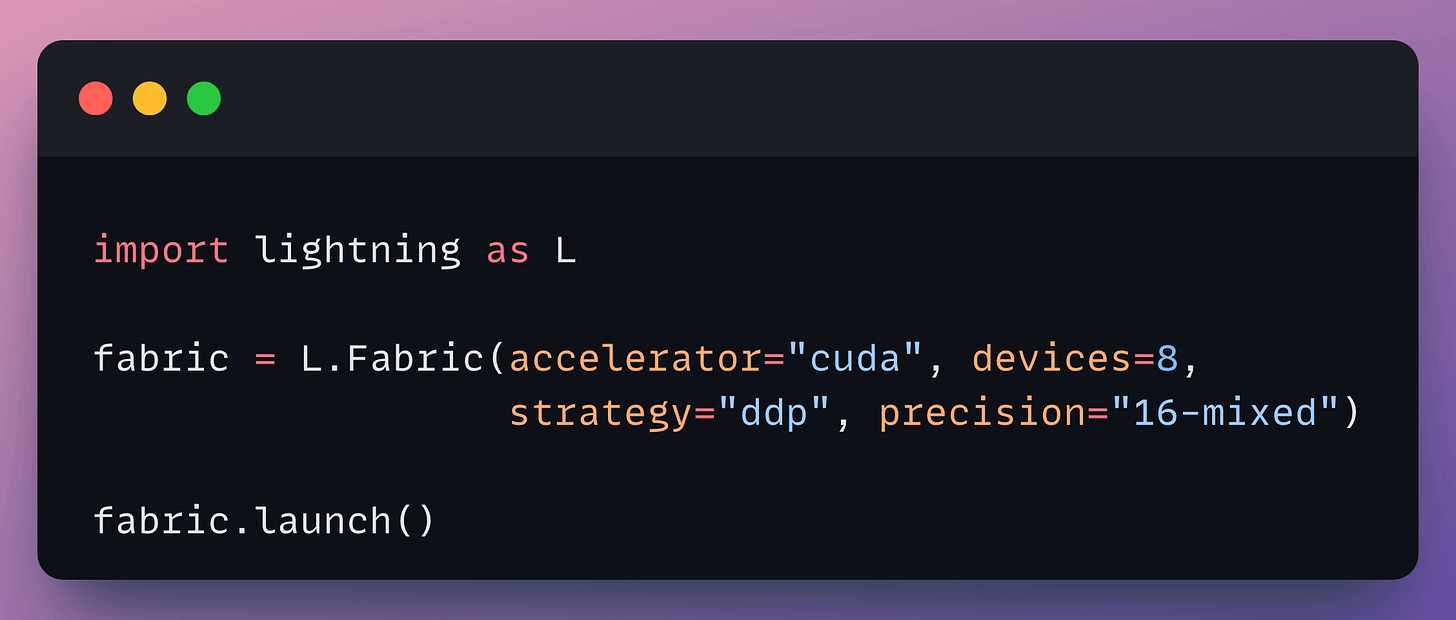
In my opinion, this Fabric
object is the most powerful aspect here since you can specify the training configuration and settings directly with parameters.
For instance, with a single line of code, you specify the number of devices and the parallelism strategy to use:
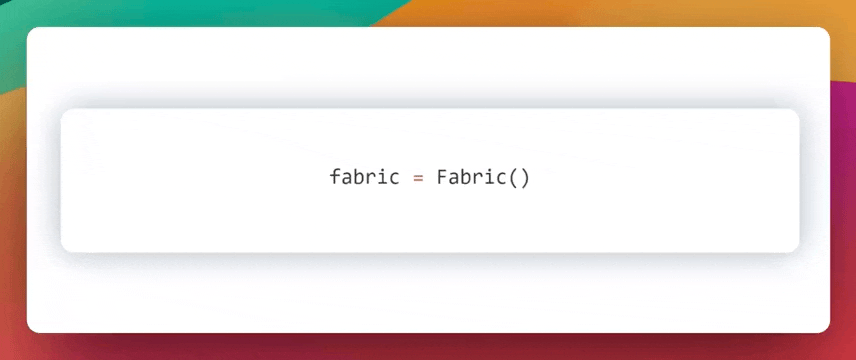
Moreover, it lets you take full advantage of the hardware on your system. It supports CPU, TPU, and GPU (NVIDIA, AMD, Apple Silicon).
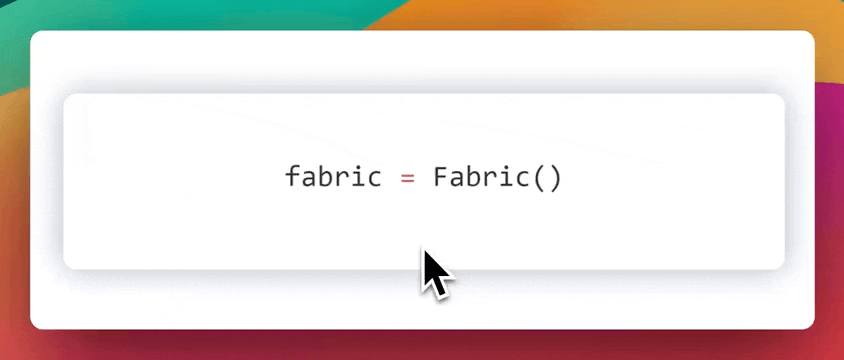
Moving on, configure the model, the optimizer, and the dataloader as follows:
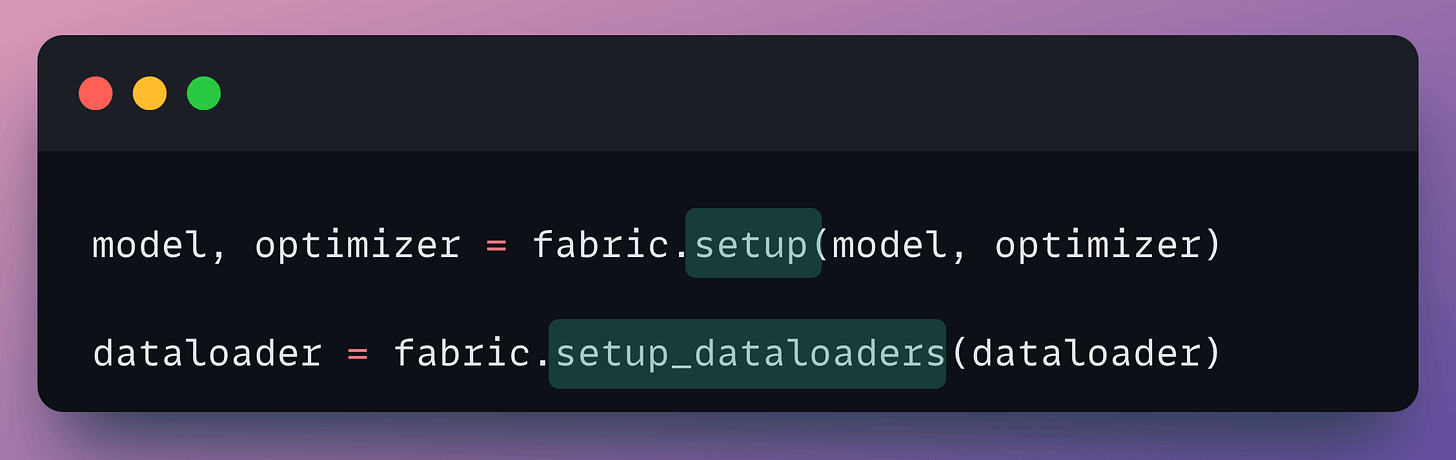
Next, remove all .to()
and .cuda()
calls since Fabric takes care of it automatically:
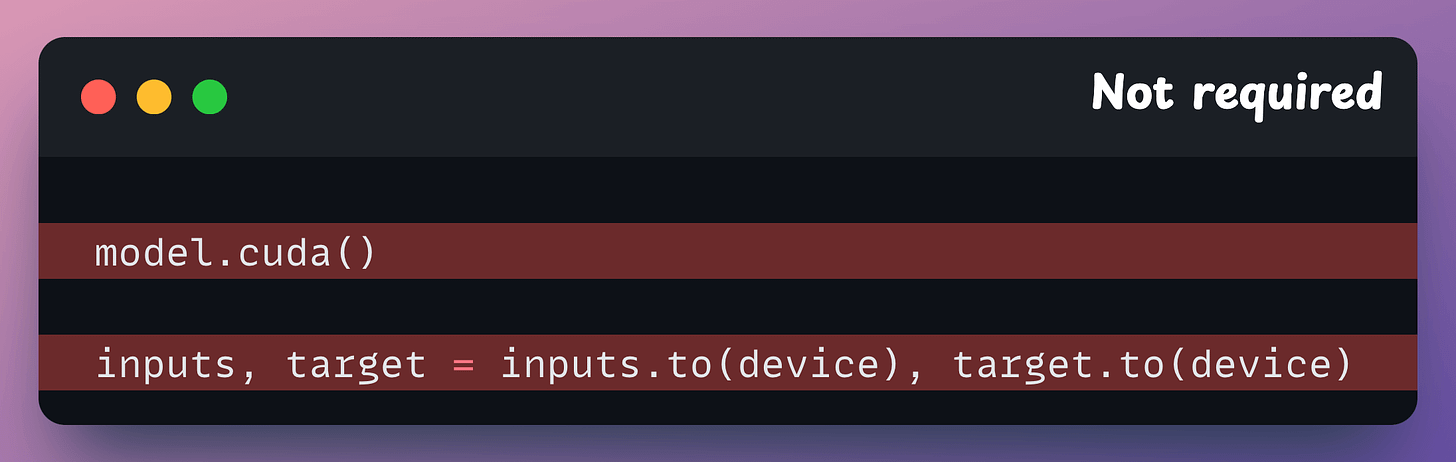
Finally, replace the loss.backward()
call by fabric.backward(loss)
call.
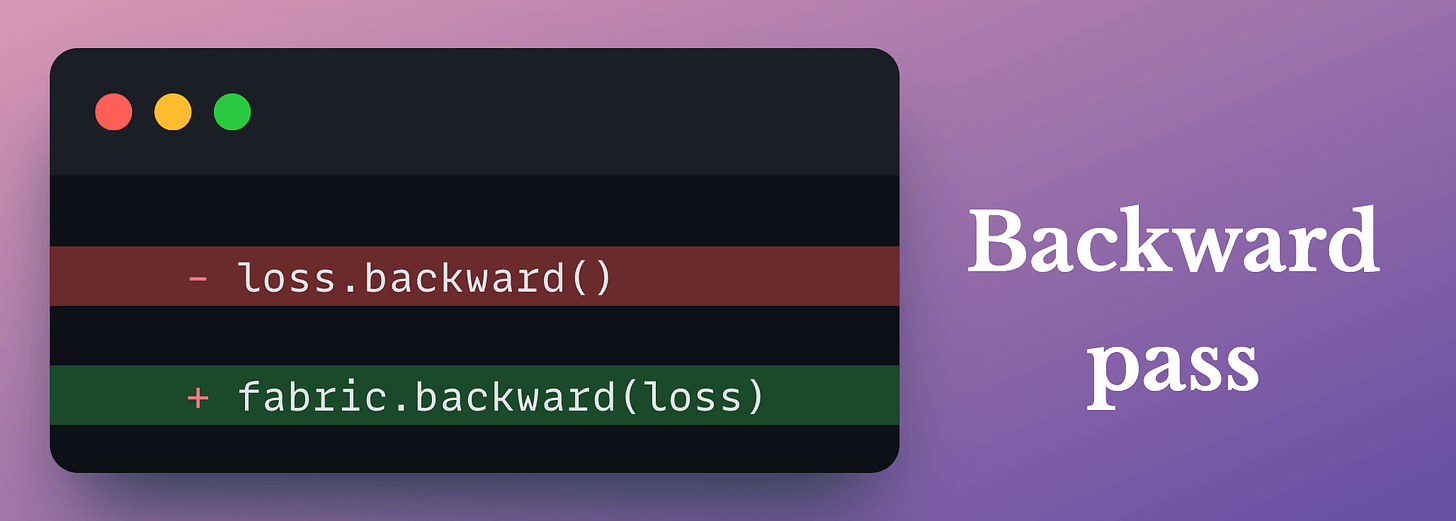
Done!
Now, you can train the model as you usually would, but with PyTorch Fabric, you have reduced the model scaling efforts.
That was simple, wasn’t it?
Here’s the documentation if you want to dive into more details about the usage: Lightning Fabric.
👉 Over to you: What are some issues with PyTorch?
Training OPTIMIZATION
Multi-GPU TRAINING (A Practical Guide)
If you look at job descriptions for Applied ML or ML engineer roles on LinkedIn, most of them demand skills like the ability to train models on large datasets:
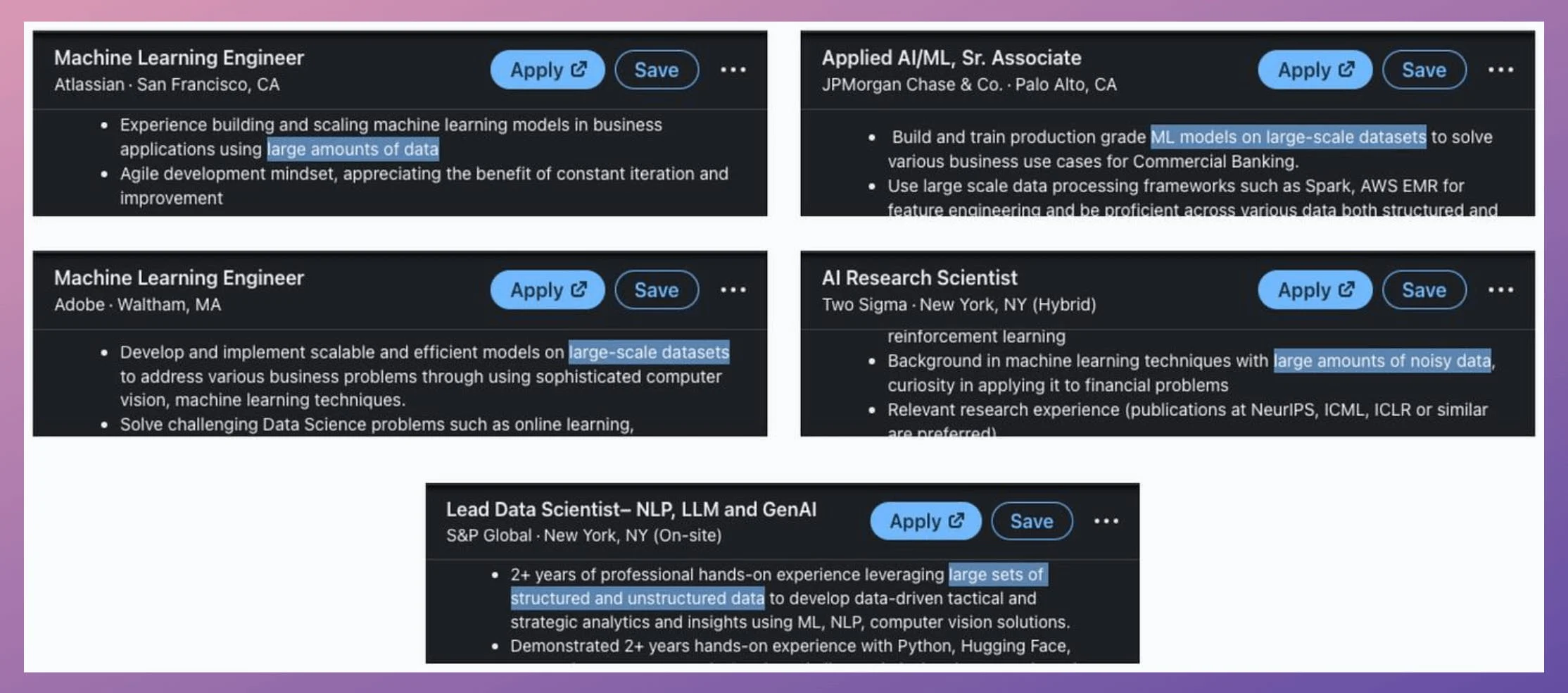
Of course, this is not something new or emerging.
But the reason they explicitly mention “large datasets” is quite simple to understand.
Businesses have more data than ever before.
Traditional single-node model training just doesn’t work because one cannot wait months to train a model.
​Distributed (or multi-GPU) training is one of the most essential ways to address this.
​Here, we covered the core technicalities behind multi-GPU training, how it works under the hood, and implementation details.
We also look at the key considerations for multi-GPU (or distributed) training, which, if not addressed appropriately, may lead to suboptimal performance or slow training.
CRASH COURSE (56 MINS)
Graph Neural Networks
- Google Maps uses graph ML for ETA prediction.
- Pinterest uses graph ML (PingSage) for recommendations.
- Netflix uses graph ML (SemanticGNN) for recommendations.
- Spotify uses graph ML (HGNNs) for audiobook recommendations.
- Uber Eats uses graph ML (a GraphSAGE variant) to suggest dishes, restaurants, etc.
The list could go on since almost every major tech company I know employs graph ML in some capacity.
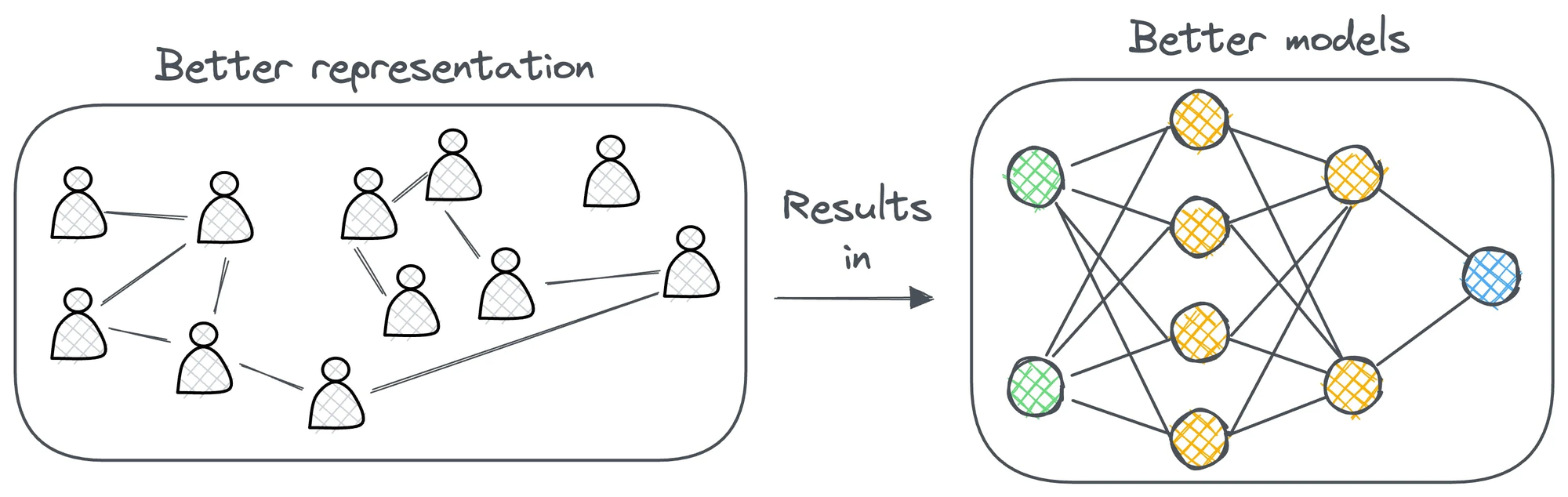
Becoming proficient in ​graph ML​ now seems to be far more critical than traditional deep learning to differentiate your profile and aim for these positions.
A significant proportion of our real-world data often exists (or can be represented) as graphs:
- Entities (nodes) are connected by relationships (edges).
- Connections carry significant meaning, which, if we knew how to model, can lead to much more robust models.
The field of ​graph neural networks (GNNs)​ intends to fill this gap by extending deep learning techniques to graph data.
Learn sophisticated graph architectures and how to train them on graph data in ​this crash course​​ →
THAT'S A WRAP
No-Fluff Industry ML resources to
Succeed in DS/ML roles
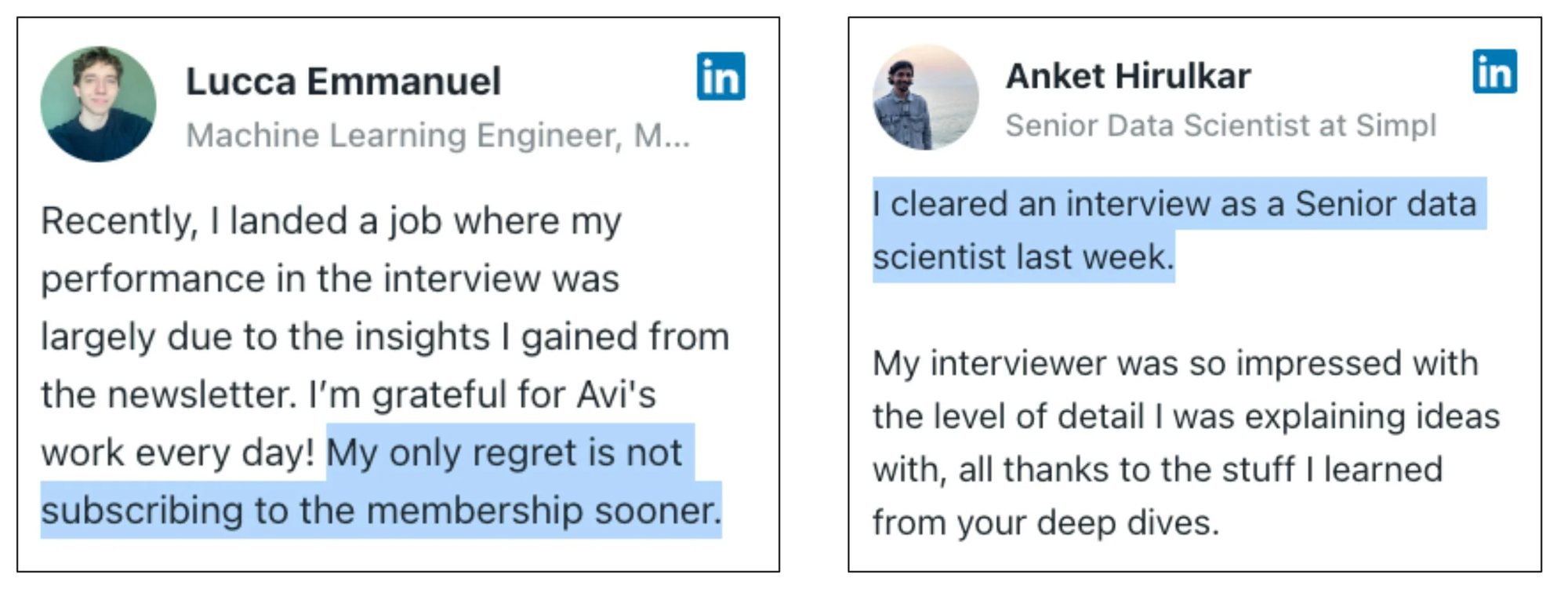
At the end of the day, all businesses care about impact. That’s it!
- Can you reduce costs?
- Drive revenue?
- Can you scale ML models?
- Predict trends before they happen?
We have discussed several other topics (with implementations) in the past that align with such topics.
Here are some of them:
- Learn sophisticated graph architectures and how to train them on graph data in this crash course.
- So many real-world NLP systems rely on pairwise context scoring. Learn scalable approaches here.
- Run large models on small devices using Quantization techniques.
- Learn how to generate prediction intervals or sets with strong statistical guarantees for increasing trust using Conformal Predictions.
- Learn how to identify causal relationships and answer business questions using causal inference in this crash course.
- Learn how to scale and implement ML model training in this practical guide.
- Learn 5 techniques with implementation to reliably test ML models in production.
- Learn how to build and implement privacy-first ML systems using Federated Learning.
- Learn 6 techniques with implementation to compress ML models.
All these resources will help you cultivate key skills that businesses and companies care about the most.
SPONSOR US
Advertise to 600k+ data professionals
Our newsletter puts your products and services directly in front of an audience that matters — thousands of leaders, senior data scientists, machine learning engineers, data analysts, etc., around the world.