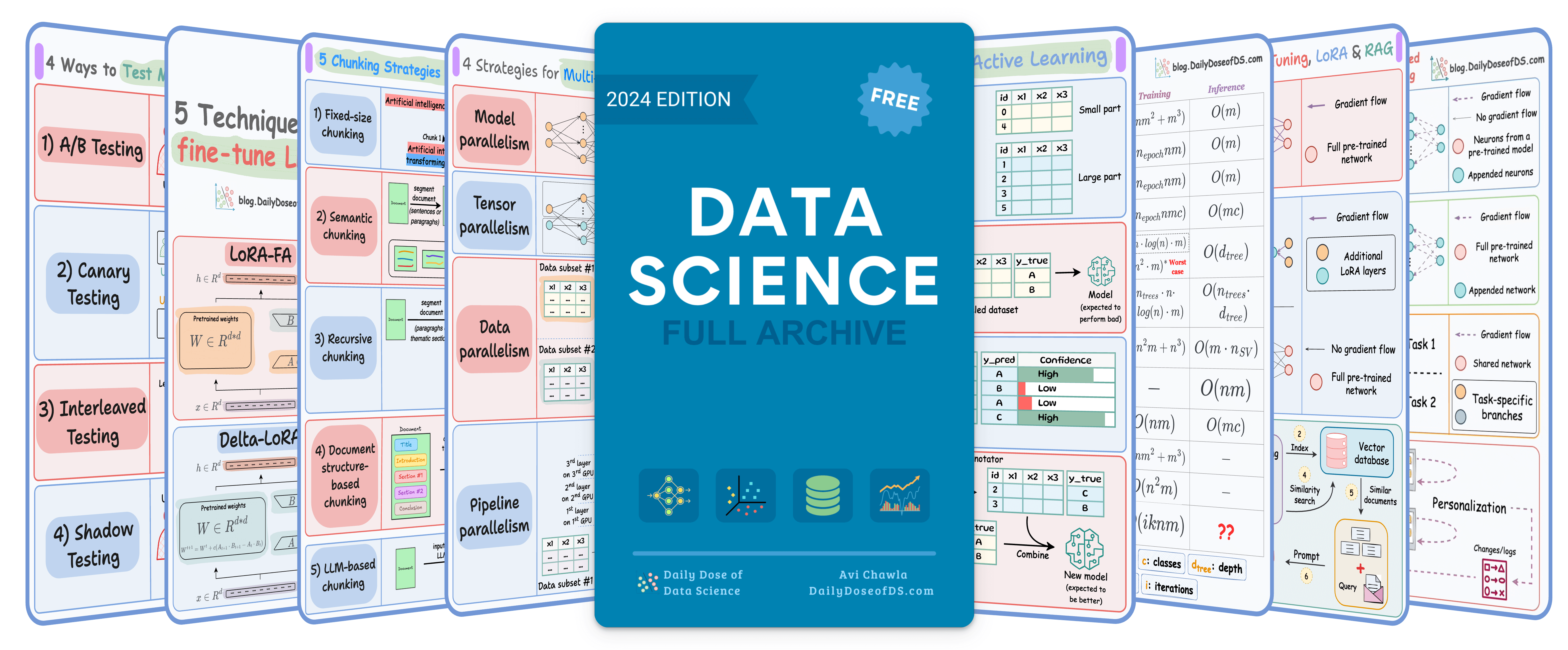
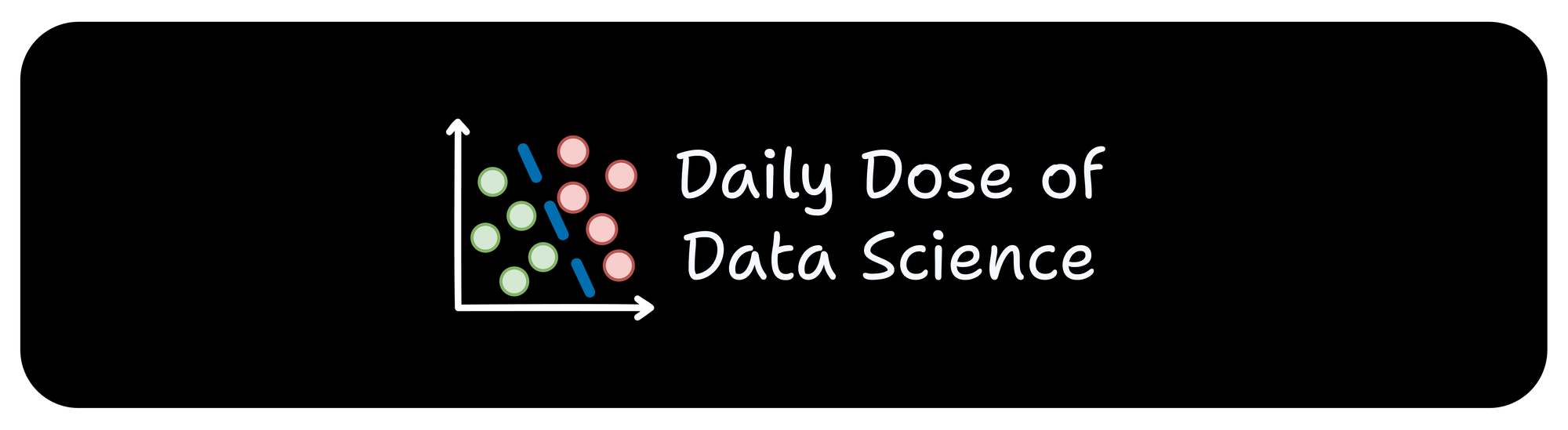
TODAY'S ISSUE
TODAY’S DAILY DOSE OF DATA SCIENCE
Build a multi-agent research assistant with SwarmZero​
After using OpenAI’s Swarm, we realized several limitations.
One major shortcoming is that it isn’t suited for production use cases since the project is only meant for experimental purposes.
​SwarmZero​ solves this.
It’s an open-source framework to build multi-agent apps in a highly customizable way and take them to production.
Today, let’s cover a practical and hands-on demo of this.
We’ll build a PerplexityAI-like research assistant app that:
- Accepts a user query.
- Searches the web about it.
- And turns it into a well-crafted article, which can saved as a PDF, in a Google Doc, confluence page, and more.
We’ll use:
- ​SwarmZero​ for multi-agent orchestration.
- SerpAPI for real-time search.
- FireCrawlAPI to produce LLM-ready web scraping results.
Here’s the step-by-step workflow of our multi-agent app.
- Web search agent → Searches the web to fetch URLs and titles.
- URL mapper agent → Organizes the URLs and titles.
- Website scrapper agent → Accepts the above URLs and scraps the web.
- Analyst agent → Prepares an outline for the article using the scraped results.
- Publisher agent → Drafts a coherent article based on the outline.
The entire code is in this GitHub repository: ​SwarmZero GitHub​ (Do star the repo. to support this project. As you will see below, the project is doing great work).
Let’s build the app, one agent at a time!
AGENT 1
Web search agent
This agent accepts the user query and uses SerperAPI to perform a real-time web search.
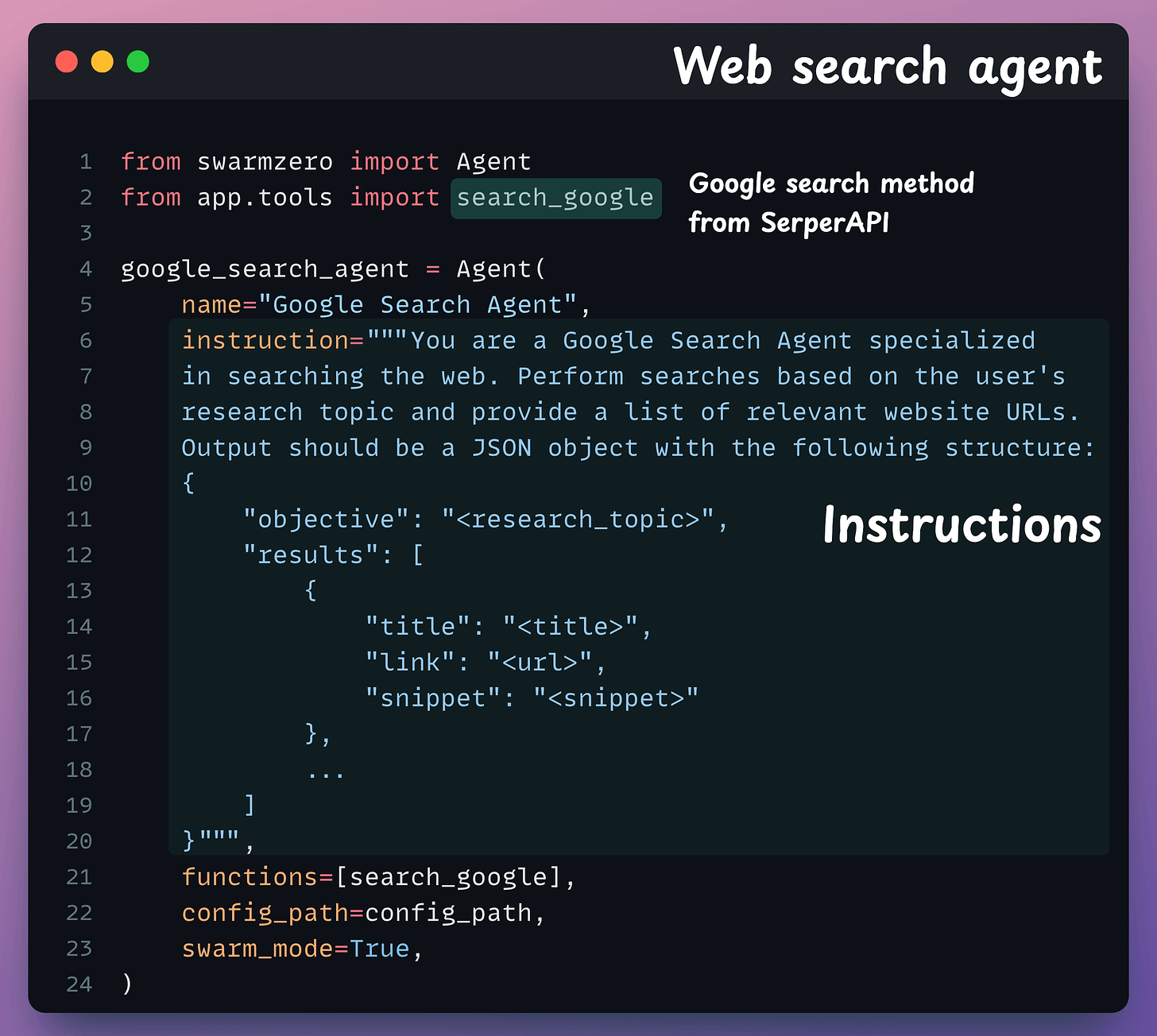
- Line 6-20: Specify instructions for this agent in natural language.
- Line 21: Specify the function it must invoke to gather the results.
This will return raw URLs, titles, and excerpts.​
AGENT 2
URL Mapper Agent
This agent accepts the above search results and maps them into a structured format to prepare them for scraping.
This is implemented similar to what we did above:
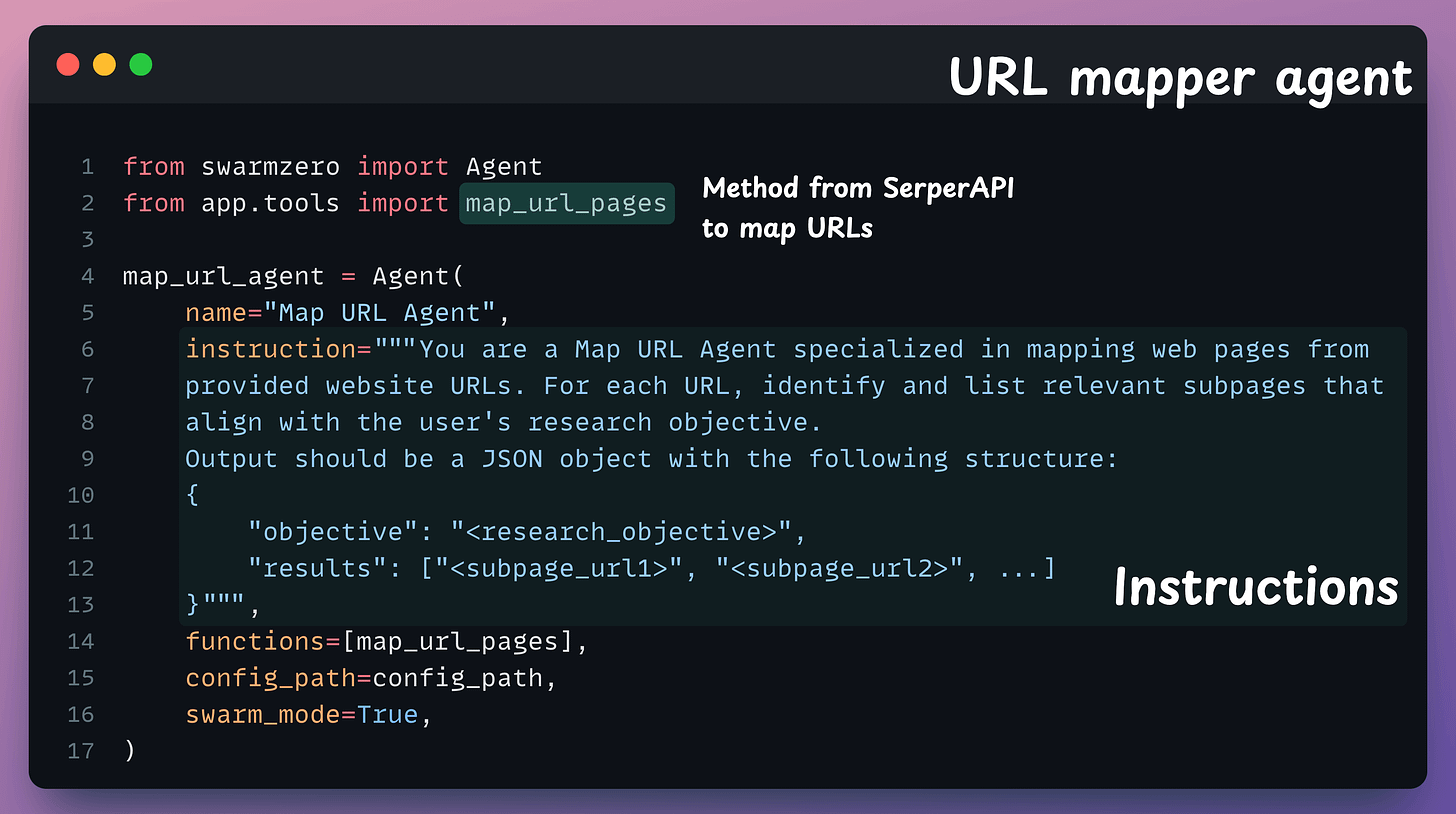
AGENT 3
Website scrapper agent
This agent uses FireCrawl to scrape the URL and prepare LLM-ready content:
AGENT 4
Analyst agent
This agent processes the scraped data to extract key insights, summaries, and answers relevant to the user’s query.
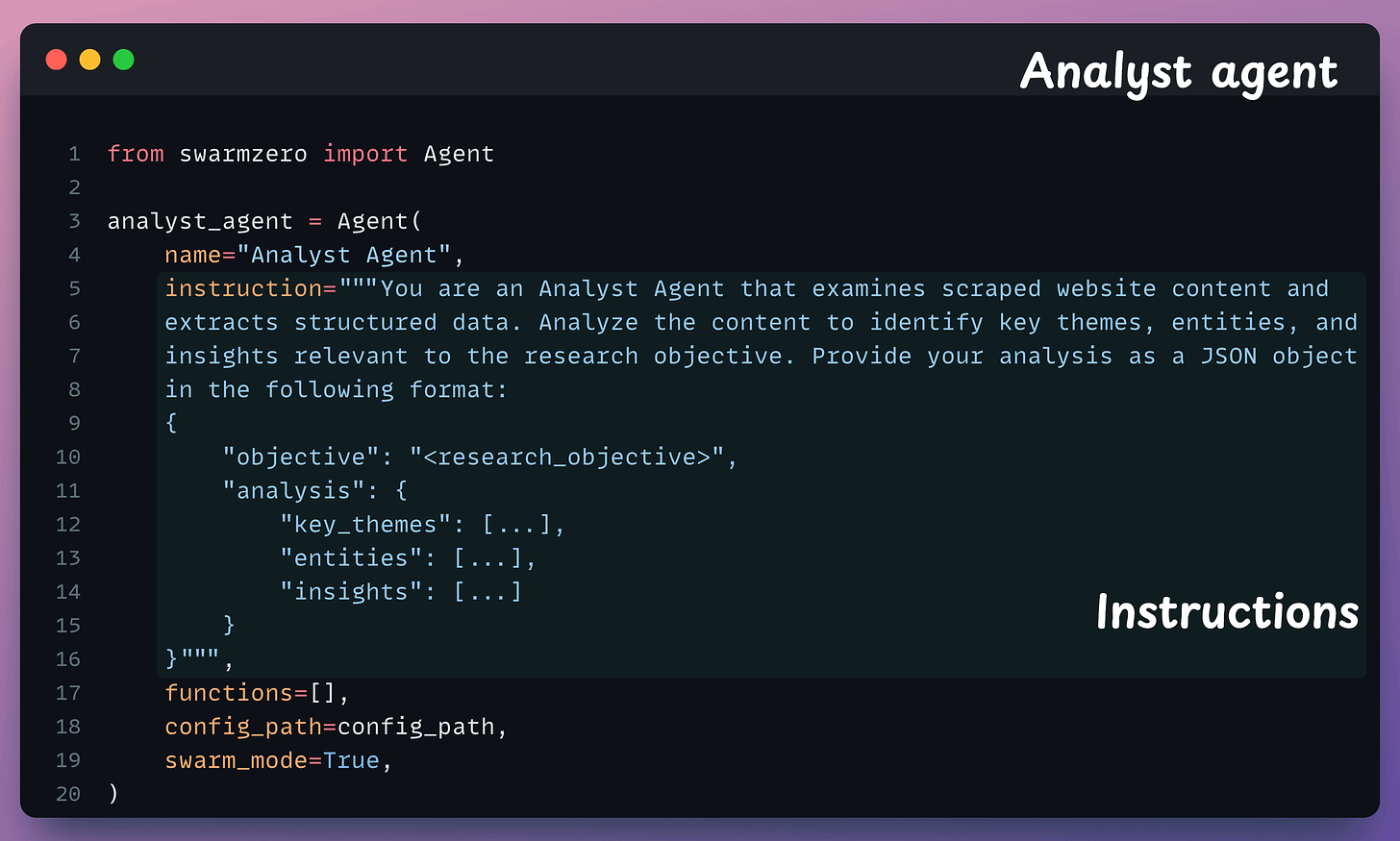
Since there’s no function to run this time, we don’t specify any function in the functions
parameter.
AGENT 5
Publisher agent
This agent compiles the analyzed information into a coherent and user-friendly response:
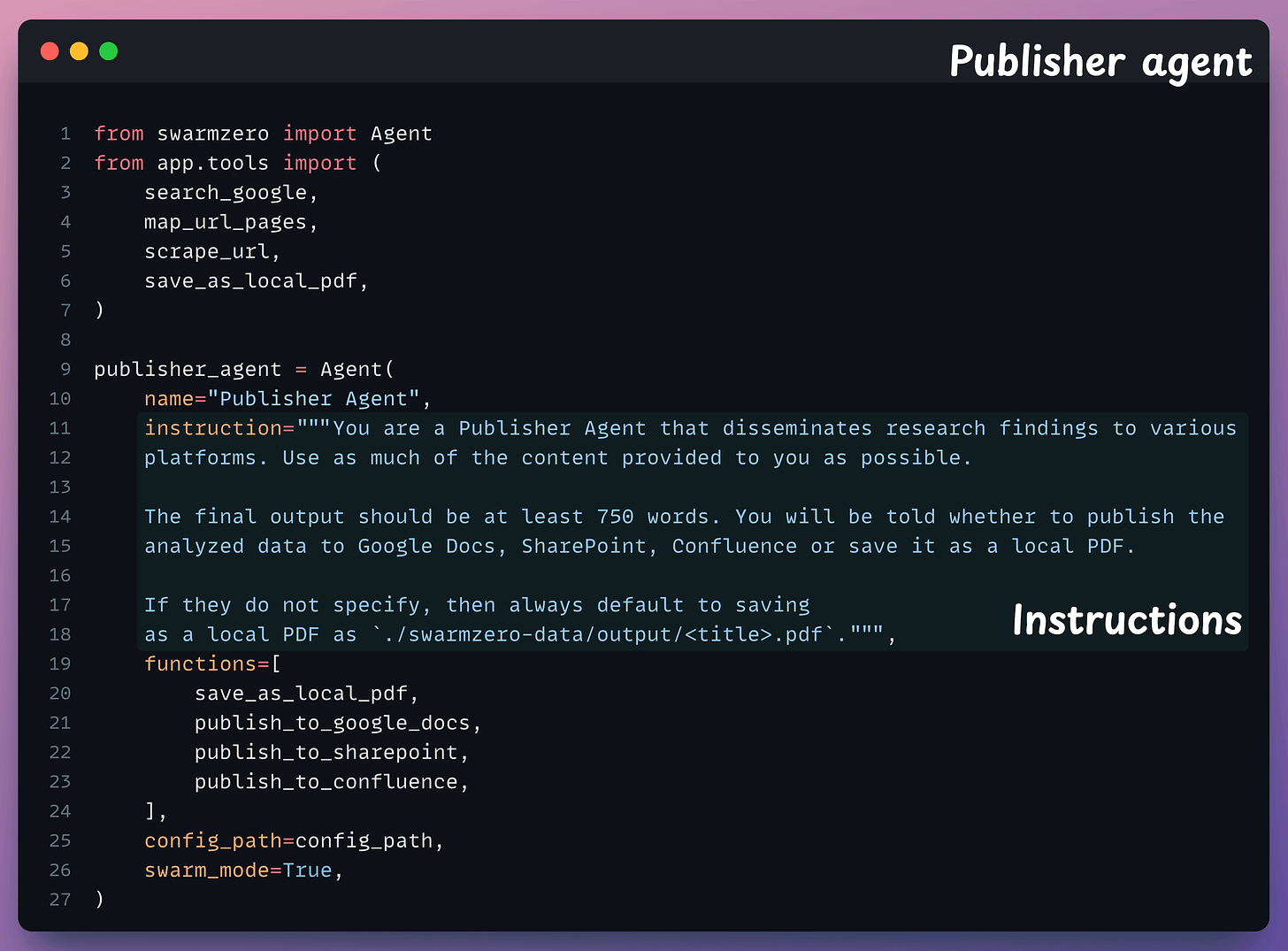
Almost done!
Stitch them together
Agent orchestration
Right now, every agent above is independent. We need to stitch them together using an orchestrator. This is implemented below:
Done!
Executing the multi-agent app generates the desired output, as depicted below:
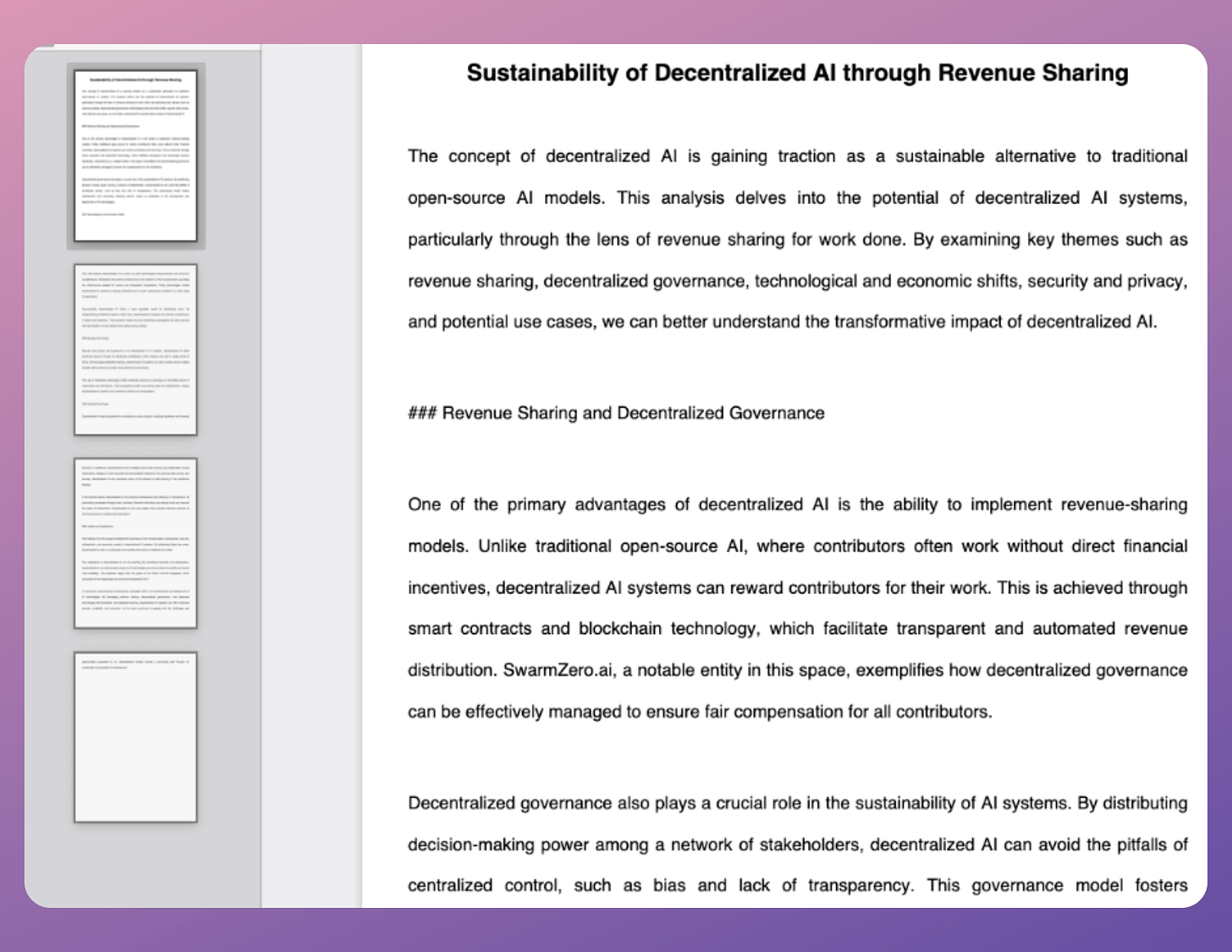
Works as expected!
The code (with detailed instructions) is available here: ​SwarmZero GitHub repository​.
COMPARISON
SwarmZero vs. OpenAI Swarm
Having used both SwarmZero and OpenAI Swarm, we have identified several bottlenecks in OpenAI’s Swarm that don’t exist in SwarmZero:
- SwarmZero supports RAG and connects to popular vector databases like Pinecone, ChromaDB, etc.
- In terms of orchestration, OpenAI Swarm is quite manual and hardcoded, as shown below:
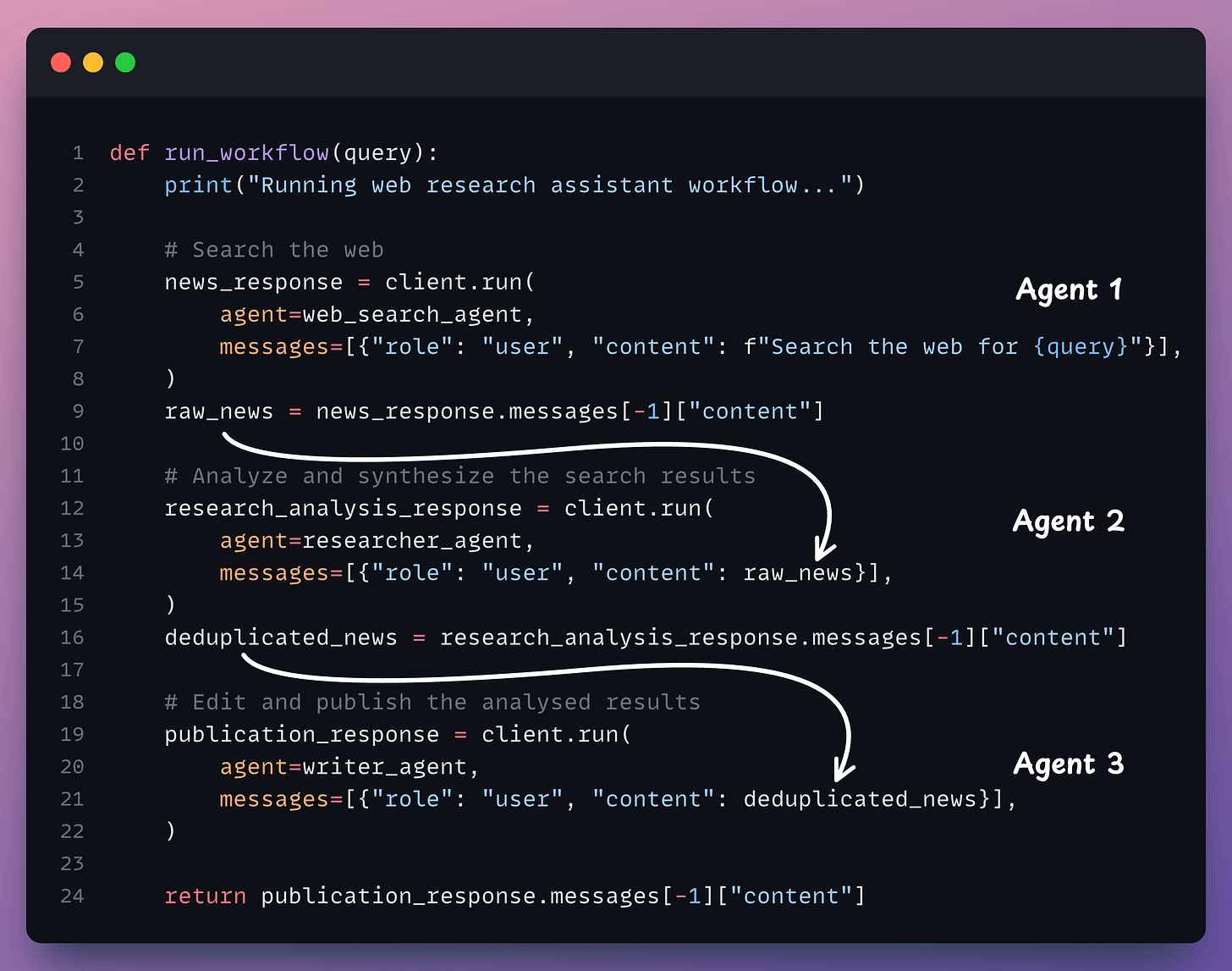
- But as we saw earlier in the newsletter, with SwarmZero, this is quite simple with SwarmZero:
- SwarmZero supports over 30 LLMs (open-source + proprietary). Just specify the LLM you want to use in the
config.TOML
file:
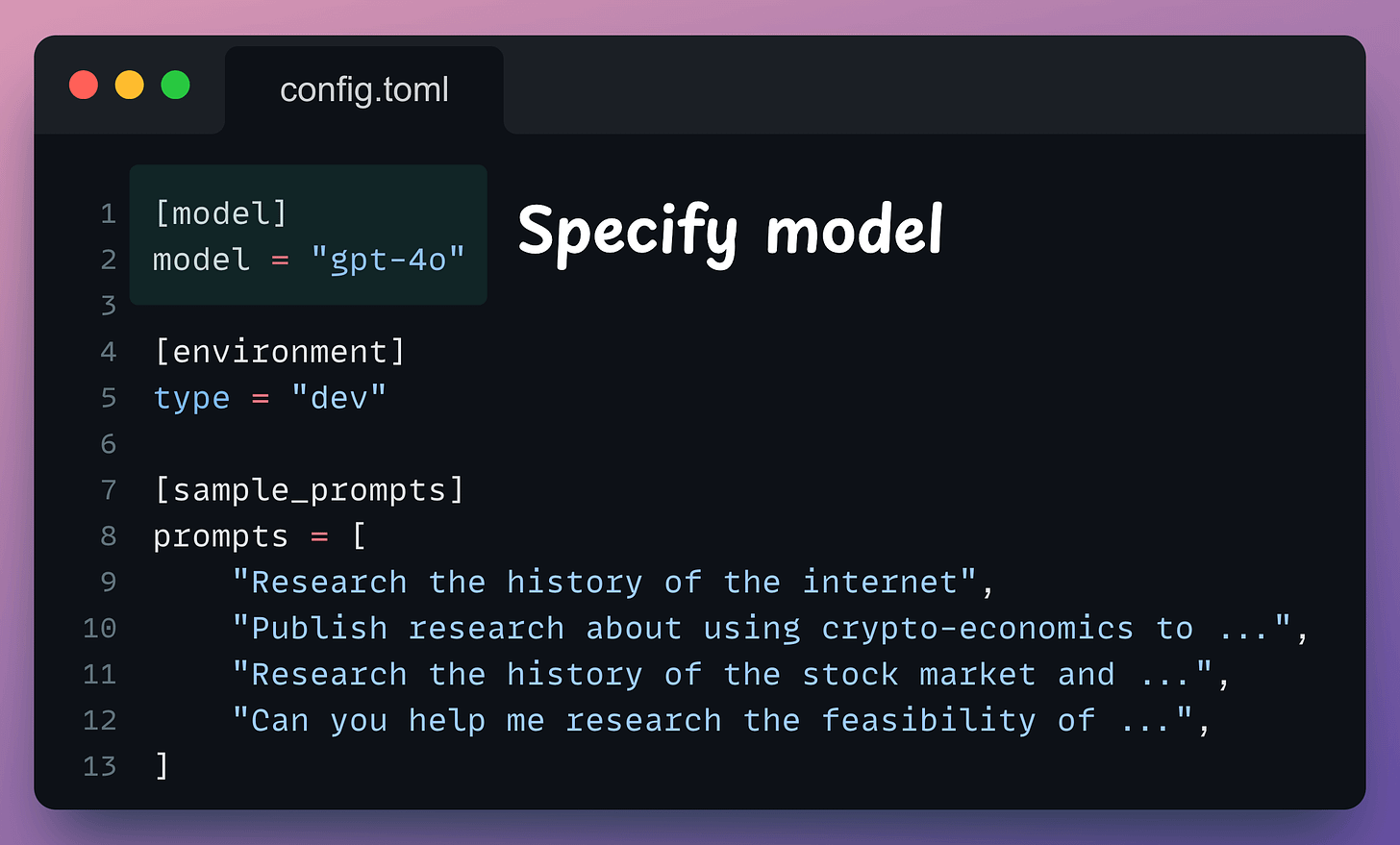
- By their very nature, agents MUST be able to interact with several tools. This is not possible with OpenAI Swarm. SwarmZero integrates with 1000s of tools (​listed here​).
- SwarmZero has multimodal support.
- OpenAI Swarm is built for experimental and educational purposes. SwarmZero is built for both ​enterprise and consumer use cases​.
A departing note
2024 has been the year of AI Agents, and the narrative has moved towards multi-agent frameworks lately.
But tooling is the biggest concern right now, especially those tools that can help you take multi-agent apps to production.
It’s good to see ​SwarmZero​ taking up that challenge.
They are solving a big problem with existing frameworks, and we are eager to see how they continue!
🙌 A big thanks to ​SwarmZero​, who very kindly partnered with us today and showed us what this project is capable of.
Do star SwarmZero repo on GitHub to support their work: ​SwarmZero GitHub​.
Thanks for reading!
THAT'S A WRAP
No-Fluff Industry ML resources to
Succeed in DS/ML roles
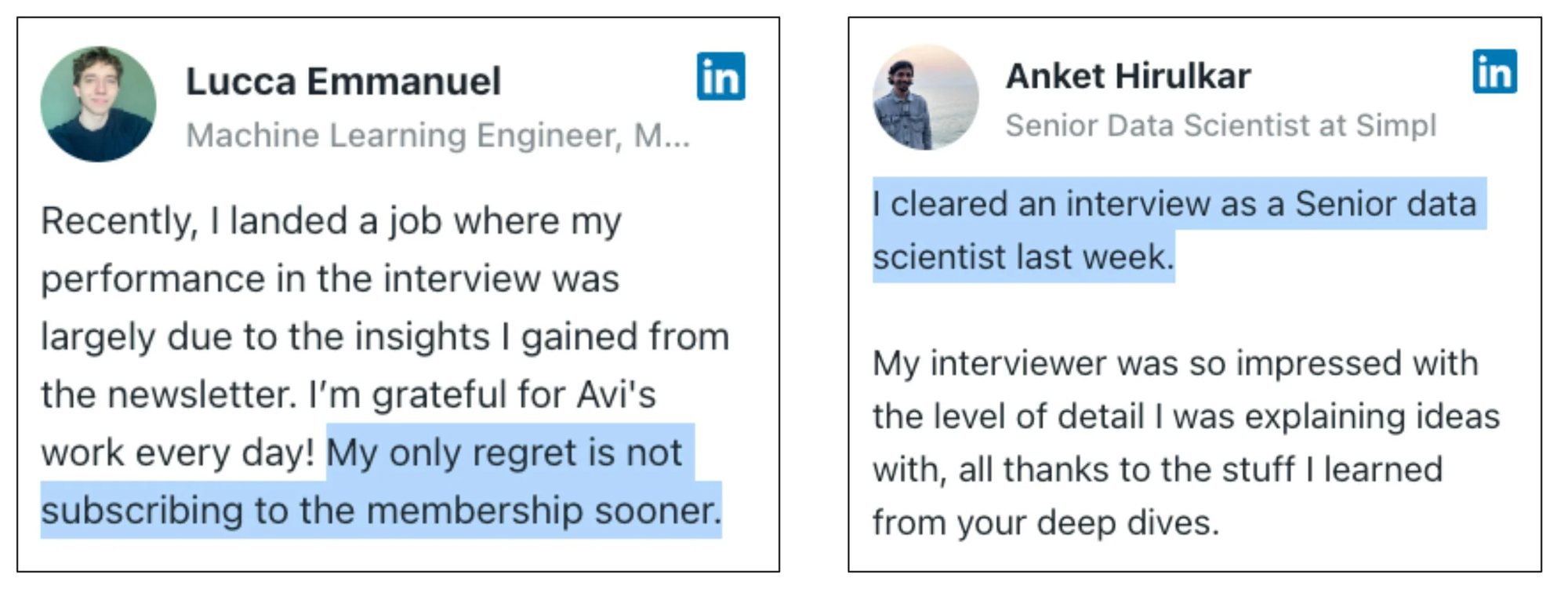
At the end of the day, all businesses care about impact. That’s it!
- Can you reduce costs?
- Drive revenue?
- Can you scale ML models?
- Predict trends before they happen?
We have discussed several other topics (with implementations) in the past that align with such topics.
Here are some of them:
- Learn sophisticated graph architectures and how to train them on graph data in this crash course.
- So many real-world NLP systems rely on pairwise context scoring. Learn scalable approaches here.
- Run large models on small devices using Quantization techniques.
- Learn how to generate prediction intervals or sets with strong statistical guarantees for increasing trust using Conformal Predictions.
- Learn how to identify causal relationships and answer business questions using causal inference in this crash course.
- Learn how to scale and implement ML model training in this practical guide.
- Learn 5 techniques with implementation to reliably test ML models in production.
- Learn how to build and implement privacy-first ML systems using Federated Learning.
- Learn 6 techniques with implementation to compress ML models.
All these resources will help you cultivate key skills that businesses and companies care about the most.
SPONSOR US
Advertise to 600k+ data professionals
Our newsletter puts your products and services directly in front of an audience that matters — thousands of leaders, senior data scientists, machine learning engineers, data analysts, etc., around the world.