A Crash Course on Model Calibration
Modern neural networks being trained today are highly misleading.
They appear to be heavily overconfident in their predictions.
For instance, if a model predicts an event with a 70% probability, then ideally, out of 100 such predictions, approximately 70 should result in the event occurring.
However, many experiments have revealed that modern neural networks appear to be losing this ability.
For instance, consider the following image, which compares two models:
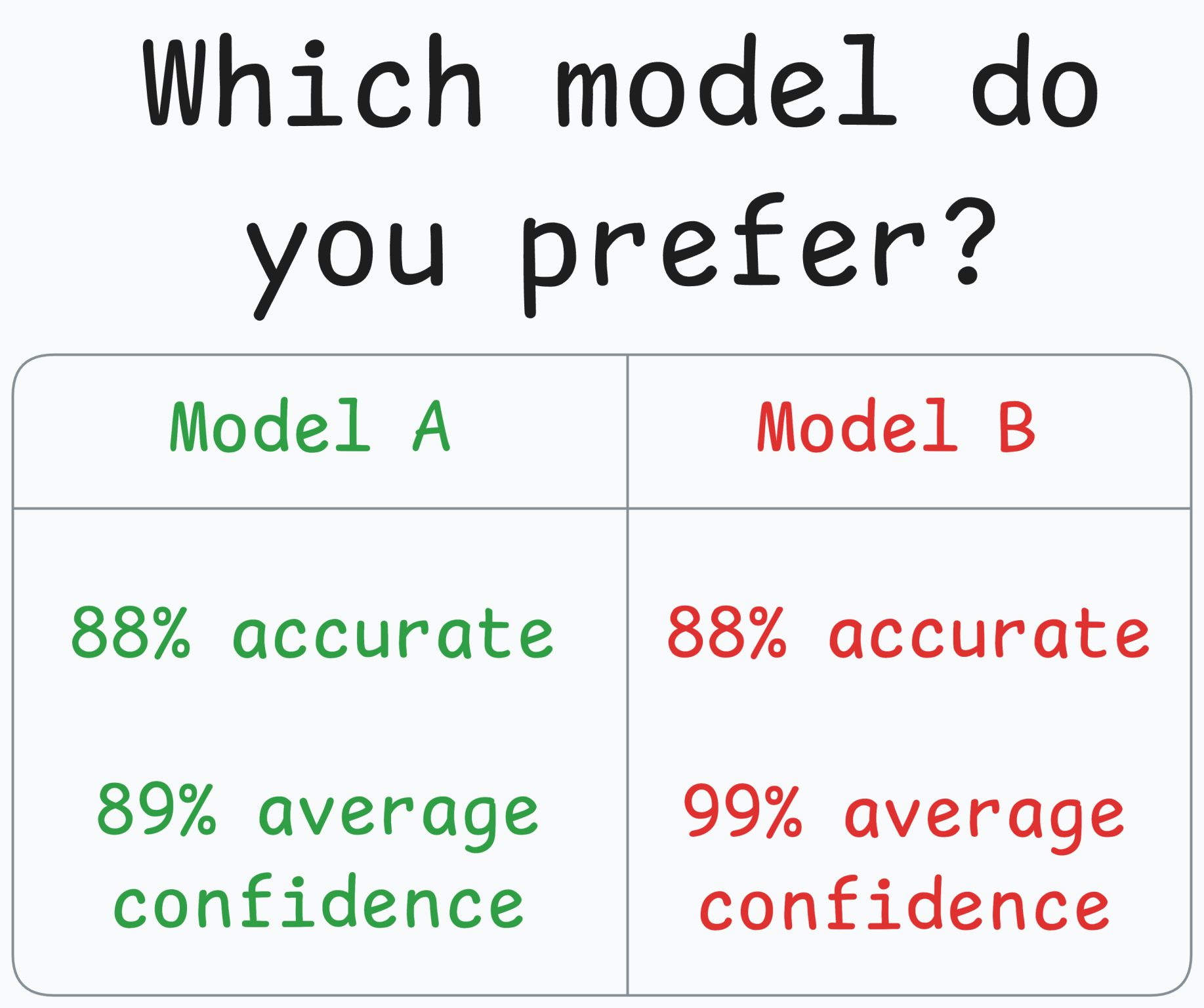
The above image indicates that even though both models are equally accurate:
- Model A produces an average confidence that aligns with its accuracy.
- However, Model B thinks it's 99% confident in its predictions, but in reality, it only turns out to be 88% accurate.
Calibration solves this.
A model is calibrated if the predicted probabilities align with the actual outcomes.
Handling this is important because the model will be used in decision-making.
In fact, an overly confident but not equally accurate model can be fatal.
To exemplify, say a government hospital wants to conduct an expensive medical test on patients.
To ensure that the govt. funding is used optimally, a reliable probability estimate can help the doctors make this decision.
If the model isn't calibrated, it will produce overly confident predictions.
This two-part crash covers model calibration in a beginner-friendly manner with implementations
In this two-part crash course, we:
- dive into the details of model calibration
- understand why it is a problem
- discuss why modern models are miscalibrating more
- learn techniques to determine miscalibration and their limitations.
- learn techniques to address miscalibration, with implementations.
- and more.
There has been a rising concern in the industry about ensuring that our machine learning models communicate their confidence effectively. Thus, being able to detect miscalibration and fix is a super skill one can possess.
Assuming you aspire to make valuable contributions to your data science job, this series will be super helpful in cultivating a diversified skill set.