Introduction
In the previous part of this crash course, we explored the ReAct pattern, a dynamic approach where an AI agent interleaves reasoning and acting steps to solve a problem.
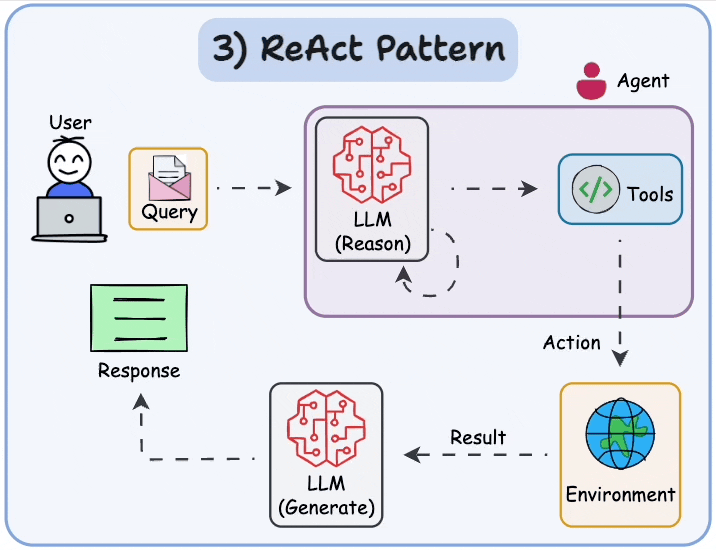
The ReAct agent thinks step-by-step (producing Thoughts) and takes actions (using tools) in a loop, adjusting its strategy as new information comes in.
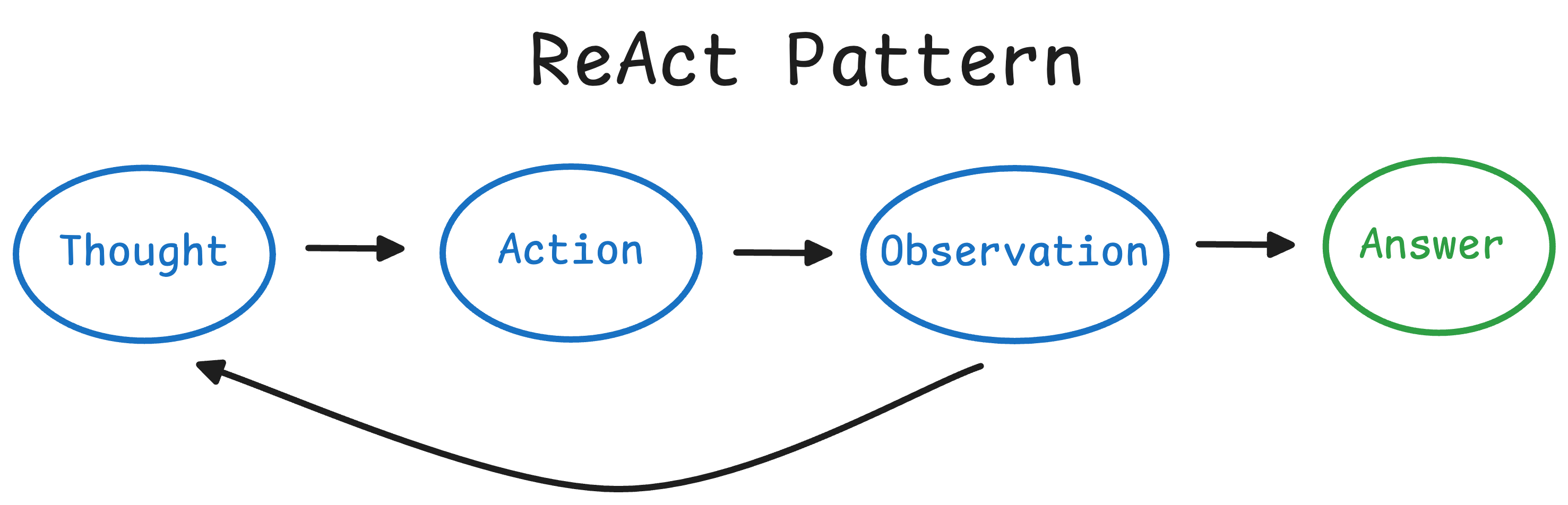
This reactive approach (Reasoning + Acting) enables an agent to adapt on the fly, combining chain-of-thought reasoning with external tool use to handle complex queries.
However, ReAct isn’t the only way to organize an agent’s thinking. Another powerful approach is the Planning pattern, which is the focus of this part.
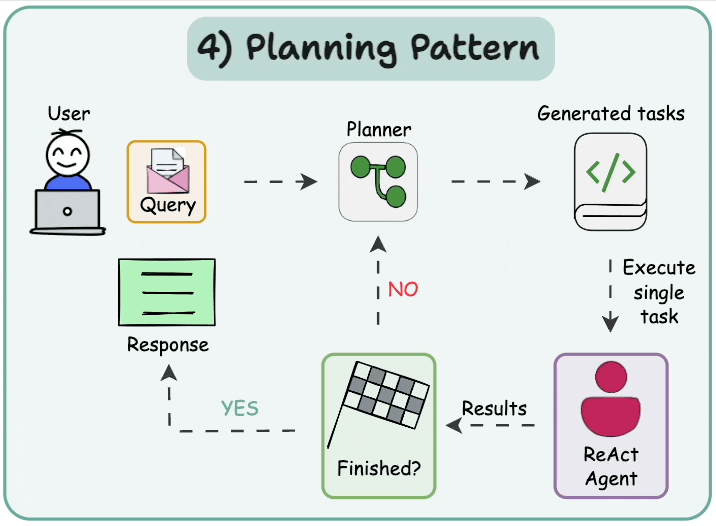
The Planning pattern has the agent plan out a solution strategy before executing any actions.
Instead of diving directly into step-by-step reasoning and tool use as ReAct does, a Planning agent will first take a step back and formulate a high-level plan or “roadmap” for the task.
Only after this plan is laid out does the agent proceed to carry out each step in the plan (using tools or other means), and finally, it produces the answer.
This approach enhances an LLM agent’s ability to handle complex tasks and decisions by first laying out a plan and then acting on it.
In this article, we’ll demystify that process by building a Planning agent from scratch using only Python and an LLM.
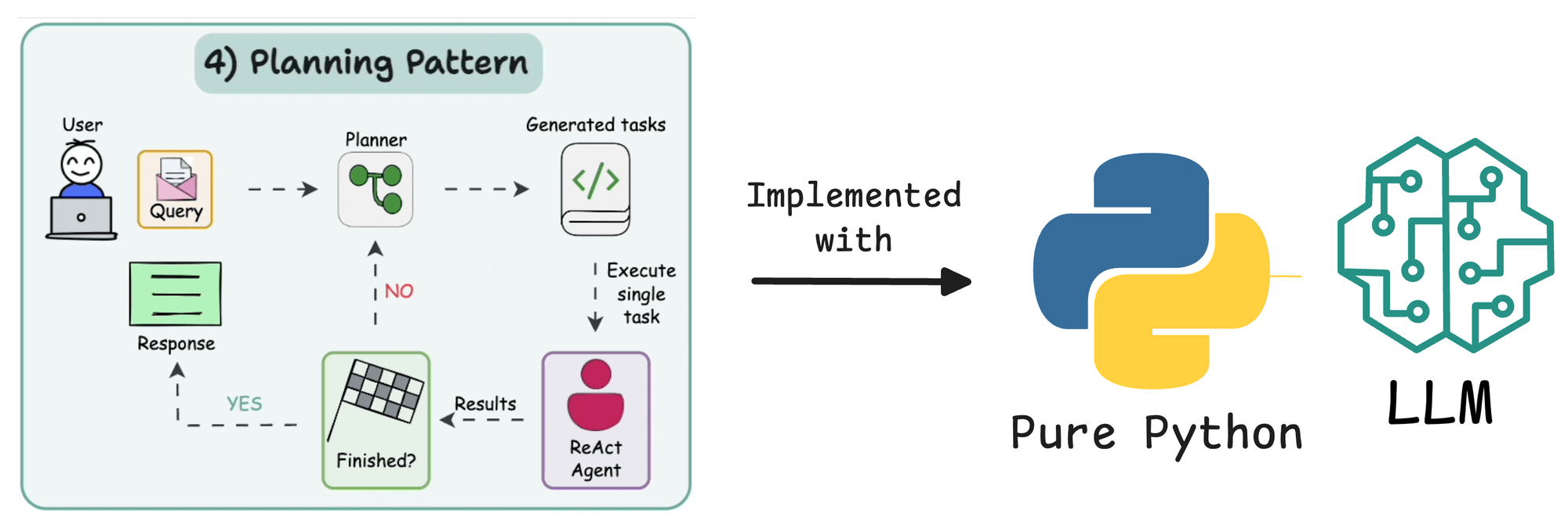
By doing so, we gain full control over the agent’s behavior, making it easier to optimize and troubleshoot.
We’ll use OpenAI, but if you prefer to do it with Ollama locally, an open-source tool for running LLMs locally, with a model like Llama3 to power the agent, you can do that as well.
Along the way, we’ll explain the Planning pattern, design an agent loop that interleaves planning and tool usage, and implement multiple tools that the agent can invoke.
Moreover, we’ll discuss why planning matters in AI agents, how the Planning pattern works in practice, what recent research says about its benefits (and challenges), and how it compares to other patterns like ReAct. By the end, you’ll understand when and how to use Planning in your own AI agent applications.
The goal is to help you understand both the theory and implementation of the Planning pattern in AI Agents.
By the end, you’ll have a working agent and a clear picture of how frameworks like CrewAI leverage Planning internally.
Let's begin!
Why planning?
Why do we need an explicit planning phase for an AI agent?
The short answer is that some problems are too complex to tackle one step at a time without a global strategy.
The ReAct paradigm excels at reactive decision-making. The agent looks at the current state, decides on one action, executes it, then observes the result and repeats.
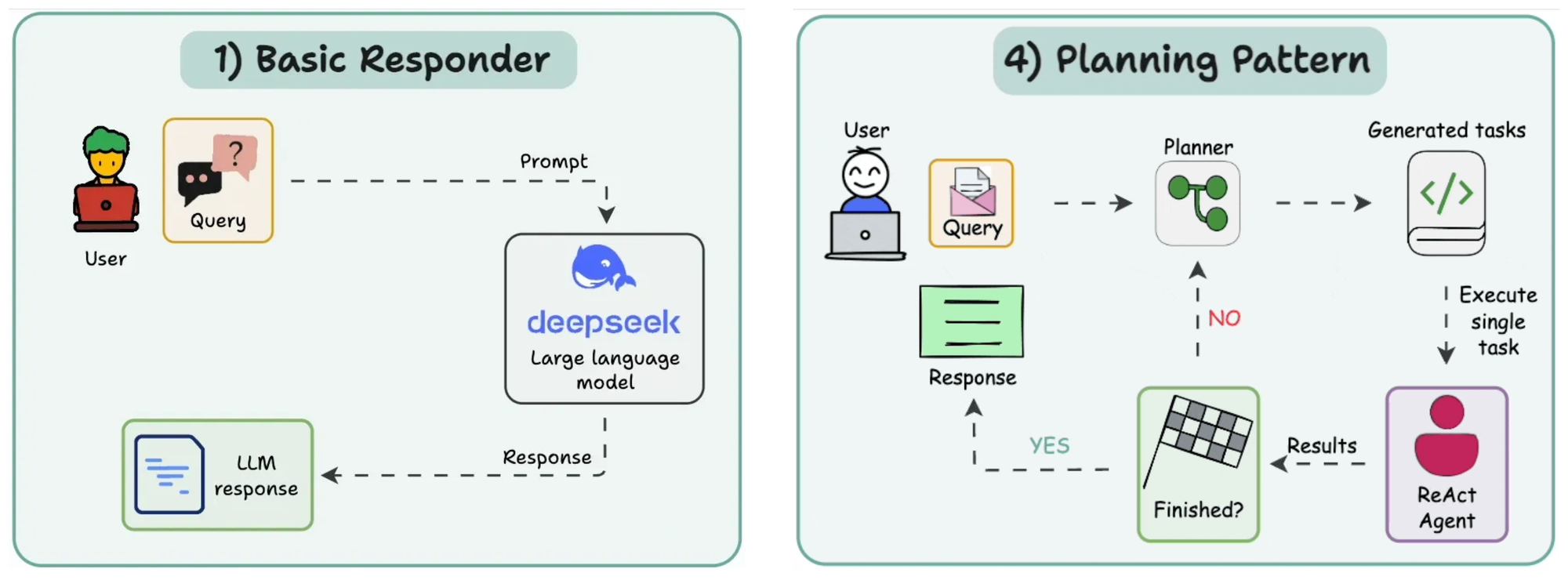
This works well for straightforward or highly uncertain tasks, but it can fall short for multi-step problems that benefit from foresight.
Consider an intuitive example: finding a pen versus making a cup of coffee.
If you ask an agent to simply get a pen from your desk, a reactive approach is fine. It can try one location, see if the pen is there, and if not, try the next.
In contrast, if you ask for a flat white coffee, the agent must handle several sub-tasks (boil water, grind beans, froth milk, etc.) and possibly adjust the plan as conditions change.
So put it into perspective, if ReAct is more suitable for tasks like getting a pen from the desk, then Plan & Solve is better suited for tasks like making a cup of flat white.
You need to plan, and the plan might change during the process (for example, if you open the fridge and find no milk, you would add ‘buy milk’ as a step.
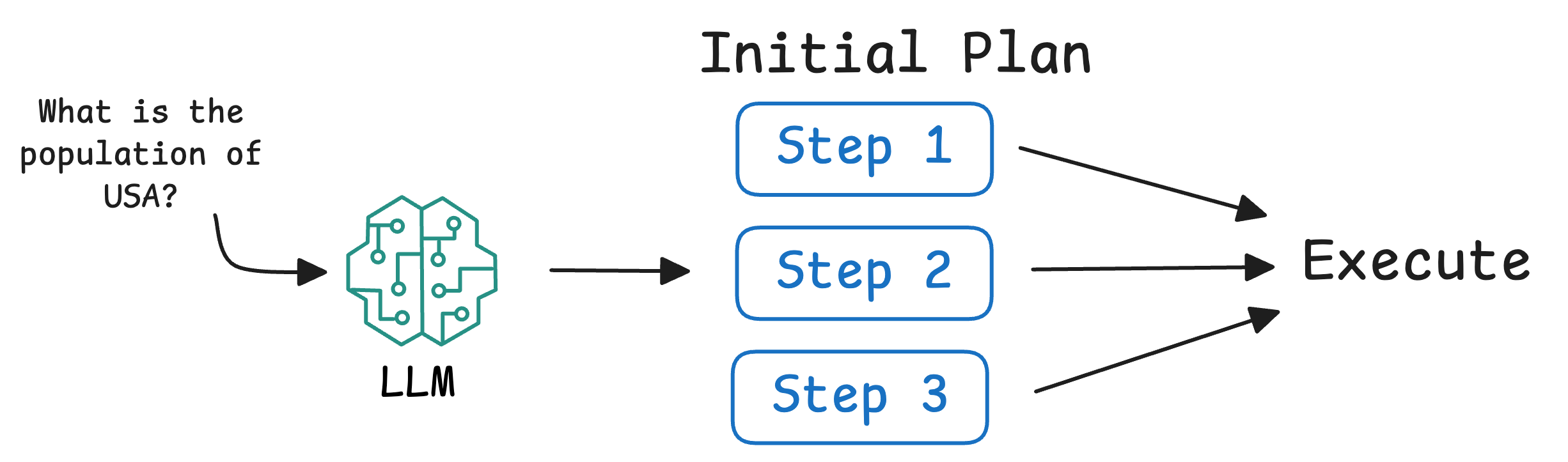
In other words, the more elaborate the task, the more an agent needs a game plan, much like a human would outline an approach before tackling a big project.
For instance, think of how you might tackle a complex question yourself: often you’ll outline a plan or break the problem into sub-tasks before doing any detailed work.
By subdividing the task and outlining objectives, the agent gains a global view of the problemby subdividing the task and outlining objectives.
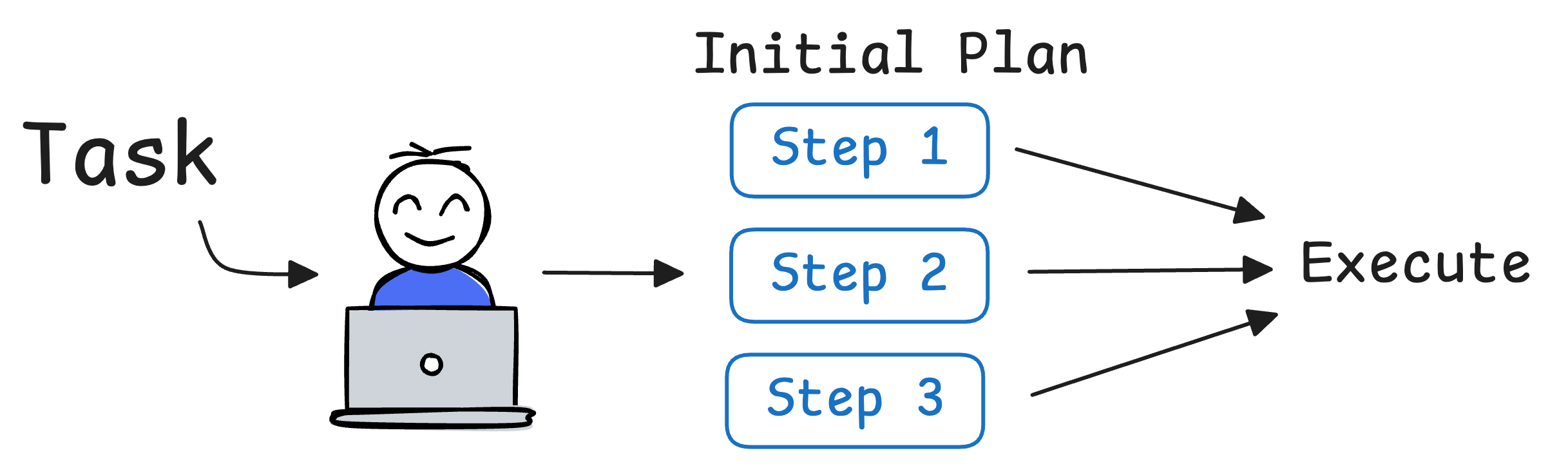
This can make its approach more strategic and efficient.
The Planning pattern is especially useful when the problem involves multiple distinct steps or pieces of information that need to be gathered and combined.
In such cases, a ReAct agent might still eventually find the answer, but it could meander or repeat work along the way because it decides actions one at a time.
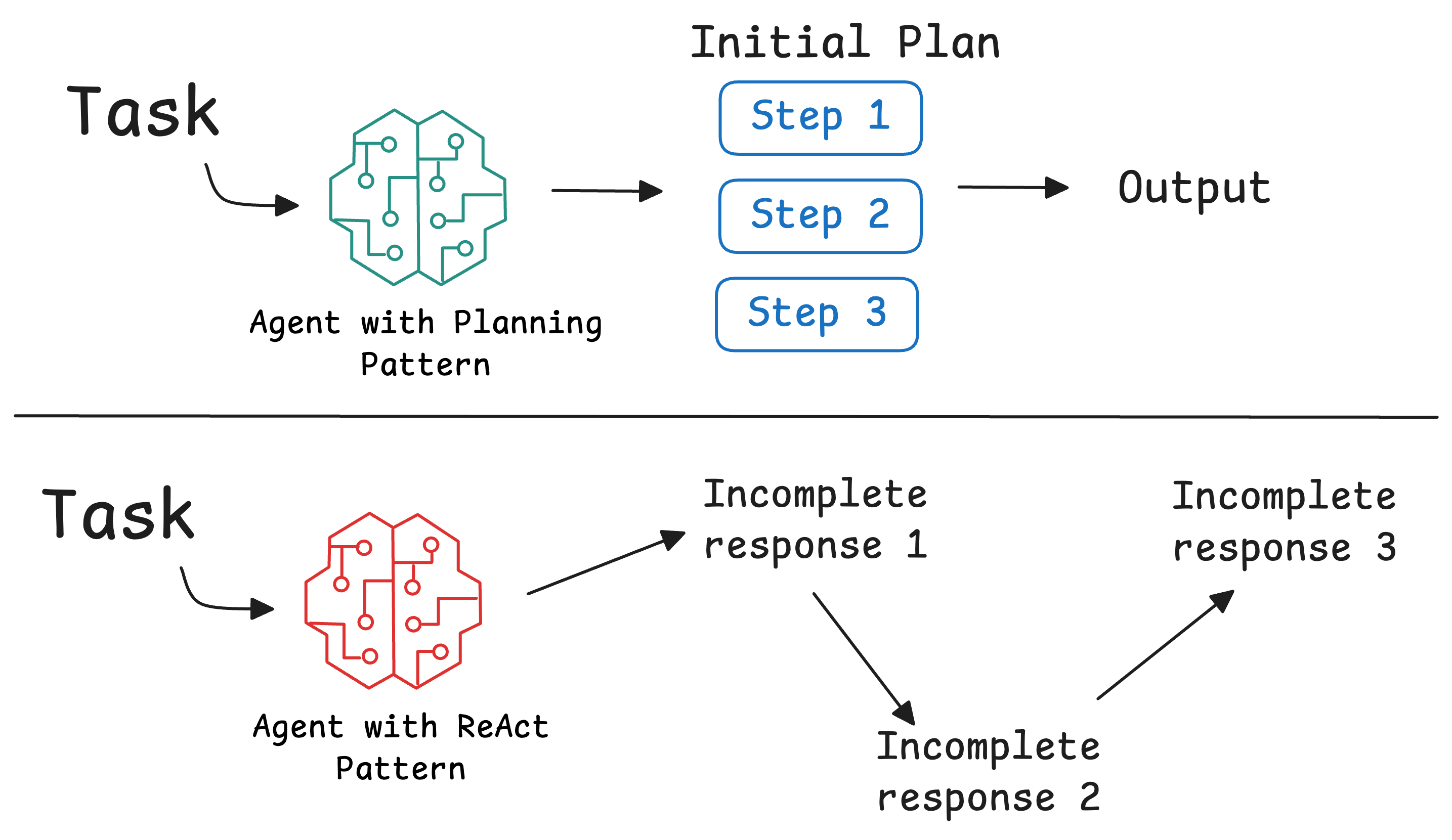
A Planning agent, on the other hand, will attempt to identify all the necessary steps up front and is less likely to get sidetracked.
Let’s compare the two patterns in a nutshell, then dive into the literature (research) review about Planning pattern and finally the implementation.
Read the full article
Sign up now to read the full article and get access to all articles for paying subscribers only.
Join today!