Do you remember the time you first learned about logistic regression?
In most cases, its loss function — log-loss, is introduced out of nowhere, without proper intuition, understanding, and most importantly, without answering the question “Why log-loss”?
$$ \text{log-loss} = - \sum_{i=1}^{N} y_{i} \cdot log(\hat y_{i}) + (1-y_{i}) \cdot log(1 - \hat y_{i}) $$
In fact, while drafting this blog, I did a quick Google search, and disappointingly, NONE of the top-ranked results discussed this.
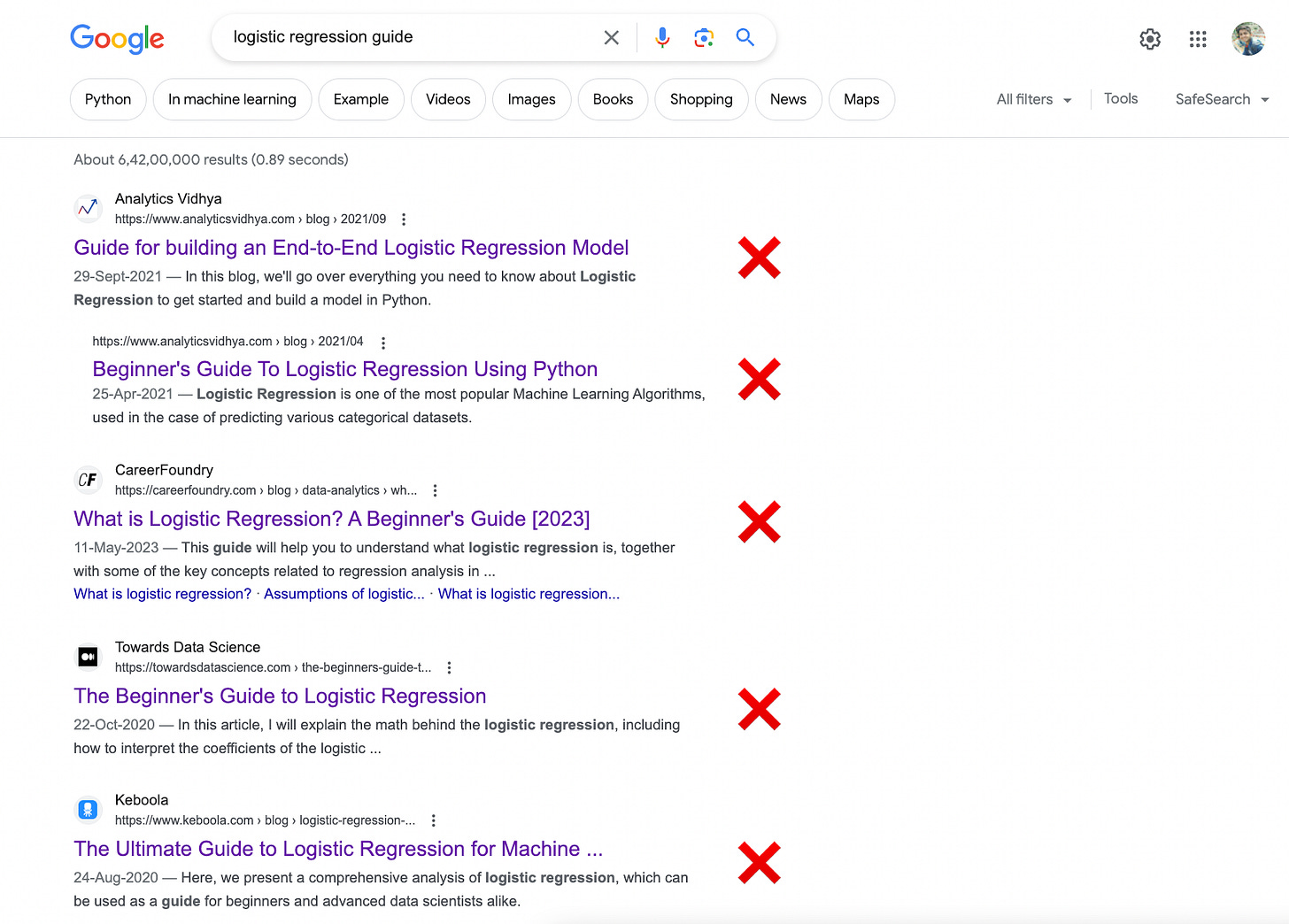
The question is: Why do we specifically minimize the log-loss to train logistic regression? What is so special about it? Where does it come from?
$$ \text{log-loss} = - \sum_{i=1}^{N} y_{i} \cdot log(\hat y_{i}) + (1-y_{i}) \cdot log(1 - \hat y_{i}) $$
Let’s dive in!
Background
Before understanding the origin and utility of this loss function in logistic regression, it is immensely crucial to know how we model data while using logistic regression.
In other words, let’s understand how we frame its modeling mathematically.